当前位置:网站首页>CANN算子:利用迭代器高效实现Tensor数据切割分块处理
CANN算子:利用迭代器高效实现Tensor数据切割分块处理
2022-07-04 18:34:00 【InfoQ】
任务场景及目标
常规方案:
准备知识及分析
1.步长
2.迭代器
template <typename T>
class PositionIterator {
public:
PositionIterator(){};
~PositionIterator(){};
PositionIterator(std::vector<T> stt, std::vector<T> sh) {
if (stt.size() != sh.size()) {
PositionIterator();
} else {
for (unsigned int i = 0; i < sh.size(); i++) {
if (stt[i] >= sh[i]) {
PositionIterator();
}
}
pos_ = stt;
shape_ = sh;
}
}
PositionIterator operator++() {
pos_[shape_.size() - 1] += 1;
for (unsigned int i = shape_.size() - 1; i > 0; i--) {
if (pos_[i] / shape_[i] != 0) {
pos_[i - 1] += pos_[i] / shape_[i];
pos_[i] = pos_[i] % shape_[i];
}
}
return *this;
}
bool End() {
if (pos_[0] != shape_[0]) {
return false;
}
return true;
}
std::vector<T> GetPos() { return pos_; }
std::vector<T> GetShape() { return shape_; }
private:
std::vector<T> pos_;
std::vector<T> shape_;
};
Diagonal算子的实现
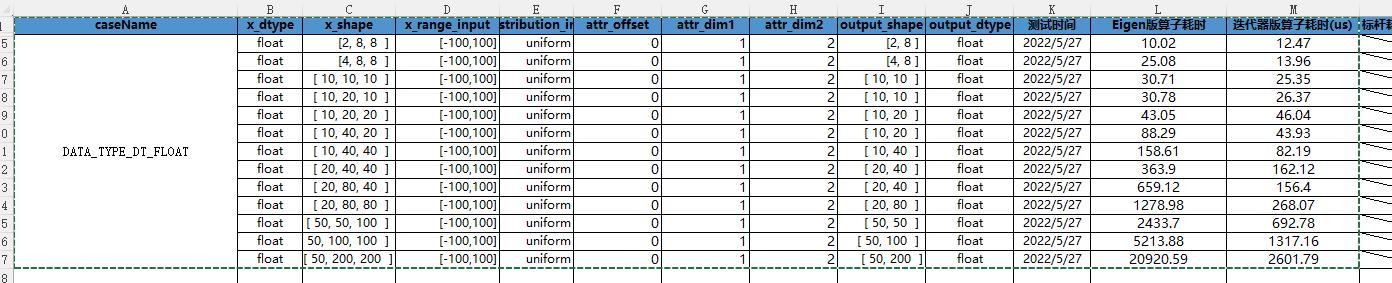
template <typename T>
uint32_t DiagonalCpuKernel::DoComputeType(CpuKernelContext &ctx,
const int64_t &offset,
const int64_t &dim1,
const int64_t &dim2) {
// Get the inuput and output
Tensor *input_x = ctx.Input(0);
Tensor *y = ctx.Output(0);
// Get some information of input
auto x_shape = input_x->GetTensorShape();
std::vector<int64_t> x_shape_ = x_shape->GetDimSizes();
const int64_t x_dim = x_shape->GetDims();
auto dataptr = reinterpret_cast<T *>(ctx.Input(0)->GetData());
auto y_dataptr = reinterpret_cast<T *>(y->GetData());
// Compute
// 首先计算出对角线元素个数
int64_t dsize = OffsetSize(offset, dim1, dim2, x_shape_);
// 生成输入Tensor的步长向量x_stride
std::vector<int64_t> x_stride = ConstructStride<int64_t>(x_shape_);
// 分情况讨论,2维和大于2维的情况
if (x_dim != N2) {
//set the vx_shape and vx_stride
// 生成x_shape和x_stride中除去dim1和dim2对应值的vx_shape与vx_stride
std::vector<int64_t> vx_shape, vx_stride;
for (unsigned int tmp_dim = 0; tmp_dim < x_shape_.size(); tmp_dim++) {
if (tmp_dim != dim1 && tmp_dim != dim2) {
vx_shape.push_back(x_shape_[tmp_dim]);
vx_stride.push_back(x_stride[tmp_dim]);
}
}
// set the y_shape, y_stride, vy_stride
// 生成输出Tensor的形状及步长向量:y_shape和y_stride
std::vector<int64_t> y_shape = vx_shape;
y_shape.push_back(dsize);
std::vector<int64_t> y_stride =
ConstructStride<int64_t>(y_shape);
// 生成输出Tensor的出去最后一维的步长向量:vy_stride
std::vector<int64_t> vy_stride = y_stride;
vy_stride.pop_back();
// 读取对角数据
std::vector<int64_t> v_start(vx_shape.size(), 0);
for (PositionIterator<int64_t> myiter(v_start, vx_shape); !myiter.End();
++myiter) {
// 利用迭代器确定除dim1和dim2维度的位置坐标
auto p = myiter.GetPos();
// 通过步长向量和位置坐标计算出输入和输出的基础位置值base_pos1和outbase_pos
int64_t base_pos1 = MulSum<int64_t>(p, vx_stride);
int64_t outbase_pos = MulSum<int64_t>(p, vy_stride);
for (int i = 0; i < dsize; i++) {
// 结合前面计算出的基础位置值,对dim1和dim2对应维度确定对角元素位置,并赋值给输出数据地址(get_data涉及对上对角还是下对角取元素,不影响对迭代器作用的理解)
int64_t base_pos2 = i * (x_stride[dim1] + x_stride[dim2]);
int64_t arr[N2] = {x_stride[dim1], x_stride[dim2]};
y_dataptr[outbase_pos + i] =
get_data(base_pos1 + base_pos2, offset, arr, dataptr);
}
}
} else {
for (int i = 0; i < dsize; i++) {
int64_t base_pos = i * (x_stride[dim1] + x_stride[dim2]);
int64_t arr[N2] = {x_stride[dim1], x_stride[dim2]};
y_dataptr[i] = get_data(base_pos, offset, arr, dataptr);
}
}
return KERNEL_STATUS_OK;
}
迭代器的其他用法
for (position_iterator<int64_t> mit(v_start, v_shape); !mit.end(); ++mit) {
auto p = mit.get_pos();
int axis_len = input_shape_[tmp_axis];
std::vector<ValueIndex<T>> data_(axis_len);
int base_pos = mul_sum<int64_t>(p, v_stride);
for (int32_t i = 0; i < axis_len; i++) {
data_[i].value = x_dataptr[base_pos + i * input_stride[tmp_axis]];
data_[i].index = i;
}
std::vector<std::vector<T1>> data_;
for (int64_t i = 0; i < dim0; i++) {
std::vector<T1> tmp_v1;
for (PositionIterator<int64_t> mit(v_start, v_shape); !mit.End(); ++mit) {
auto pos = mit.GetPos();
tmp_v1.push_back(
x_dataptr[MulSum<int64_t>(pos, v_stride) + i * input_stride[axis]]);
}
data_.push_back(tmp_v1);
}
边栏推荐
- kotlin 条件控制
- 如何使用Async-Awati异步任务处理代替BackgroundWorker?
- Crawler (6) - Web page data parsing (2) | the use of beautifulsoup4 in Crawlers
- 勾股数规律(任意三个数能够满足勾股定理需要满足的条件)
- Cbcgpprogressdlgctrl progress bar used by BCG
- 黑马程序员-软件测试--07阶段2-linux和数据库-09-24-linux命令学习步骤,通配符,绝对路径,相对路径,文件和目录常用命令,文件内容相关操作,查看日志文件,ping命令使用,
- Master the use of auto analyze in data warehouse
- Jetpack Compose 教程
- Technologie de base de la programmation Shell IV
- Socket programming demo II
猜你喜欢
线上数据库迁移的几种方法
c# .net mvc 使用百度Ueditor富文本框上传文件(图片,视频等)
Stream流
在线SQL转Excel(xls/xlsx)工具
The explain statement in MySQL queries whether SQL is indexed, and several types in extra collate and summarize
Chrome开发工具:VMxxx文件是什么鬼
BCG 使用之新建向导效果
Hough Transform 霍夫变换原理
如何使用Async-Awati异步任務處理代替BackgroundWorker?
Several methods of online database migration
随机推荐
BCG 使用之CBCGPProgressDlgCtrl進度條使用
欧拉函数
1009 product of polynomials (25 points) (PAT class a)
Chrome开发工具:VMxxx文件是什么鬼
kotlin 基本使用
SSRS筛选器的IN运算(即包含于)用法
. Net ORM framework hisql practice - Chapter 2 - using hisql to realize menu management (add, delete, modify and check)
Cbcgpprogressdlgctrl progress bar used by BCG
Allure of pytest visual test report
Crawler (6) - Web page data parsing (2) | the use of beautifulsoup4 in Crawlers
Shell 编程核心技术《三》
HDU 6440 2018中国大学生程序设计网络选拔赛
FTP, SFTP file transfer
Hough Transform 霍夫变换原理
实战模拟│JWT 登录认证
添加命名空间声明
线上数据库迁移的几种方法
Niuke Xiaobai month race 7 who is the divine Archer
TCP两次挥手,你见过吗?那四次握手呢?
Shell 编程核心技术《四》