当前位置:网站首页>你心目中的数据分析 Top 1 选 Pandas 还是选 SQL?
你心目中的数据分析 Top 1 选 Pandas 还是选 SQL?
2022-07-06 21:04:00 【游戏编程】

作者 | 俊欣
来源 | 关于数据分析与可视化
今天小编打算来讲一下 Pandas
和 SQL
之间语法的差异,相信对于不少数据分析师而言,无论是 Pandas
模块还是 SQL
,都是日常学习工作当中用的非常多的工具,当然我们也可以在 Pandas
模块当中来调用 SQL
语句,通过调用 read_sql()
方法。
建立数据库
首先我们通过 SQL
语句在新建一个数据库,基本的语法相信大家肯定都清楚,
CREATE TABLE 表名 ( 字段名称 数据类型 ...)
那么我们来看一下具体的代码
import pandas as pdimport sqlite3connector = sqlite3.connect('public.db')my_cursor = connector.cursor()my_cursor.executescript("""CREATE TABLE sweets_types( id integer NOT NULL, name character varying NOT NULL, PRIMARY KEY (id));...篇幅有限,详细参考源码...""")
同时我们也往这些新建的表格当中插入数据,代码如下
my_cursor.executescript("""INSERT INTO sweets_types(name) VALUES ('waffles'), ('candy'), ('marmalade'), ('cookies'), ('chocolate');...篇幅有限,详细参考源码...""")
我们可以通过下面的代码来查看新建的表格,并且转换成 DataFrame
格式的数据集,代码如下
df_sweets = pd.read_sql("SELECT * FROM sweets;", connector)
output
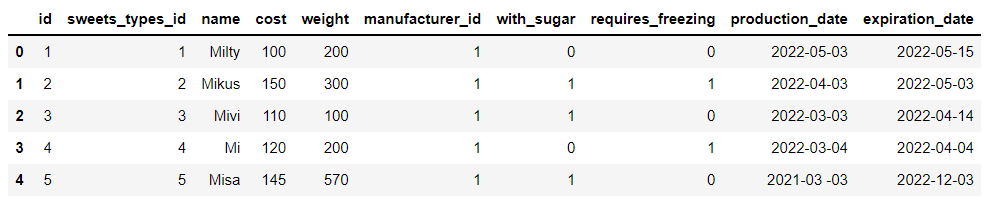
我们总共新建了5个数据集,主要是涉及到了甜品、甜品的种类以及加工和仓储的数据,而例如甜品的数据集当中主要包括的有甜品的重量、糖分的含量、生产的日期和过期的时间、成本等数据,以及:
df_manufacturers = pd.read_sql("SELECT * FROM manufacturers", connector)
output
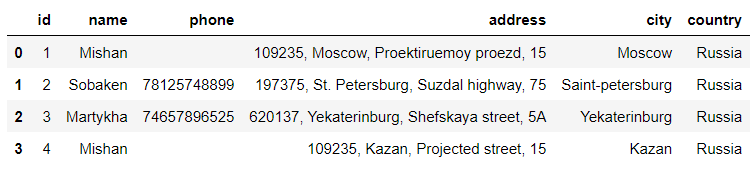
加工的数据集当中则涉及到了工厂的主要负责人和联系方式,而仓储的数据集当中则涉及到了仓储的详细地址、城市所在地等等。
df_storehouses = pd.read_sql("SELECT * FROM storehouses", connector)
output

还有甜品的种类数据集,
df_sweets_types = pd.read_sql("SELECT * FROM sweets_types;", connector)
output

数据筛查
简单条件的筛选
接下来我们来做一些数据筛查,例如筛选出甜品当中重量等于300的甜品名称,在 Pandas
模块中的代码是这个样子的
# 转换数据类型df_sweets['weight'] = pd.to_numeric(df_sweets['weight'])# 输出结果df_sweets[df_sweets.weight == 300].name
output
1 Mikus6 Soucus11 MacusName: name, dtype: object
当然我们还可以通过 pandas
当中的 read_sql()
方法来调用 SQL
语句
pd.read_sql("SELECT name FROM sweets WHERE weight = '300'", connector)
output

我们再来看一个相类似的案例,筛选出成本等于100的甜品名称,代码如下
# Pandasdf_sweets['cost'] = pd.to_numeric(df_sweets['cost'])df_sweets[df_sweets.cost == 100].name# SQLpd.read_sql("SELECT name FROM sweets WHERE cost = '100'", connector)
output
Milty
针对文本型的数据,我们也可以进一步来筛选出我们想要的数据,代码如下
# Pandasdf_sweets[df_sweets.name.str.startswith('M')].name# SQLpd.read_sql("SELECT name FROM sweets WHERE name LIKE 'M%'", connector)
output
MiltyMikusMiviMiMisaMaltikMacus
当然在 SQL
语句当中的通配符, %
表示匹配任意数量的字母,而 _
表示匹配任意一个字母,具体的区别如下
# SQLpd.read_sql("SELECT name FROM sweets WHERE name LIKE 'M%'", connector)
output
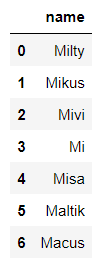
pd.read_sql("SELECT name FROM sweets WHERE name LIKE 'M_'", connector)
output

复杂条件的筛选
下面我们来看一下多个条件的数据筛选,例如我们想要重量等于300并且成本价控制在150的甜品名称,代码如下
# Pandasdf_sweets[(df_sweets.cost == 150) & (df_sweets.weight == 300)].name# SQLpd.read_sql("SELECT name FROM sweets WHERE cost = '150' AND weight = '300'", connector)
output
Mikus
或者是筛选出成本价控制在200-300之间的甜品名称,代码如下
# Pandasdf_sweets[df_sweets['cost'].between(200, 300)].name# SQLpd.read_sql("SELECT name FROM sweets WHERE cost BETWEEN '200' AND '300'", connector)
output

要是涉及到排序的问题,在 SQL
当中使用的是 ORDER BY
语句,代码如下
# SQLpd.read_sql("SELECT name FROM sweets ORDER BY id DESC", connector)
output
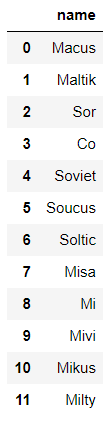
而在 Pandas
模块当中调用的则是 sort_values()
方法,代码如下
# Pandasdf_sweets.sort_values(by='id', ascending=False).name
output
11 Macus10 Maltik9 Sor8 Co7 Soviet6 Soucus5 Soltic4 Misa3 Mi2 Mivi1 Mikus0 MiltyName: name, dtype: object
筛选出成本价最高的甜品名称,在 Pandas
模块当中的代码是这个样子的
df_sweets[df_sweets.cost == df_sweets.cost.max()].name
output
11 MacusName: name, dtype: object
而在SQL语句当中的代码,我们需要首先筛选出成本最高的是哪个甜品,然后再进行进一步的处理,代码如下
pd.read_sql("SELECT name FROM sweets WHERE cost = (SELECT MAX(cost) FROM sweets)", connector)
我们想要看一下是仓储的城市具体是有哪几个,在 Pandas
模块当中的代码是这个样子的,通过调用 unique()
方法
df_storehouses['city'].unique()
output
array(['Moscow', 'Saint-petersburg', 'Yekaterinburg'], dtype=object)
而在 SQL
语句当中则对应的是 DISTINCT
关键字
pd.read_sql("SELECT DISTINCT city FROM storehouses", connector)
数据分组统计
在 Pandas
模块当中分组统计一般调用的都是 groupby()
方法,然后后面再添加一个统计函数,例如是求分均值的 mean()
方法,或者是求和的 sum()
方法等等,例如我们想要查找出在不止一个城市生产加工甜品的名称,代码如下
df_manufacturers.groupby('name').name.count()[df_manufacturers.groupby('name').name.count() > 1]
output
nameMishan 2Name: name, dtype: int64
而在 SQL
语句当中的分组也是 GROUP BY
,后面要是还有其他条件的话,用的是 HAVING
关键字,代码如下
pd.read_sql("""SELECT name, COUNT(name) as 'name_count' FROM manufacturersGROUP BY name HAVING COUNT(name) > 1""", connector)
数据合并
当两个数据集或者是多个数据集需要进行合并的时候,在 Pandas
模块当中,我们可以调用 merge()
方法,例如我们将 df_sweets
数据集和 df_sweets_types
两数据集进行合并,其中 df_sweets
当中的 sweets_types_id
是该表的外键
df_sweets.head()
output
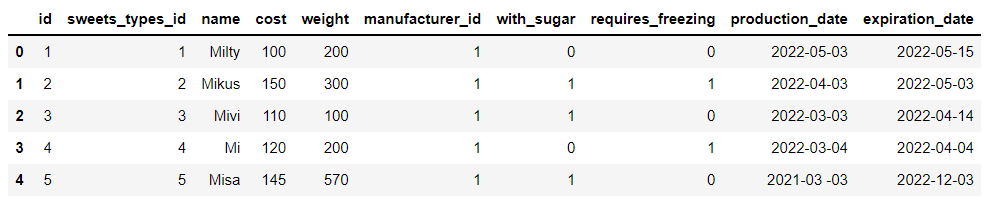
df_sweets_types.head()
output

具体数据合并的代码如下所示
df_sweets_1 = df_sweets.merge(df_sweets_types, left_on='sweets_types_id', right_on='id')
output
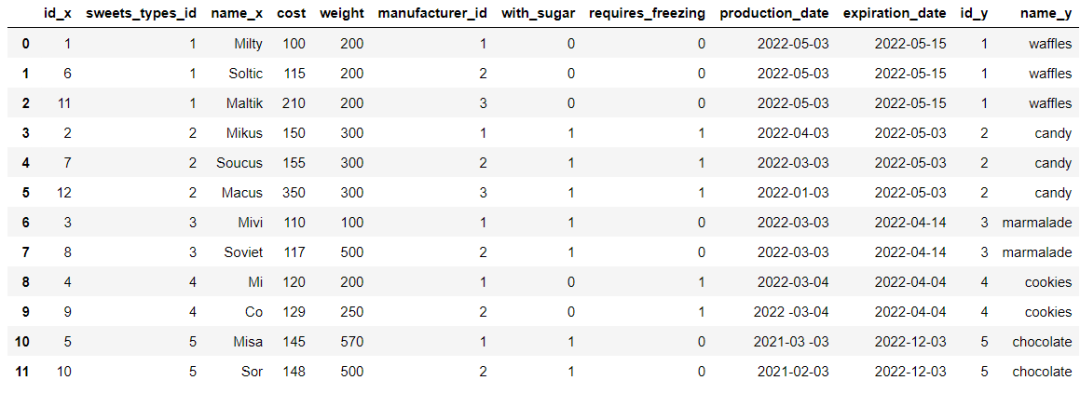
我们再进一步的筛选出巧克力口味的甜品,代码如下
df_sweets_1.query('name_y == "chocolate"').name_x
output
10 Misa11 SorName: name_x, dtype: object
而 SQL
语句则显得比较简单了,代码如下
# SQLpd.read_sql("""SELECT sweets.name FROM sweetsJOIN sweets_types ON sweets.sweets_types_id = sweets_types.idWHERE sweets_types.name = 'chocolate';""", connector)
output

数据集的结构
我们来查看一下数据集的结构,在 Pandas
模块当中直接查看 shape
属性即可,代码如下
df_sweets.shape
output
(12, 10)
而在 SQL
语句当中,则是
pd.read_sql("SELECT count(*) FROM sweets;", connector)
output


往期回顾
分享点收藏点点赞点在看
作者:AI科技大本营
游戏编程,一个游戏开发收藏夹~
如果图片长时间未显示,请使用Chrome内核浏览器。
边栏推荐
- First understand the principle of network
- VHDL实现任意大小矩阵乘法运算
- 21.(arcgis api for js篇)arcgis api for js矩形采集(SketchViewModel)
- CVPR 2022 best paper candidate | pip: six inertial sensors realize whole body dynamic capture and force estimation
- Tencent cloud native database tdsql-c was selected into the cloud native product catalog of the Academy of communications and communications
- [MySQL] row sorting in MySQL
- What about SSL certificate errors? Solutions to common SSL certificate errors in browsers
- SSL certificate deployment
- [development software] tilipa Developer Software
- When QT uses qtooltip mouse to display text, the picture of the button will also be displayed and the prompt text style will be modified
猜你喜欢
Force buckle ----- path sum III
Restcloud ETL Community Edition June featured Q & A
U.S. Air Force Research Laboratory, "exploring the vulnerability and robustness of deep learning systems", the latest 85 page technical report in 2022
1200.Minimum Absolute Difference
Flutter3.0, the applet is not only run across mobile applications
预处理——插值
Adaptive non European advertising retrieval system amcad
Ubuntu20 installation redisjson record
It's too convenient. You can complete the code release and approval by nailing it!
链表面试常见题
随机推荐
Native MySQL
2022.6.28
About Estimation Statistics
概率论公式
Docker部署Mysql8的实现步骤
Ubuntu20 installation redisjson record
QT 打开文件 使用 QFileDialog 获取文件名称、内容等
ggplot 分面的细节调整汇总
维护万星开源向量数据库是什么体验
24.(arcgis api for js篇)arcgis api for js点修改点编辑(SketchViewModel)
Sorting operation partition, argpartition, sort, argsort in numpy
10 ways of interface data security assurance
RestClould ETL 社区版六月精选问答
Class常量池与运行时常量池
Optimization cases of complex factor calculation: deep imbalance, buying and selling pressure index, volatility calculation
ERROR: Could not build wheels for pycocotools which use PEP 517 and cannot be installed directly
Introduction to opensea platform developed by NFT trading platform (I)
Clock in during winter vacation
Confirm the future development route! Digital economy, digital transformation, data This meeting is very important
Gpt-3 is a peer review online when it has been submitted for its own research