当前位置:网站首页>I wrote a learning and practice tutorial for beginners!
I wrote a learning and practice tutorial for beginners!
2022-07-04 18:05:00 【Datawhale】
Datawhale dried food
author : Herding bear ,Datawhale member
In the last week Datawhale Shared an article about the data mining competition baseline programme , Some teachers use it as learning materials for students to practice and study , Many students reported that they still had difficulties in learning and practice :
classmate A:baseline Some codes are not understood
classmate B: Just started learning , Want to get started , I don't know where to start , Then the theory is not very solid , I don't know whether to learn theory first or start first ; See the plan , I don't know what knowledge to use , Still quite confused ;
classmate C: For beginners, you need to be careful and comprehensive , For example, including file reading 、 Feature handling 、 Definition of input / output data structure 、 Preliminary results 、 Is there anything worth optimizing …… wait
So I wrote a new article , For beginners in competition practice : It provides a complete process of competition practice 、 Complete code comments 、 And reference learning materials .
Introduction to the contest question
Hkust xunfei : Diabetes genetic risk testing challenge . By 2022 year , Diabetes mellitus in China 1.3 Billion . The causes of diabetes in China are influenced by lifestyle 、 Aging 、 Urbanization 、 Family heredity and other factors affect . meanwhile , People with diabetes tend to be younger .
Diabetes can lead to cardiovascular disease 、 Kidneys 、 Occurrence of cerebrovascular complications . therefore , Accurate diagnosis of individuals with diabetes has very important clinical significance . Early genetic risk prediction of diabetes will help to prevent the occurrence of diabetes .
Event practice address :
https://challenge.xfyun.cn/topic/info?type=diabetes&ch=ds22-dw-gzh02
Match task
In this competition , You need to go through Training data set structure Genetic risk prediction model of diabetes , And then predict Test data set Whether the middle-aged individual has diabetes , Join us to help diabetes patients solve this problem “ Sweet troubles ”.
Question data
The competition data consists of training set and test set , The details are as follows :
Training set : share 5070 Data , Used to build your forecasting model
Test set : share 1000 Data , Used to verify the performance of the prediction model .
The training set data package contains 9 A field : Gender 、 Year of birth 、 Body mass index 、 Family history of diabetes 、 diastolic pressure 、 Oral glucose tolerance test 、 Insulin release test 、 Triceps brachii skinfold thickness 、 Signs of diabetes ( Data labels ).
Standard for evaluation
Use... In the two category task F1-score Indicators for evaluation ,F1-score The larger the size, the better the performance of the prediction model ,F1-score Is defined as follows :
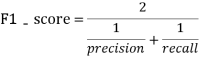
among :


Tips: According to the meaning , Diabetes genetic risk testing challenge will provide 2 Data sets , They are training data set and test data set , The training data set includes characteristic data and data labels ( Whether the patient has diabetes ), The test data set has only characteristic data , We need to Genetic risk prediction model of diabetes , The competitor evaluates the prediction accuracy of the model by testing the data set , The higher the accuracy of the model prediction, the better .
Ref:
You know : II. Common evaluation indicators for classification problems
https://zhuanlan.zhihu.com/p/55324860
Competition questions Baseline
Tips: In this competition , We will provide python The code is used for the analysis of competition data and model construction , If you are not familiar with the relevant codes and principles in the competition , You can refer to relevant learning materials or in Datawhale Communicate in groups to solve the problems you encounter .
step 1: Sign up for the competition :
https://challenge.xfyun.cn/topic/info?type=diabetes&ch=ds22-dw-gzh02
step 2: Download game data ( Click the question data on the competition page )
step 3: Decompress the game data , And use pandas To read
Import third-party library
Tips: In Ben baseline in , We go through pandas Process the data , adopt lightgbm Algorithm to build Genetic risk prediction model of diabetes
Ref:
pandas usage - The most detailed course of the whole network
https://blog.csdn.net/yiyele/article/details/80605909
import pandas as pd
import lightgbm
Data preprocessing
Tips: In this session , We usually need to check the quality of data , Including duplicate values 、 outliers 、 Missing value 、 Data distribution and data characteristics, etc , Through the preprocessing of training data , We can get higher quality training data , This helps to build a more accurate prediction model .
In Ben baseline in , We found that
diastolic pressure
There is a missing value in the feature , We use the method of filling in missing values , Of course, there are other ways to deal with it , If you are interested, you can try .Ref:
machine learning ( 3、 ... and ): Data preprocessing -- The basic method of data preprocessing
https://zhuanlan.zhihu.com/p/100442371
data1=pd.read_csv(' Game training set .csv',encoding='gbk')
data2=pd.read_csv(' Competition test set .csv',encoding='gbk')
#label Marked as -1
data2[' Signs of diabetes ']=-1
# The training set and the testing machine are merged
data=pd.concat([data1,data2],axis=0,ignore_index=True)
# Fill the missing values in the diastolic blood pressure characteristics with -1
data[' diastolic pressure ']=data[' diastolic pressure '].fillna(-1)
Feature Engineering
Tips: In this session , We need to construct features of data , The aim is to extract features from the original data to the maximum extent for use by algorithms and models , This helps to build a more accurate prediction model .
Ref:
# Feature Engineering
"""
Convert the year of birth into age
"""
data[' Year of birth ']=2022-data[' Year of birth '] # Change to age
"""
The normal value of the body mass index for adults is 18.5-24 Between
lower than 18.5 It's a low BMI
stay 24-27 Between them is overweight
27 The above consideration is obesity
higher than 32 You are very fat .
"""
def BMI(a):
if a<18.5:
return 0
elif 18.5<=a<=24:
return 1
elif 24<a<=27:
return 2
elif 27<a<=32:
return 3
else:
return 4
data['BMI']=data[' Body mass index '].apply(BMI)
# Family history of diabetes
"""
No record
One uncle or aunt has diabetes / One uncle or aunt has diabetes
One parent has diabetes
"""
def FHOD(a):
if a==' No record ':
return 0
elif a==' One uncle or aunt has diabetes ' or a==' One uncle or aunt has diabetes ':
return 1
else:
return 2
data[' Family history of diabetes ']=data[' Family history of diabetes '].apply(FHOD)
"""
The diastolic pressure range is 60-90
"""
def DBP(a):
if a<60:
return 0
elif 60<=a<=90:
return 1
elif a>90:
return 2
else:
return a
data['DBP']=data[' diastolic pressure '].apply(DBP)
#------------------------------------
# The processed feature engineering is divided into training set and test set , The training set is used to train the model , The test set is used to evaluate the accuracy of the model
# There is no relationship between the number and whether the patient has diabetes , Irrelevant features shall be deleted
train=data[data[' Signs of diabetes '] !=-1]
test=data[data[' Signs of diabetes '] ==-1]
train_label=train[' Signs of diabetes ']
train=train.drop([' Number ',' Signs of diabetes '],axis=1)
test=test.drop([' Number ',' Signs of diabetes '],axis=1)
Build the model
Tips: In this session , We need to train the training set to build the corresponding model , In Ben baseline And we used that Lightgbm Algorithm for data training , Of course, you can also use other machine learning algorithms / Deep learning algorithm , You can even synthesize the results predicted by different algorithms , Anyway, the final goal is to obtain higher prediction accuracy , Towards this goal ~
In this section , We will use training data 5 Fold cross validation training method for training , This is a good way to improve the accuracy of model prediction
Ref:
# Use Lightgbm Methods training data set , Use 5 Fold cross validation method to obtain 5 Test set prediction results
def select_by_lgb(train_data,train_label,test_data,random_state=2022,n_splits=5,metric='auc',num_round=10000,early_stopping_rounds=100):
kfold = KFold(n_splits=n_splits, shuffle=True, random_state=random_state)
fold=0
result=[]
for train_idx, val_idx in kfold.split(train_data):
random_state+=1
train_x = train_data.loc[train_idx]
train_y = train_label.loc[train_idx]
test_x = train_data.loc[val_idx]
test_y = train_label.loc[val_idx]
clf=lightgbm
train_matrix=clf.Dataset(train_x,label=train_y)
test_matrix=clf.Dataset(test_x,label=test_y)
params={
'boosting_type': 'gbdt',
'objective': 'binary',
'learning_rate': 0.1,
'metric': metric,
'seed': 2020,
'nthread':-1 }
model=clf.train(params,train_matrix,num_round,valid_sets=test_matrix,early_stopping_rounds=early_stopping_rounds)
pre_y=model.predict(test_data)
result.append(pre_y)
fold+=1
return result
test_data=select_by_lgb(train,train_label,test)
#test_data Namely 5 In cross validation 5 The result of this prediction
pre_y=pd.DataFrame(test_data).T
# take 5 Calculate the average value of the prediction results , Of course, other methods can also be used
pre_y['averge']=pre_y[[i for i in range(5)]].mean(axis=1)
# Because the competition requires you to submit the final prediction and judgment , The prediction result given by the model is probability , So we think that probability >0.5 That is, the patient has diabetes , probability <=0.5 There is no diabetes
pre_y['label']=pre_y['averge'].apply(lambda x:1 if x>0.5 else 0)
pre_y
Results submitted
Tips: In this session , We need to submit the final prediction results to the data competition platform , It should be noted that we should strictly follow the file format submission requirements of the competition platform .
result=pd.read_csv(' Submit sample .csv')
result['label']=pre_y['label']
result.to_csv('result.csv',index=False)
among result.csv The files that need to be submitted to the platform , Enter the data competition platform , Click on Submit results
, choice result.csv The document can complete the result submission
follow-up
Through simple learning , We completed the diabetes genetic risk testing challenge baseline Mission , What should we do next ? It is mainly the following several aspects :
Continue to try different prediction models or feature engineering to improve the accuracy of model prediction
Join in Datawhale Competition exchange group , Get other more effective scoring information
Refer to relevant data on genetic risk prediction of diabetes , Get other model building methods
...
All in all , Is in the baseline On the basis of continuous transformation and try , Improve your data mining ability through continuous practice , As the saying goes 【 It's on paper , We must know that we must do it 】, Maybe you are proficient in machine learning algorithms , Be able to deduce various formulas skillfully , But how to apply the learned methods to practical engineering , We need to constantly try and improve , No model is a one-step result , To the final champion ~
Ref:
Focus on Datawhale official account , reply “ data mining ” or “CV” or “NLP” You can be invited to the exchange group of relevant events , You don't need to add any more if you are already there .
Sorting is not easy to , spot Fabulous Three even ↓
边栏推荐
- Open source PostgreSQL extension age for graph database was announced as the top-level project of Apache Software Foundation
- 90后开始攒钱植发,又一个IPO来了
- Self reflection of a small VC after two years of entrepreneurship
- 【系统盘转回U盘】记录系统盘转回U盘的操作
- 【Proteus仿真】基于VSM 串口printf调试输出示例
- celebrate! Kelan sundb and Zhongchuang software complete the compatibility adaptation of seven products
- 【Hot100】31. 下一个排列
- Recast of recastnavigation
- 项目通用环境使用说明
- 股价大跌、市值缩水,奈雪推出虚拟股票,深陷擦边球争议
猜你喜欢
Oppo Xiaobu launched Obert, a large pre training model, and promoted to the top of kgclue
Electronic pet dog - what is the internal structure?
[HCIA continuous update] WLAN overview and basic concepts
[Huawei HCIA continuous update] SDN and FVC
就在今天丨汇丰4位专家齐聚,共讨银行核心系统改造、迁移、重构难题
Detectron2 installation method
庆贺!科蓝SUNDB与中创软件完成七大产品的兼容性适配
RecastNavigation 之 Recast
【HCIA持续更新】广域网技术
补能的争议路线:快充会走向大一统吗?
随机推荐
解读数据安全治理能力评估框架2.0,第四批DSG评估征集中
ISO27001认证办理流程及2022年补贴政策汇总
雨量预警广播自动化数据平台BWII 型广播预警监测仪
Cann operator: using iterators to efficiently realize tensor data cutting and blocking processing
Make a grenade with 3DMAX
Rainfall warning broadcast automatic data platform bwii broadcast warning monitor
Redis master-slave replication
Implementation of shell script replacement function
估值900亿,超级芯片IPO来了
Stars open stores, return, return, return
你应该懂些CI/CD
Initial experience of domestic database tidb: simple and easy to use, quick to start
智捷云——元宇宙综合解决方案服务商
Summary of subsidy policies across the country for dcmm certification in 2022
R language plot visualization: plot visualization of multiple variable violin plot in R with plot
VSCode修改缩进不成功,一保存就缩进四个空格
[proteus simulation] printf debugging output example based on VSM serial port
【Hot100】31. 下一个排列
Performance test of Gatling
[daily question] 871 Minimum refueling times