当前位置:网站首页>图节点分类与消息传递 - 知乎
图节点分类与消息传递 - 知乎
2020-11-09 07:30:00 【osc_gnljyuoo】
Message passing and node classification
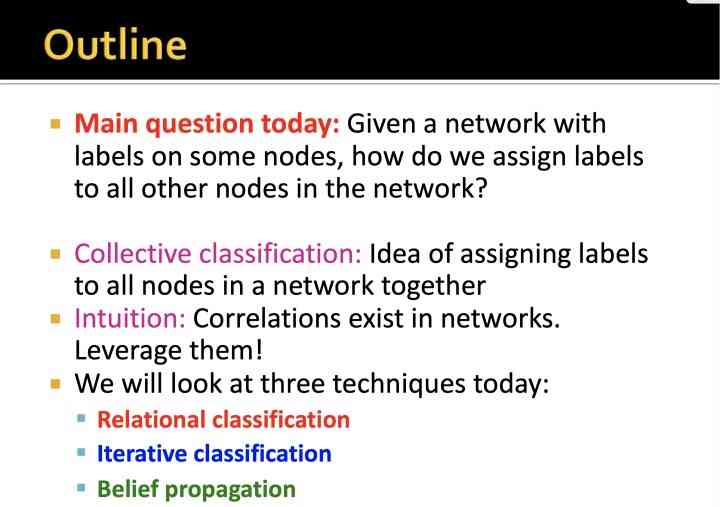
本文主要解决的问题:
给定一个网络, 其中部分节点有label, 如何能将其他的节点分配对应的节点label呢? (在生活中有很多这样的例子, 比如通过交互行为来判断用户是否是薅羊毛党)直觉上来说会考虑网络节点间彼此的连接关系, 本文中会学习的模型是collective classification:
这其中会涉及到三类具体的方法:
- Relational classification;
- Iterative classification;
- Belief propagation;
Relational classification
Correlations Exists In Network
网络中的节点的行为是corrlated, 具体表现为三种:
- Homophily:有相同性质的节点可能有更多的网络联系,如有相同音乐爱好的人更容易在相同的论坛版块;
- Influence:一个节点的特征可能会受其他节点的影响,如假如我向我的朋友推荐我感兴趣的音乐专辑, 朋友中的一些很有可能变成和我一样对这些音乐专辑感兴趣的人;
- Confounding:环境能够对节点的特征和节点间的联系产生影响;
Classification with network data
Motivation
相似的节点通常在网络中彼此相连,或连接比较紧密; 而”Guilt-by-association“:关联推定就是考虑这部分信息来对网络中节点类型进行推断; 所以网络中节点类型的判断主要通过以下三个来源的特征:
- 本身节点的特征;
- 节点相连邻居的label;
- 节点相连邻居的特征;
Guilt-by-association
"Guilt-by-association"的问题比较好定义,如下图,给定Graph以及少量有label的节点,如何将剩余的节点标注为对应label,要求:网络有同质性:
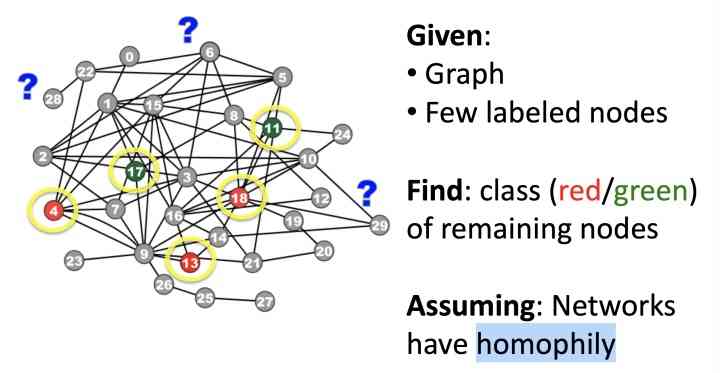
对该问题进行数学建模:给定邻接矩阵W,大小为n*n, 以及Y为节点对应label,1为positive节点,-1位negative节点, 0位unlabeled节点,预测unlabeled的节点中postive的概率。 这里做马尔科夫假设:节点的label类型,仅取决于相连节点的label类型:
Collective classification 通常包括三个步骤:
- local classifier:仅考虑节点特征信息进行分类;
- Relational Classifier:考虑节点邻居的特征和类别信息;
- collective inference:将Relational Classifier应用到每一个节点上,直到整个网络的”inconsistency“最小化;
Probabilistic Relational Classifier
Probabilistic Relational Classifier 基本思路简单,将每个节点类别的概率是其相邻节点的加权平均,具体过程如下:
- 对于有标签的数据,将节点的类表标注为label;
- 对于没有标签的数据,将其类随机初始化;
- 然后按照随机顺序进行邻接节点类别加权,直到整个网络收敛或者达到最大迭代次数:
其中 是指节点i与节点j的连接权重。
这个模型存在两个问题:
- 不能保证模型一定收敛;
- 该模型并没有使用到节点的特征信息;
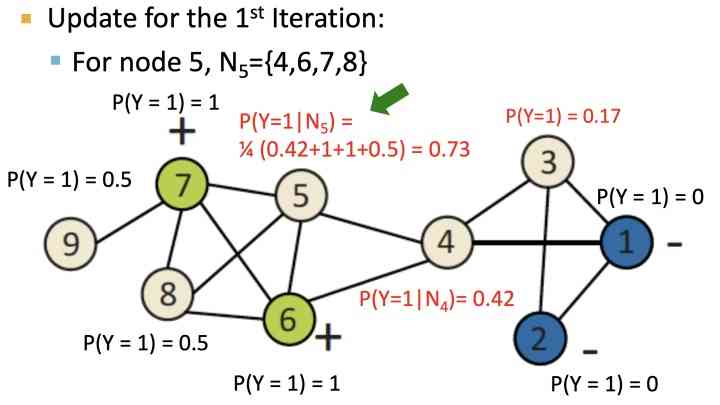
如上图,对初始化后的节点, 按label邻接节点来进行加权计算,得到节点类型概率,直到收敛:经过5轮迭代之后:
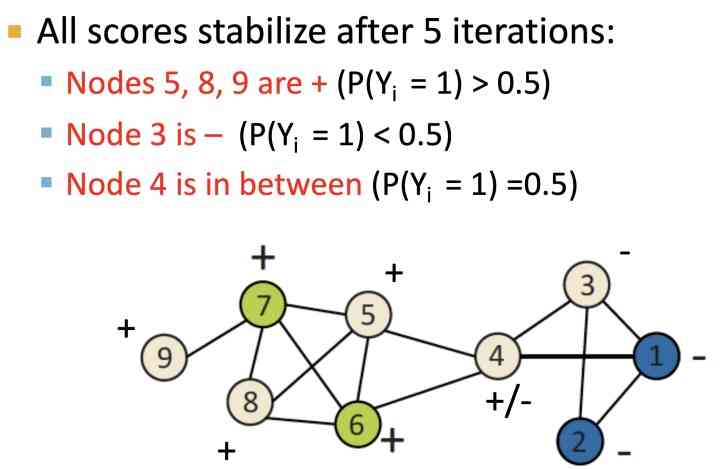
Iterative Classifier
Iterative Classifier即加上节点特征后的迭代过程,主要包括两个过程:
- Bootstrap Phase:将节点i用其特征表示,使用local classifier 去计算每个节点的label概率;
- Iteration Phase:重复针对网络中每个节点,更新节点特征以及按local classifier对该节点进行分类,直到label稳定;
视频教程中,举了一个使用Iterative Classifier通过考虑网络结构信息来做fake review detection的, 建议大家可以详细看看论文: REV2_Fraudulent_User_Prediction_in_Rating_Platforms
Collective Classification
Belief Propagation是一个动态规划过程, 通过节点间passing message的手段, 主要用于解决图当中条件概率的问题。
Message Passing
举个例子来解释message passing的过程:
给定任务:统计图当中所有节点的数量, 其中每个节点只能与它的邻居节点来交互
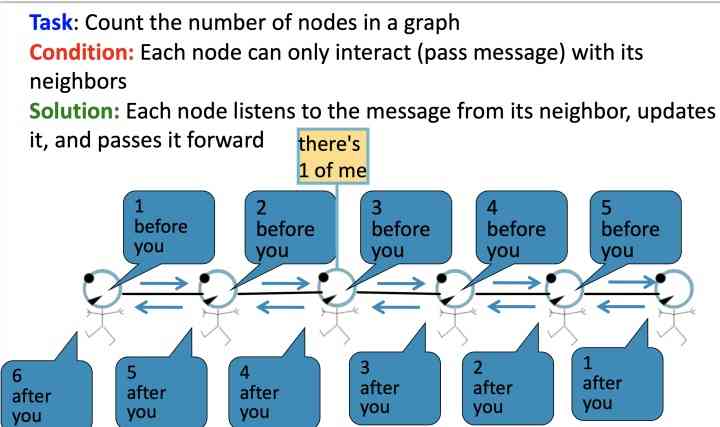
如上图,每一个节点会监听来自于它邻居的信息,然后更新信息,并将其向前传递, 如下图,标红圈的节点仅能接受到incoming messages:
- 2 nodes before you;
- 3 nodes behind you:
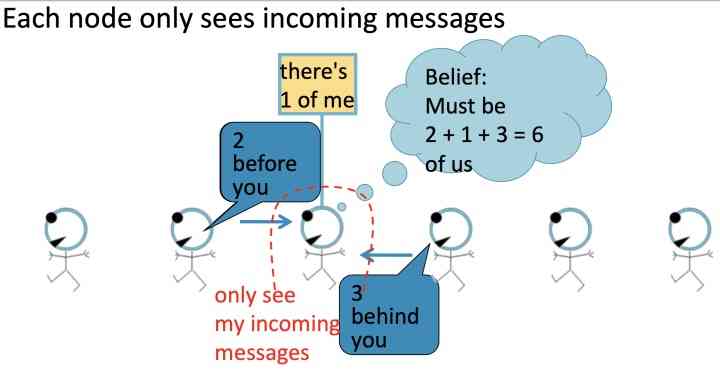
在稍微更复杂的tree型的graph来展示:
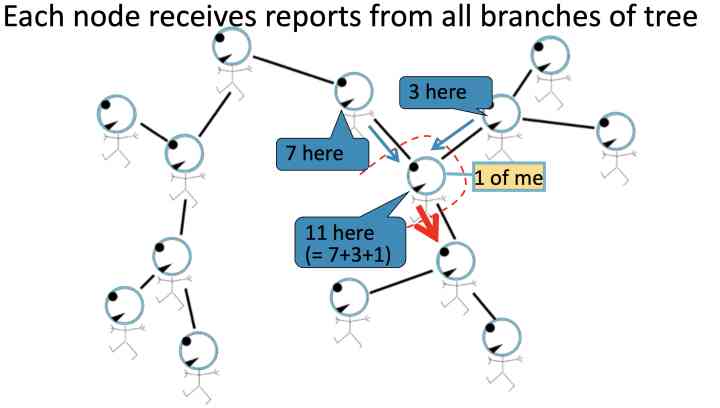
Loopy Belief Propagation
为了讲清楚, 这里做一个基础的定义:
- Label-Label potential matrix
: 表示节点i是类别
的条件下,其邻接节点 j 为类别
的概率;
- prior belief
:表示节点i为类别
的先验概率;
:节点i预测其邻接节点j为状态
:
上面其实很好理解:该公式考虑节点i为 的先验概率ψ,且根据类似于状态转移矩阵来得到节点j为
, 同时考虑所有邻居节点传递的信息
, 随机顺序迭代,直到最终状态稳定, 得到节点i为类别Y_{i}Yi的概率:
考虑到节点,在做message passing时,其实没办法判断是否有cycle, 所以LBP在遇到cycles时会粗在一些问题,如下图,这里稍稍有一些不明白,如果有理解的欢迎评论区讨论:
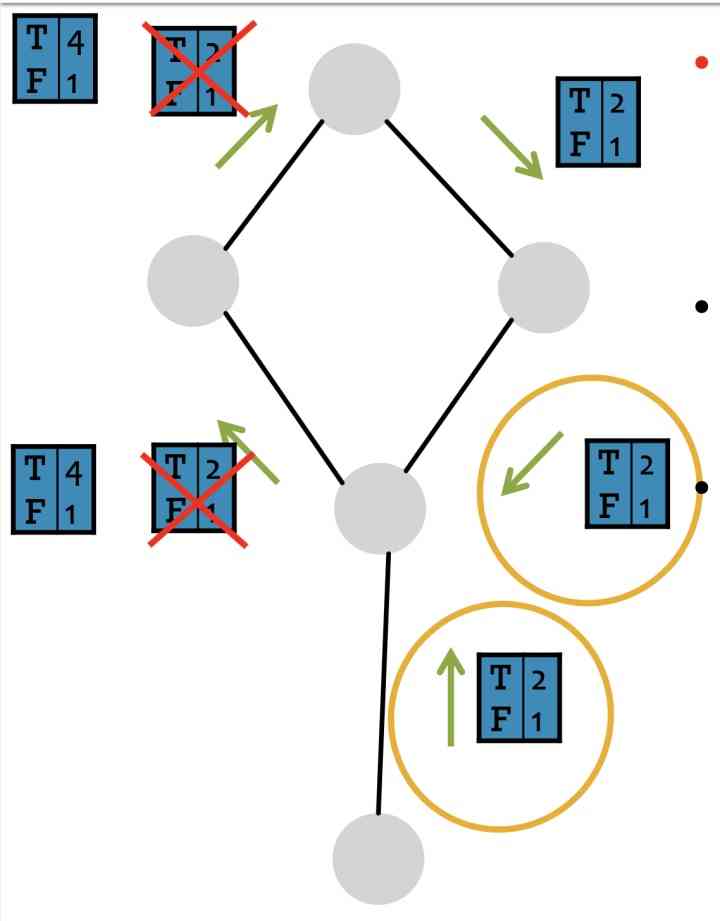
LBP的优缺点如下:
- 优点:
- 实现简单,极易并行化;
- 普适性强,适用所有图模型;
- 挑战:
- 无法保证收敛,尤其是在用很多闭合的连接;
- 势函数:
- 需要魔性训练;
- 训练学习是基于梯度优化的方法,在训练过程中可能存在手链问题;
Summary
本文基于cs224w学习整理而来,在本文中主要介绍了在node classification经典的三类处理方法:Relational classification、Iterative Classifier、Collective Classification, 介绍了经典的解决方案,如Probabilistic Relational Classifier、Message Passing, 文章中有若干案例,因为时间问题未整理,需要详细了解的建议学习本章教程,以及相关paper, node classification是经典的graph的问题,在很多反欺诈案例中有应用。
版权声明
本文为[osc_gnljyuoo]所创,转载请带上原文链接,感谢
https://my.oschina.net/u/4329790/blog/4708500
边栏推荐
- Nodejs中request出现ESOCKETTIMEDOUT解决方案
- How to reduce the resource consumption of istio agent through sidecar custom resource
- When we talk about data quality, what are we talking about?
- salesforce零基础学习(九十八)Salesforce Connect & External Object
- Travel notes of csp-s 2020
- SQL语句的执行
- Chapter 5 programming
- Flink's datasource Trilogy 3: customization
- Linked blocking queue based on linked list
- object
猜你喜欢
Programmers should know the URI, a comprehensive understanding of the article
OpenGL ES 框架详细解析(八) —— OpenGL ES 设计指南
C / C + + Programming Notes: pointer! Understand pointer from memory, let you understand pointer completely
几行代码轻松实现跨系统传递 traceId,再也不用担心对不上日志了!
STC转STM32第一次开发
B. protocal has 7000eth assets in one week!
FC 游戏机的工作原理是怎样的?
14. Introduction to kubenetes
Esockettimeout solution in request in nodejs
Several common playing methods of sub database and sub table and how to solve the problem of cross database query
随机推荐
When iperf is installed under centos7, the solution of make: * no targets specified and no makefile found. Stop
Introduction to nmon
Pipedrive如何在每天部署50+次的情况下支持质量发布?
写时复制集合 —— CopyOnWriteArrayList
Database design: paradigms and anti paradigms
Core knowledge of C + + 11-17 template (2) -- class template
What are the basic requirements for big data posts?
leetcode之反转字符串中的元音字母
First development of STC to stm32
C / C + + Programming Notes: pointer! Understand pointer from memory, let you understand pointer completely
14.Kubenetes简介
Five design patterns frequently used in development
The difference between GDI and OpenGL
作业2020.11.7-8
How to do thread dump analysis in Windows Environment
Esockettimeout solution in request in nodejs
2. Introduction to computer hardware
API部分的知识点复习
《MFC dialog中加入OpenGL窗体》
This program cannot be started because msvcp120.dll is missing from your computer. Try to install the program to fix the problem