当前位置:网站首页>Mysql index details (with pictures and texts)
Mysql index details (with pictures and texts)
2022-08-02 13:20:00 【[email protected]】
This article is relatively long,I won't be able to watch it in a while,It is recommended that you collect it and watch it slowly,The relevant knowledge about the index is basically recorded.,This article is enough to make yourMysql知识更上一层楼!
1. 索引概述
1.1 什么是索引?
索引(index)是帮助MySQL高效获取数据的数据结构(有序)
.在数据之外,数据库系统还维护着满足特定查找算法的数据结构,这些The data structure is referenced in some way(指向)数据, 这样就可以在这些数据结构上实现高级查找算法
,这种数据结构就是索引
.
Want to learn good index,Then you must mastermysql的数据结构
,In fact, when it comes to data structures,For those with a poor foundation,Sometimes very headache,But don't worry about it here,Next, we will focus on the data structure.,Try to describe each data structure in vernacular form!!!
1.2 The difference between using an index and not using an index
Here we mainly demonstrate the difference between not using an index and using an index.
表结构及其数据如下:
假如我们要执行的SQL语句为 : select * from user where age = 45;
(1)无索引情况
在无索引情况下,就需要从第一行开始扫描,一直扫描到最后一行,我们称之为 全表扫描,性能很低.可能有的人该说了,明明在id为7data has been foundage为45的数据,Why is a full table scan?
因为对于mysqlHe did not know whether there is behindage为45的数据,So he will not drop any data!
(2)有索引情况
If we target this table'sage字段建立了索引,假设索引结构就是二叉树,那么也就意味着,会对age这个字段建立一个二叉树的索引结构.And each node in this binary tree stores the location of the real data,We just found out the corresponding of the treeagemeans finding the real data!
如下图:当查找age为45的时候,At this time, it will judge from the root node,根节点为36,比36big so start walking to the right node,This light directly excludes the data on the left side of the tree,Then make a judgment48小,At this time, go to the left node,然后就找到了,只需要扫描三次就可以找到数据了,极大的提高的查询的效率.
Whether it's binary tree orB+树,must be in order,He is adding data,Sorted by the size of the data and then forked.也正因为如此,So the query speed is improved!
备注: 这里我们只是假设索引的结构是二叉树,介绍一下索引的大概原理,只是一个示意图,并不是索引的真实结构,索引的真实结构,后面会详细介绍.
1.3 索引的特点
降低数据库的IO,什么是IO?
IO就是所谓的流,Streams are divided into read and write,When we want to find data from a file, we need to read,When you need to modify the file, you need to write,MysqlThe final stored data is in the disk file,Then we want to find a piece of data,怎么办呢?
First think about how we want to find any data in a file in reality.,直接打开文件,然后全局搜索,If the file is large,Search will also have a point card.mysqlHe's not the same with us,We belong to the familywindowsThe system provides us with this convenience,We can open the file directly,然后进行搜索.
mysqlIf it is a full table scan,First you need to get from the data file Read all the data of this table into memory,Then, determine which data is eligible.Among them, this also tests the ability of our computer to read,Of course, the higher the configuration of the computer read faster.
If the index,We just need to read the index,Because the index points to the address of the data on the file.So just need to find the index of the corresponding data,Then get the location of the data through the index,Then read this data from the data file.,also reducedIO成本.
If the dataset is read into memory,If the computer memory is only16G,And this table has200G,Once full table scan,Did the computer just hang up??
实际上,服务端并不需要保存一个完整的结果集.取数据和发数据的流程是这样的:
- 获取一行,写到 net_buffer 中.这块内存的大小是由参数 net_buffer_length 定义的,默认是 16k.
- 重复获取行,直到 net_buffer 写满,调用网络接口发出去.
- 如果发送成功,就清空 net_buffer,然后继续取下一行,并写入 net_buffer.
- 如果发送函数返回 EAGAIN 或 WSAEWOULDBLOCK,就表示本地网络栈(socket send buffer)写满了,进入等待.直到网络栈重新可写,再继续发送.
So we are using the process,basically impossible becausemysqlThe server memory is full due to query data,mysqlMainly occupy our serverIO.
2. 索引结构
2.1 概述
MySQL的索引是在存储引擎层实现的,不同的存储引擎有不同的索引结构,主要包含以下几种:
上述是MySQL中所支持的所有的索引结构,接下来,我们再来看看不同的存储引擎对于索引结构的支持情况.
注意: It will be mainly used in actual developmentB+Tree,Therefore, in this article, we will focus onB+Tree的存储结构!我们平常所说的索引,如果没有特别指明,都是指B+Tree结构组织的索引.
2.2 二叉树
假如说MySQL的索引结构采用二叉树的数据结构,比较理想的结构如下:
如果主键是顺序插入的,则会形成一个单向链表,结构如下:
The so-called order is that each insertion is smaller than the previous node.,或者大,This will form a linked list
所以,如果选择二叉树作为索引结构,会存在以下缺点:
- 顺序插入时,会形成一个链表,查询性能大大降低.
- 大数据量情况下,层级较深,检索速度慢.
此时大家可能会想到,我们可以选择红黑树,红黑树是一颗自平衡二叉树,那这样即使是顺序插入数据,最终形成的数据结构也是一颗平衡的二叉树,结构如下:
但是,即使如此,由于红黑树也是一颗二叉树,所以也会存在一个缺点:
- 大数据量情况下,层级较深,检索速度慢.
所以,在MySQL的索引结构中,并没有选择二叉树或者红黑树,而选择的是B+Tree,那么什么是B+Tree呢?在详解B+Tree之前,先来介绍一个B-Tree.
2.3 B-Tree
在说B+Tree之前,我们先了解一下B-Tree,B-Tree又被称之为B树,而B+Tree是B-Tree的变种,B树是一种多叉路平衡查找树,相对于二叉树,B树每个节点可以有多个分支,即多叉.
以一颗最大度数(max-degree)为5(5阶)的b-tree为例,那这个B树每个节点最多存储4个key,5个指针,指针永远比key最多多1个:
知识小贴士: 树的度数指的是一个节点的子节点个数.
我们可以通过一个数据结构可视化的网站来简单演示一下.https://www.cs.usfca.edu/~galles/visualization/BTree.html
插入一组数据: 100 65 169 368 900 556 780 35 215 1200 234 888 158 90 1000 88 120 268 250 .然后观察一些数据插入过程中,节点的变化情况.
Insert data process one:Since it is set to fifth order,Up to five levels of storage4个key,5个指针,一旦节点存储的key数量到达5,就会裂变.
Insert data process two:fission directly,中间元素向上分裂
Insert data process three:
Insert data process four:这时候会发现556Put it in the middle of the right node,因为B-TREE是有序的
Here is the final result,I won't show any more,It is strongly recommended that you go to the website and insert it yourself,This allows for better familiarity with the data structure!
B-Tree特点:
- 5阶的B树,每一个节点最多存储4个key,对应5个指针.
- 一旦节点存储的key数量到达5,就会裂变,中间元素向上分裂.
- 在B树中,非叶子节点和叶子节点都会存放数据.
2.4 B+Tree
B+Tree是B-Tree的变种,我们以一颗最大度数(max-degree)为4(4阶)的b+tree为例,来看一下其结构示意图:
我们可以看到,两部分:
- 绿色框框起来的部分,是索引部分,仅仅起到索引数据的作用,不存储数据.
- 红色框框起来的部分,是数据存储部分,在其叶子节点中要存储具体的数据.
我们可以通过一个数据结构可视化的网站来简单演示一下.
https://www.cs.usfca.edu/~galles/visualization/BPlusTree.html
插入一组数据: 100 65 169 368 900 556 780 35 215 1200 234 888 158 90 1000 88 120 268 250 .然后观察一些数据插入过程中,节点的变化情况.
The following is the result of the final insertion:
最终我们看到,B+Tree 与 B-Tree相比,主要有以下三点区别:
- 所有的数据都会出现在叶子节点.
- 叶子节点形成一个单向链表.
- 非叶子节点仅仅起到索引数据作用,具体的数据都是在叶子节点存放的.
上述我们所看到的结构是标准的B+Tree的数据结构,接下来,我们再来看看MySQL中优化之后的B+Tree.
MySQL索引数据结构对经典的B+Tree进行了优化.在原B+Tree的基础上,增加一个指向相邻叶子节点的链表指针
,就形成了带有顺序指针的B+Tree,提高区间访问的性能,利于排序.
mysqlOne page representsB+TREEA leaf node in a data structure,and a page has a fixed size of16kb.
总结:mysql的B+Tree数据结构,就是在原来的B+Tree结构基础上,Changed the singly linked list of leaf nodes to a doubly linked list
2.5 Hash
MySQL中除了支持B+Tree索引,还支持一种索引类型—Hash索引.
(1) 结构
哈希索引就是采用一定的hash算法,将键值换算成新的hash值,映射到对应的槽位上,然后存储在hash表中.
以下图为例:将name设置为hash索引,If want to find the correspondingname数据,首先会对name进行hashCalculates a subscript value,Get the corresponding data by subscripting the value.
如果两个(或多个)键值,映射到一个相同的槽位上,他们就产生了hash冲突(也称为hash碰撞),可以通过链表来解决.
(2) 特点
- Hash索引只能用于对等比较(=,in),不支持范围查询(between,>,< ,…)
- 无法利用索引完成排序操作
- 查询效率高,通常(不存在hash冲突的情况)只需要一次检索就可以了,效率通常要高于B+tree索 引
(3) 存储引擎支持
在MySQL中,支持hash索引的是Memory存储引擎. 而InnoDB中具有自适应hash功能,hash索引是InnoDB存储引擎根据B+Tree索引在指定条件下自动构建的.
思考题: 为什么InnoDB存储引擎选择使用B+tree索引结构?
- 相对于二叉树,层级更少,搜索效率高;
- 对于B-tree,无论是叶子节点还是非叶子节点,都会保存数据,这样导致一页中存储的键值减少,指针跟着减少,要同样保存大量数据,只能增加树的高度,导致性能降低;
- 相对Hash索引,B+tree支持范围匹配及排序操作;
3.索引分类
3.1 索引分类
在MySQL数据库,将索引的具体类型主要分为以下几类:主键索引、唯一索引、常规索引、全文索引.
Classification index structure is actually the index type,They are the same thing,没有区别!
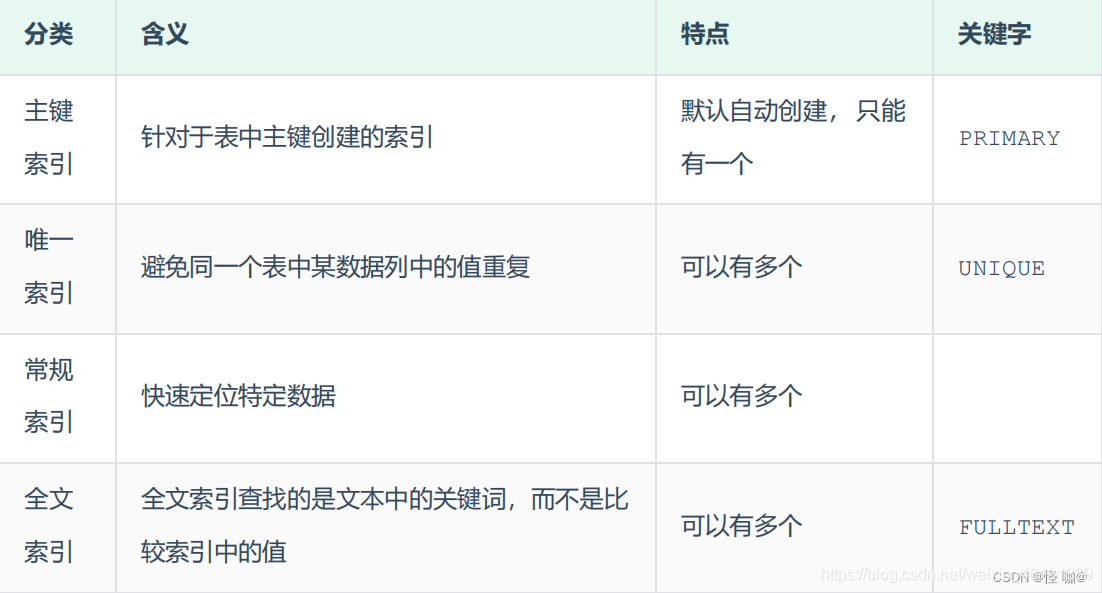
索引分类,也可以在NavcatOf the client,View available index types!
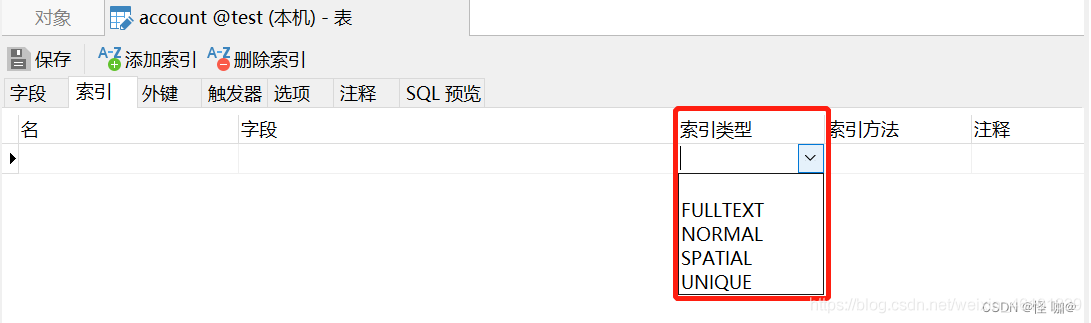
3.2 聚集索引&二级索引
而在在InnoDB存储引擎中,根据索引的存储形式,又可以分为以下两种:
Clustered indexes and secondary indexes are not the same as the index types we mentioned above,Don't get confused!
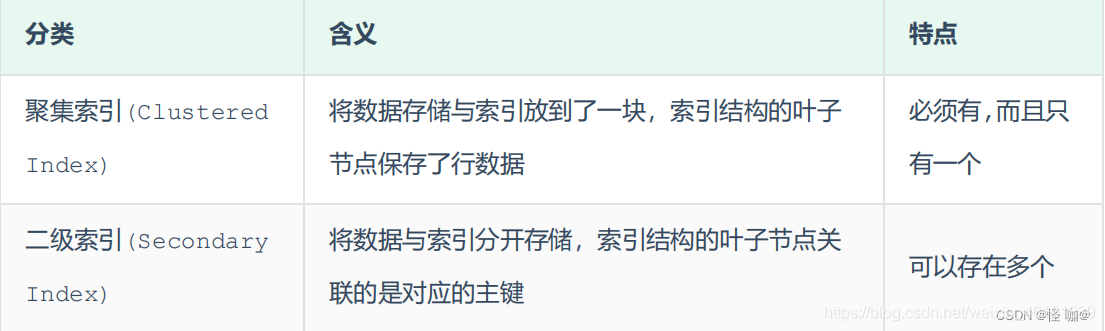
聚集索引选取规则:
- 如果存在主键,主键索引就是聚集索引.
- 如果不存在主键,将使用第一个唯一(UNIQUE)索引作为聚集索引.
- 如果表没有主键,或没有合适的唯一索引,则InnoDB会自动生成一个rowid作为隐藏的聚集索 引.
基于B+Tree的索引类型,聚集索引和二级索引的具体结构如下:
假如有一张表,There are three fields in the tableid、name、gender,然后id为主键,Then he is the clustered index,And then we givenamealso added an index,Then he is called the secondary index.
- 聚集索引的叶子节点下挂的是这一行的数据 .
- 二级索引的叶子节点下挂的是该字段值对应的主键值.
接下来,我们来分析一下,当我们执行如下的SQL语句时,具体的查找过程是什么样子的.
具体过程如下:
- 由于是根据name字段进行查询,所以先根据name='Arm’到name字段的二级索引中进行匹配查找.但是在二级索引中只能查找到 Arm 对应的主键值 10.
- 由于查询返回的数据是*,所以此时,还需要根据主键值10,到聚集索引中查找10对应的记录,最终找到10对应的行row.
- 最终拿到这一行的数据,直接返回即可.
回表查询: 这种先到二级索引中查找数据,找到主键值,然后再到聚集索引中根据主键值,获取数据的方式,就称之为回表查询.
思考题:
(1)以下两条SQL语句,那个执行效率高? 为什么?
- select * from user where id = 10 ;
- select * from user where name = ‘Arm’ ;
备注: id为主键,name字段创建的有索引;
解答: A 语句的执行性能要高于B 语句.
因为A语句直接走聚集索引,直接返回数据. 而B语句需要先查询name字段的二级索引,然后再查询聚集索引,也就是需要进行回表查询.
(2)InnoDB主键索引的B+tree高度为多高呢?
Not very knowledgeable about pages,建议看看mysql存储引擎篇:
https://blog.csdn.net/weixin_43888891/article/details/125958409?spm=1001.2014.3001.5501
一页最大为16KB,假设一行数据大小为1k,then one page can store16行这样的数据.InnoDB的指针占用6个字节的空间,主键假设为bigint类型,Then the number of bytes occupied is8.The number of pointers is the number of key values+1.
高度为2:
第一步:非叶子节点是不存储数据的,Then we can calculate how much the leaf node can store through the known existing conditionskey值.
n * 8 + (n + 1) * 6 = 16*1024
,算出n约为1170
(这里的n代表的就是key值,8represents the assumptionid为8字节,n+1代表的是指针,6Represents pointer occupation6字节,16*1024
Represents a page with a maximum of16kb*1024字节数)第二步:有了keyafter the number of values,通过key+1get the number of pointers,The number of pointers represents the maximum number of pages,Because the height is in itself2,then multiply by the page size,The maximum space is obtained as18736:
1171* 16 = 18736
也就是说,如果树的高度为2,Suppose a piece of data is1kb,则可以存储 18000 多条记录.
高度为3:
- 1171 * 1171 * 16 = 21939856
- There are as many pointers as there are pages,Because we are asking is for the biggest amount of data,So a pointer must correspond to a page
- 也就是说,如果树的高度为3,则可以存储 2200w 左右的记录.
4. 索引语法
创建索引:CREATE [ UNIQUE | FULLTEXT ] INDEX index_name ON table_name ( index_col_name,... ) ;
查看索引:SHOW INDEX FROM table_name ;
删除索引:DROP INDEX index_name ON table_name ;
案例演示: 先来创建一张表 tb_user,并且查询测试数据.
CREATE TABLE tb_user (
id INT PRIMARY KEY auto_increment COMMENT '主键',
NAME VARCHAR ( 50 ) NOT NULL COMMENT '用户名',
phone VARCHAR ( 11 ) NOT NULL COMMENT '手机号',
email VARCHAR ( 100 ) COMMENT '邮箱',
profession VARCHAR ( 11 ) COMMENT '专业',
age TINYINT UNSIGNED COMMENT '年龄',
gender CHAR ( 1 ) COMMENT '性别 , 1: 男, 2: 女',
STATUS CHAR ( 1 ) COMMENT '状态',
createtime datetime COMMENT '创建时间'
) COMMENT '系统用户表';
INSERT INTO tb_user ( NAME, phone, email, profession, age, gender, STATUS, createtime )
VALUES ( '吕布', '17799990000', '[email protected]', '软件工程', 23, '1', '6', '2001-02-02 00:00:00' );
INSERT INTO tb_user ( NAME, phone, email, profession, age, gender, STATUS, createtime )
VALUES ( '曹操', '17799990001', '[email protected]', '通讯工程', 33, '1', '0', '2001-03-05 00:00:00' );
INSERT INTO tb_user ( NAME, phone, email, profession, age, gender, STATUS, createtime )
VALUES ( '赵云', '17799990002', '[email protected]', '英语', 34, '1', '2', '2002-03-02 00:00:00' );
INSERT INTO tb_user ( NAME, phone, email, profession, age, gender, STATUS, createtime )
VALUES ( '孙悟空', '17799990003', '[email protected]', '工程造价', 54, '1', '0', '2001-07-02 00:00:00' );
INSERT INTO tb_user ( NAME, phone, email, profession, age, gender, STATUS, createtime )
VALUES ( '花木兰', '17799990004', '[email protected]', '软件工程', 23, '2', '1', '2001-04-22 00:00:00' );
INSERT INTO tb_user ( NAME, phone, email, profession, age, gender, STATUS, createtime )
VALUES ( '大乔', '17799990005', '[email protected]', '舞蹈', 22, '2', '0', '2001-02-07 00:00:00' );
INSERT INTO tb_user ( NAME, phone, email, profession, age, gender, STATUS, createtime )
VALUES ( '露娜', '17799990006', '[email protected]', '应用数学', 24, '2', '0', '2001-02-08 00:00:00' );
INSERT INTO tb_user ( NAME, phone, email, profession, age, gender, STATUS, createtime )
VALUES ( '程咬金', '17799990007', '[email protected]', '化工', 38, '1', '5', '2001-05-23 00:00:00' );
INSERT INTO tb_user ( NAME, phone, email, profession, age, gender, STATUS, createtime )
VALUES ( '项羽', '17799990008', '[email protected]', '金属材料', 43, '1', '0', '2001-09-18 00:00:00' );
INSERT INTO tb_user ( NAME, phone, email, profession, age, gender, STATUS, createtime )
VALUES ( '白起', '17799990009', '[email protected]', '机械工程及其自动 化', 27, '1', '2', '2001-08-16 00:00:00' );
INSERT INTO tb_user ( NAME, phone, email, profession, age, gender, STATUS, createtime )
VALUES ( '韩信', '17799990010', '[email protected]', '无机非金属材料工 程', 27, '1', '0', '2001-06-12 00:00:00' );
INSERT INTO tb_user ( NAME, phone, email, profession, age, gender, STATUS, createtime )
VALUES ( '荆轲', '17799990011', '[email protected]', '会计', 29, '1', '0', '2001-05-11 00:00:00' );
INSERT INTO tb_user ( NAME, phone, email, profession, age, gender, STATUS, createtime )
VALUES ( '兰陵王', '17799990012', '[email protected]', '工程造价', 44, '1', '1', '2001-04-09 00:00:00' );
INSERT INTO tb_user ( NAME, phone, email, profession, age, gender, STATUS, createtime )
VALUES ( '狂铁', '17799990013', '[email protected]', '应用数学', 43, '1', '2', '2001-04-10 00:00:00' );
INSERT INTO tb_user ( NAME, phone, email, profession, age, gender, STATUS, createtime )
VALUES ( '貂蝉', '17799990014', '[email protected]', '软件工程', 40, '2', '3', '2001-02-12 00:00:00' );
INSERT INTO tb_user ( NAME, phone, email, profession, age, gender, STATUS, createtime )
VALUES ( '妲己', '17799990015', '[email protected]', '软件工程', 31, '2', '0', '2001-01-30 00:00:00' );
INSERT INTO tb_user ( NAME, phone, email, profession, age, gender, STATUS, createtime )
VALUES ( '芈月', '17799990016', '[email protected]', '工业经济', 35, '2', '0', '2000-05-03 00:00:00' );
INSERT INTO tb_user ( NAME, phone, email, profession, age, gender, STATUS, createtime )
VALUES ( '嬴政', '17799990017', '[email protected]', '化工', 38, '1', '1', '2001-08-08 00:00:00' );
INSERT INTO tb_user ( NAME, phone, email, profession, age, gender, STATUS, createtime )
VALUES ( '狄仁杰', '17799990018', '[email protected]', '国际贸易', 30, '1', '0', '2007-03-12 00:00:00' );
INSERT INTO tb_user ( NAME, phone, email, profession, age, gender, STATUS, createtime )
VALUES ( '安琪拉', '17799990019', '[email protected]', '城市规划', 51, '2', '0', '2001-08-15 00:00:00' );
INSERT INTO tb_user ( NAME, phone, email, profession, age, gender, STATUS, createtime )
VALUES ( '典韦', '17799990020', '[email protected]', '城市规划', 52, '1', '2', '2000-04-12 00:00:00' );
INSERT INTO tb_user ( NAME, phone, email, profession, age, gender, STATUS, createtime )
VALUES ( '廉颇', '17799990021', '[email protected]', '土木工程', 19, '1', '3', '2002-07-18 00:00:00' );
INSERT INTO tb_user ( NAME, phone, email, profession, age, gender, STATUS, createtime )
VALUES ( '后羿', '17799990022', '[email protected]', '城市园林', 20, '1', '0', '2002-03-10 00:00:00' );
INSERT INTO tb_user ( NAME, phone, email, profession, age, gender, STATUS, createtime )
VALUES ( '姜子牙', '17799990023', '[email protected]', '工程造价', 29, '1', '4', '2003-05-26 00:00:00' );
数据准备好了之后,接下来,我们就来完成如下需求:
- name字段为姓名字段,该字段的值可能会重复,为该字段创建索引:
CREATE INDEX idx_user_name ON tb_user(name);
- phone手机号字段的值,是非空,且唯一的,为该字段创建唯一索引:
CREATE UNIQUE INDEX idx_user_phone ON tb_user(phone);
- 为profession、age、status创建联合索引(The created index specifies multiple columns at the same time,We call it a joint index or a composite index):
CREATE INDEX idx_user_pro_age_sta ON tb_user(profession,age,status);
- 为email建立合适的索引来提升查询效率:
CREATE INDEX idx_email ON tb_user(email);
完成上述的需求之后,我们再查看tb_user表的所有的索引数据:
5. SQL性能分析
5.1 SQL执行频率
MySQL 客户端连接成功后,通过 show [session|global] status
命令可以提供服务器状态信息.通过如下指令,可以查看当前数据库的INSERT、UPDATE、DELETE、SELECT的访问频次:
-- session 是查看当前会话 ;
-- global 是查询全局数据 ;
SHOW GLOBAL STATUS LIKE 'Com_______';
- Com_delete: 删除次数
- Com_insert: 插入次数
- Com_select: 查询次数
- Com_update: 更新次数
我们可以在当前数据库再执行几次查询操作,然后再次查看执行频次,看看 Com_select 参数会不会变化.
通过上述指令,我们可以查看到当前数据库到底是以查询为主,还是以增删改为主,从而为数据库优化提供参考依据. 如果是以增删改为主,我们可以考虑不对其进行索引的优化. 如果是以查询为主,那么就要考虑对数据库的索引进行优化了.
那么通过查询SQL的执行频次,我们就能够知道当前数据库到底是增删改为主,还是查询为主. 那假如说是以查询为主,我们又该如何定位针对于那些查询语句进行优化呢? 次数我们可以借助于慢查询日志.
接下来,我们就来介绍一下MySQL中的慢查询日志.
5.2 慢查询日志
慢查询日志记录了所有执行时间超过指定参数(long_query_time,单位:秒,默认10秒)的所有SQL语句的日志.
MySQL的慢查询日志默认没有开启,我们可以查看一下系统变量 slow_query_log
show variables like 'slow_query_log';
如果要开启慢查询日志,需要在MySQL的配置文件(如果是linux则是/etc/my.cnf,如果是windows在mysql安装目录下的my.ini)中配置如下信息:Note that the configuration must be loaded at the end of the configuration file,不然可能会出现问题!
这里我用的是mysql5.5版本,然后是在windowsThe following parameters are configured under,并没有出现问题,If you have a problem,那么可能mysqlVariable names changed between versions!
ft_min_word_len=1
#Slow query log storage path address
log-slow-queries = D:\slow.log
#Slow query record time
long_query_time = 2
测试: 配置完毕之后,重新启动MySQL服务器进行测试,查看慢日志文件中记录的信息.
mysqlGenerate millions of data quickly:https://blog.csdn.net/weixin_43888891/article/details/121223778
(1)执行如下SQL语句 :
-- 这条SQL执行效率比较高, 执行耗时 0.00sec
select * from tb_user;
-- 由于t_user表中, 预先存入了700w的记录, count一次,耗时6s
select count(*) from t_user;
(2)检查慢查询日志
最终我们发现,在慢查询日志中,只会记录执行时间超多我们预设时间(2s)的SQL,执行较快的SQL是不会记录的.
那这样,通过慢查询日志,就可以定位出执行效率比较低的SQL,从而有针对性的进行优化.
5.3 profile详情
show profiles 能够在做SQL优化时帮助我们了解时间都耗费到哪里去了.通过have_profiling参数,能够看到当前MySQL是否支持profile操作:SELECT @@have_profiling ;
profileThe queried data is isolated from each client.
查看prifile是否开启了:select @@profiling;
可以看到,当前MySQL是支持 profile操作的,但是开关是关闭的.可以通过set语句在session/global级别开启profiling:
SET profiling = 1;
开关已经打开了,接下来,我们所执行的SQL语句,都会被MySQL记录,并记录执行时间消耗到哪儿去了. 我们直接执行如下的SQL语句:
select * from tb_user;
select * from tb_user where id = 1;
select * from tb_user where name = '白起';
select count(*) from t_user;
执行一系列的业务SQL的操作,然后通过如下指令查看指令的执行耗时:
- 查看每一条SQL的耗时基本情况:
show profiles;
- 查看指定query_id的SQL语句各个阶段的耗时情况:
show profile for query query_id;
- 查看指定query_id的SQL语句CPU的使用情况:
show profile cpu for query query_id;
查看每一条SQL的耗时情况:
查看指定SQL各个阶段的耗时情况 :
5.4 explain
EXPLAIN 或者 DESC命令获取 MySQL 如何执行 SELECT 语句的信息,包括在 SELECT 语句执行过程中表如何连接和连接的顺序.
语法:
-- 直接在select语句之前加上关键字
explain / desc EXPLAIN SELECT 字段列表 FROM 表名 WHERE 条件 ;
Explain 执行计划中各个字段的含义:
6. 索引使用
6.1 验证索引效率
在讲解索引的使用原则之前,先通过一个简单的案例,来验证一下索引,看看是否能够通过索引来提升数据查询性能.在演示的时候,我们还是使用之前准备的一张表 t_user, 在这张表中准备了700w的记录.
这张表中id为主键,有主键索引,而其他字段是没有建立索引的. 我们先来查询其中的一条记录,看看里面的字段情况,执行如下SQL:
可以看到即使有1000w的数据,根据id进行数据查询,性能依然很快,因为主键id是有索引的. 那么接下来,我们再来根据 c_name字段进行查询,执行如下SQL:
我们可以看到根据c_name字段进行查询,查询返回了一条数据,结果耗时 3.52 sec,就是因为c_name没有索引,而造成查询效率很低.
那么我们可以针对于c_name字段,建立一个索引,建立了索引之后,我们再次根据c_name进行查询,再来看一下查询耗时情况.
创建索引:create index idx_user_cname on t_user(c_name);
然后再次执行相同的SQL语句,再次查看SQL的耗时.
我们明显会看到,c_name字段建立了索引之后,查询性能大大提升.建立索引前后,查询耗时都不是一个数量级的.
6.2 最左前缀法则
如果索引了多列(联合索引),要遵守最左前缀法则.最左前缀法则指的是查询从索引的最左列开始,并且不跳过索引中的列.如果跳跃某一列,索引将会部分失效(后面的字段索引失效)
.
以 tb_user 表为例,我们先来查看一下之前 tb_user 表所创建的索引.
在 tb_user 表中,有一个联合索引,这个联合索引涉及到三个字段,顺序分别为:profession,age,status.
对于最左前缀法则指的是,查询时,最左变的列,也就是profession必须存在,否则索引全部失效.而且中间不能跳过某一列,否则该列后面的字段索引将失效.
(1)The first step is to demonstrate the successful case of indexing,看一下具体的执行计划:
explain select * from tb_user where profession = '软件工程' and age = 31 and status = '0';
explain select * from tb_user where profession = '软件工程' and age = 31;
explain select * from tb_user where profession = '软件工程';
以上的这三组测试中,我们发现
只要联合索引最左边的字段 profession存在,索引就会生效
,只不过索引的长度不同. 而且由以上三组测试,我们也可以推测出profession字段索引长度为36、age字段索引长度为2、status字段索引长度为4.Next, we mainly judge which index in the combined index is not valid according to this length.!
注意:如果看不懂explain执行计划的,一定要看explaminIntroduced parameter explanation!Different database encodings and database versions maykey_lenlength will vary,这里我用的mysql是5.5版本,表用的是utf-8编码
(2)Below we demonstrate the leftmostprofessionWhen the column is not used,索引失效的情况:
explain select * from tb_user where age = 31 and status = '0';
explain select * from tb_user where status = '0';
而通过上面的这两组测试,我们也可以看到索引并未生效
,原因是因为不满足最左前缀法则,联合索引最左边的列profession不存在.
(3)Below we demonstrate skipping the middle column,索引失效的情况:
explain select * from tb_user where profession = '软件工程' and status = '0';
上述的SQL查询时,存在profession字段,最左边的列是存在的,索引满足最左前缀法则的基本条件.但是查询时,跳过了age这个列,所以后面的列索引是不会使用的,也就是索引部分生效,所以索引的长度就是36.
思考题:当执行SQL语句:
explain select * from tb_user where age = 31 and status = '0' and profession = '软件工程';
时,是否满足最左前缀法则,走不走上述的联合索引,索引长度?
可以看到,是完全满足最左前缀法则的,索引长度42,联合索引是生效的.
注意 : 最左前缀法则中指的最左边的列,是指在查询时,联合索引的最左边的字段(即是第一个字段)必须存在,与我们编写SQL时,条件编写的先后顺序无关.
6.3 索引失效情况
6.3.1 ><范围查询
联合索引中,出现范围查询(>,<),范围查询右侧的列索引失效.
explain select * from tb_user where profession = '软件工程' and age > 30 and status = '0';
当范围查询使用> 或 < 时,走联合索引了,但是索引的长度为38,就说明范围查询右边的status字段是没有走索引的.
explain select * from tb_user where profession = '软件工程' and age >= 30 and status = '0';
当范围查询使用>= 或 <= 时,走联合索引了,但是索引的长度为42,就说明所有的字段都是走索引
的.
所以,在业务允许的情况下,尽可能的使用类似于 >= 或 <= 这类的范围查询,而避免使用 > 或 < .
6.3.2 索引列运算
不要在索引列上进行运算操作, 索引将失效.
在tb_user表中,除了前面介绍的联合索引之外,还有一个索引,是phone字段的单列索引.
当根据phone字段进行等值匹配查询时, 索引生效.
explain select * from tb_user where phone = '17799990015';
当根据phone字段进行函数运算操作之后,索引失效.
explain select * from tb_user where substring(phone,10,2) = '15';
6.3.3 字符串不加引号
字符串类型字段使用时,不加引号,索引将失效.
接下来,我们通过两组示例,来看看对于字符串类型的字段,加单引号与不加单引号的区别.
第一组:
explain select * from tb_user where profession = '软件工程' and age = 31 and status = '0';
explain select * from tb_user where profession = '软件工程' and age = 31 and status = 0;
第二组:
explain select * from tb_user where phone = '17799990015';
explain select * from tb_user where phone = 17799990015;
6.3.4 模糊查询
如果仅仅是尾部模糊匹配,索引不会失效.如果是头部模糊匹配,索引失效.
接下来,我们来看一下这三条SQL语句的执行效果,查看一下其执行计划:
由于下面查询语句中,都是根据profession字段查询,符合最左前缀法则,联合索引是可以生效的,我们主要看一下,模糊查询时,%加在关键字之前,和加在关键字之后的影响.
explain select * from tb_user where profession like ‘软件%’;
explain select * from tb_user where profession like ‘%工程’;
explain select * from tb_user where profession like ‘%工%’;
经过上述的测试,我们发现,在like模糊查询中,在关键字后面加%,索引可以生效.而如果在关键字前面加了%,索引将会失效.
6.3.5 or连接条件
用or分割开的条件, 如果or前的条件中的列有索引,而后面的列中没有索引,那么涉及的索引都不会被用到.
explain select * from tb_user where id = 10 or age = 23;
explain select * from tb_user where phone = ‘17799990017’ or age = 23;
由于age没有索引,所以即使id、phone有索引,索引也会失效.所以需要针对于age也要建立索引.
然后,我们可以对age字段建立索引:create index idx_user_age on tb_user(age);
建立了索引之后,我们再次执行上述的SQL语句,看看前后执行计划的变化.
这里我发现一个问题,我用的mysql5.5版本orregardless of whether there are indexes on both sides,Don't walk index directly,但是又用了mysql 8测试了一下,.当ageDon't go when no index index,When there is an index on both sides will indeed go index.
6.3.6 数据分布影响
如果MySQL评估使用索引比全表更慢,则不使用索引.
explain select * from tb_user where phone >= '17799990005';
explain select * from tb_user where phone >= '17799990015';
mysql5.5版本执行如下:
mysql8.0版本执行如下:
经过测试我们发现,在mysql8版本当中,相同的SQL语句,只是传入的字段值不同,The final execution plan is completely different,这是为什么呢?
就是因为MySQL 8 版本 在查询时,会评估使用索引的效率与走全表扫描的效率,如果走全表扫描更快,则放弃索引,走全表扫描. 因为索引是用来索引少量数据的,如果通过索引查询返回大批量的数据,则还不如走全表扫描来的快,此时索引就会失效.
接下来,我们再来看看 is null 与 is not null 操作是否走索引.
explain select * from tb_user where profession is null;
explain select * from tb_user where profession is not null;
接下来,我们做一个操作将profession字段值全部更新为null:update tb_user set profession = null;
然后,再次执行上述的两条SQL,查看SQL语句的执行计划.
最终我们看到,一模一样的SQL语句,先后执行了两次,结果查询计划是不一样的,为什么会出现这种现象,这是和数据库的数据分布有关系.查询时MySQL会评估,走索引快,还是全表扫描快,如果全表扫描更快,则放弃索引走全表扫描. 因此,is null 、is not null是否走索引,得具体情况具体分析,并不是固定的.
6.4 SQL提示
目前tb_user表的索引情况如下:
(1)执行SQL :
explain select * from tb_user where profession = '软件工程';
查询走了联合索引.
(2)执行SQL,创建profession的单列索引:
create index idx_user_pro on tb_user(profession);
创建单列索引后,再次执行A中的SQL语句,查看执行计划,看看到底走哪个索引.
测试结果,我们可以看到,possible_keys中 idx_user_pro_age_sta,idx_user_pro 这两个索引都可能用到,最终MySQL选择了idx_user_pro_age_sta索引.这是MySQL自动选择的结果.
那么,我们能不能在查询的时候,自己来指定使用哪个索引呢? 答案是肯定的,此时就可以借助于MySQL的SQL提示来完成. 接下来,介绍一下SQL提示.
SQL提示,是优化数据库的一个重要手段,简单来说,就是在SQL语句中加入一些人为的提示来达到优化操作的目的.
use index : 建议MySQL使用哪一个索引完成此次查询(仅仅是建议,mysql内部还会再次进行评估).
explain select * from tb_user use index(idx_user_pro) where profession = '软件工 程'
;
示例演示:
ignore index : 忽略指定的索引.
explain select * from tb_user ignore index(idx_user_pro) where profession = '软件工 程'
;
示例演示:
force index : 强制使用索引.
explain select * from tb_user force index(idx_user_pro) where profession = '软件工 程';
示例演示:
6.5 覆盖索引
尽量使用覆盖索引,减少select *. 那么什么是覆盖索引呢? 覆盖索引是指 查询使用了索引,并且需要返回的列,在该索引中已经全部能够找到 .To put it bluntly, it is to avoid back-to-table queries
接下来,我们来看一组SQL的执行计划,看看执行计划的差别,然后再来具体做一个解析.
explain select id, profession from tb_user where profession = ‘软件工程’ and age = 31 and status = ‘0’ ;
explain select id,profession,age, status from tb_user where profession = ‘软件工程’ and age = 31 and status = ‘0’ ;
explain select id,profession,age, status, name from tb_user where profession = ‘软 件工程’ and age = 31 and status = ‘0’ ;
explain select * from tb_user where profession = ‘软件工程’ and age = 31 and status = ‘0’;
mysql5.5执行结果:
mysql8.0执行结果:
从上述的执行计划我们可以看到,这四条SQL语句的执行计划前面所有的指标都是一样的,看不出来差异.但是此时,我们主要关注的是后面的Extra.
在5.5版本当中,前面两条SQL的结果为 Using where; Using Index ;
而后面两条SQL的结果为: Using where
.
在8.0版本当中,前面两条SQL的结果为 Using Index ;
而后面两条SQL的结果为: NULL
.
因为,在tb_user表中有一个联合索引 idx_user_pro_age_sta,该索引关联了三个字段profession、age、status,而这个索引也是一个二级索引,所以叶子节点下面挂的是这一行的主键id. 所以当我们查询返回的数据在 id、profession、age、status 之中,则直接走二级索引直接返回数据了. 如果超出这个范围,就需要拿到主键id,再去扫描聚集索引,再获取额外的数据了,这个过程就是回表. 而我们如果一直使用select * 查询返回所有字段值,很容易就会造成回表查询(除非是根据主键查询,此时只会扫描聚集索引).
表结构及索引示意图:
id是主键,是一个聚集索引. name字段建立了普通索引,是一个二级索引(辅助索引).
执行SQL : select * from tb_user where id = 2;
根据id查询,直接走聚集索引查询,一次索引扫描,直接返回数据,性能高.
执行SQL:selet id,name from tb_user where name = 'Arm';
执行SQL:selet id,name,gender from tb_user where name = 'Arm';
由于在name的二级索引中,不包含gender,所以,需要两次索引扫描,也就是需要回表查询,性能相对较差一点.
思考题: 一张表, 有四个字段(id, username, password, status), 由于数据量大, 需要对以下SQL语句进行优化, 该如何进行才是最优方案:
select id,username,password from tb_user where username ='zhangsan';
答案: 针对于 username, password建立联合索引, sql为:create index idx_user_name_pass on tb_user(username,password);
这样可以避免上述的SQL语句,在查询的过程中,出现回表查询.
6.6 前缀索引
当字段类型为字符串(varchar,text,longtext等)时,有时候需要索引很长的字符串,这会让索引变得很大,查询时,浪费大量的磁盘IO, 影响查询效率.此时可以只将字符串的一部分前缀,建立索引,这样可以大大节约索引空间,从而提高索引效率.
语法:create index idx_xxxx on table_name(column(n)) ;
示例: 为tb_user表的email字段,建立长度为5的前缀索引.
create index idx_email_5 on tb_user(email(5));
可以根据索引的选择性来决定,而选择性是指不重复的索引值(基数)和数据表的记录总数的比值,索引选择性越高则查询效率越高, 唯一索引的选择性是1,这是最好的索引选择性,性能也是最好的.
select count(distinct email) / count(*) from tb_user ;
select count(distinct substring(email,1,5)) / count(*) from tb_user ;
前缀索引的查询流程
6.7 单列索引与联合索引
- 单列索引:即一个索引只包含单个列.
- 联合索引:即一个索引包含了多个列.
我们先来看看 tb_user 表中目前的索引情况:在查询出来的索引中,既有单列索引,又有联合索引.
接下来,我们来执行一条SQL语句,看看其执行计划:
通过上述执行计划我们可以看出来,在and连接的两个字段 phone、name上都是有单列索引的,但是最终mysql只会选择一个索引,也就是说,只能走一个字段的索引,此时是会回表查询的.
紧接着,我们再来创建一个phone和name字段的联合索引来查询一下执行计划.
此时,查询时,就走了联合索引,而在联合索引中包含 phone、name的信息,在叶子节点下挂的是对应的主键id,所以查询是无需回表查询的.
在业务场景中,如果存在多个查询条件,考虑针对于查询字段建立索引时,建议建立联合索引,而非单列索引.
如果查询使用的是联合索引,具体的结构示意图如下:
7. 索引设计原则
- 针对于数据量较大,且查询比较频繁的表建立索引.
- 针对于常作为查询条件(where)、排序(order by)、分组(group by)操作的字段建立索引.
- 尽量选择区分度高的列作为索引,尽量建立唯一索引,区分度越高,使用索引的效率越高.
- 如果是字符串类型的字段,字段的长度较长,可以针对于字段的特点,建立前缀索引.
- 尽量使用联合索引,减少单列索引,查询时,联合索引很多时候可以覆盖索引,节省存储空间,避免回表,提高查询效率.
- 要控制索引的数量,索引并不是多多益善,索引越多,维护索引结构的代价也就越大,会影响增删改的效率.
create unique index idx_user_phone_name on tb_user(phone,name);
- 如果索引列不能存储NULL值,请在创建表时使用NOT NULL约束它.当优化器知道每列是否包含NULL值时,它可以更好地确定哪个索引最有效地用于查询.
This article requires mastery:
- 什么是索引?
- 索引是个什么样的数据结构呢?
- 为什么使用索引?
- Hash 索引和 B+ 树索引有什么区别或者说优劣呢?
- 什么是聚簇索引(一个)
- 说一说索引的底层实现?(一般就是指的B+Tree)
- 索引有哪些优缺点?
- 聚簇索引和非聚簇索引的区别(A non-clustered index is a secondary index)
- MySQL中有几种索引类型,可以简单说说吗?(主键,唯一,常规(Conventional is divided into multi-column and single-column,Multi-column is generally called a composite index),全文)
- 覆盖索引是什么? (Covering index refers to the query column as far as possible the column covered by the index,This avoids backtracking)
- 非聚簇索引一定会回表查询吗?(if it happensselectThe column is the index column used in the condition,Is not back to the table)
- 联合索引是什么?为什么需要注意联合索引中的顺序?(最左前缀法则)
- 创建的索引有没有被使用到?或者说怎么才可以知道这条语句运行很慢的原因?(使用explain)
- 索引什么情况下会失效?
- 为什么Mysql用B+树做索引而不用B-树或红黑树、二叉树?
- 索引在什么情况下遵循最左前缀的规则?(组合索引)
版权声明
本文为[[email protected]]所创,转载请带上原文链接,感谢
https://yzsam.com/2022/214/202208021302456647.html
边栏推荐
猜你喜欢
图论之Prim,最小生成树该怎么解?
JS中的闭包
How to improve the originality of self-media creation and create popular works?
短视频美食自媒体怎么做?5步教你快速上手
嵌入式系统驱动初级【2】——字符设备驱动基础上_基础框架
Get out of the machine learning world forever!
Win11怎么修改关机界面颜色?Win11修改关机界面颜色的方法
SQL Server 2019 installation error 0 x80004005 service there is no timely response to the start or control request a detailed solution
Intouch Historian历史曲线配置导入导出
PHP+MYSQL [Student Information Management System] (Minimalist Edition)
随机推荐
qt 编译报错 No rule to make target
RestTemplate 使用:设置请求头、请求体
最小割和对偶图(未完成)
Win11怎么修改关机界面颜色?Win11修改关机界面颜色的方法
SQL Server database generation and execution of SQL scripts
Custom mvc framework review
package.json and package-lock.json
To eliminate air bubbles to save the mushroom h5 small game source code
photo-sphere-viewer Chinese documentation
国产 GPU 创业潮 喧嚣下的资本游戏
SQL Server 2014 installation tutorial (nanny-level graphic tutorial)
GCC版本升级到指定版本
Good shooting js game source code
Markdown怎么加入emoji
无线振弦采集仪远程修改参数方式
【C语言】手撕循环结构 —— for语句
路由-嵌套路由
SQL Server 2019 installation error 0 x80004005 service there is no timely response to the start or control request a detailed solution
How to do short video food from the media?5 steps to teach you to get started quickly
【C语言】手撕循环结构 —— while语句