当前位置:网站首页>AI超清修复出黄家驹眼里的光、LeCun大佬《深度学习》课程生还报告、绝美画作只需一行代码、AI最新论文 | ShowMeAI资讯日报 #07.06
AI超清修复出黄家驹眼里的光、LeCun大佬《深度学习》课程生还报告、绝美画作只需一行代码、AI最新论文 | ShowMeAI资讯日报 #07.06
2022-07-06 16:46:00 【ShowMeAI】
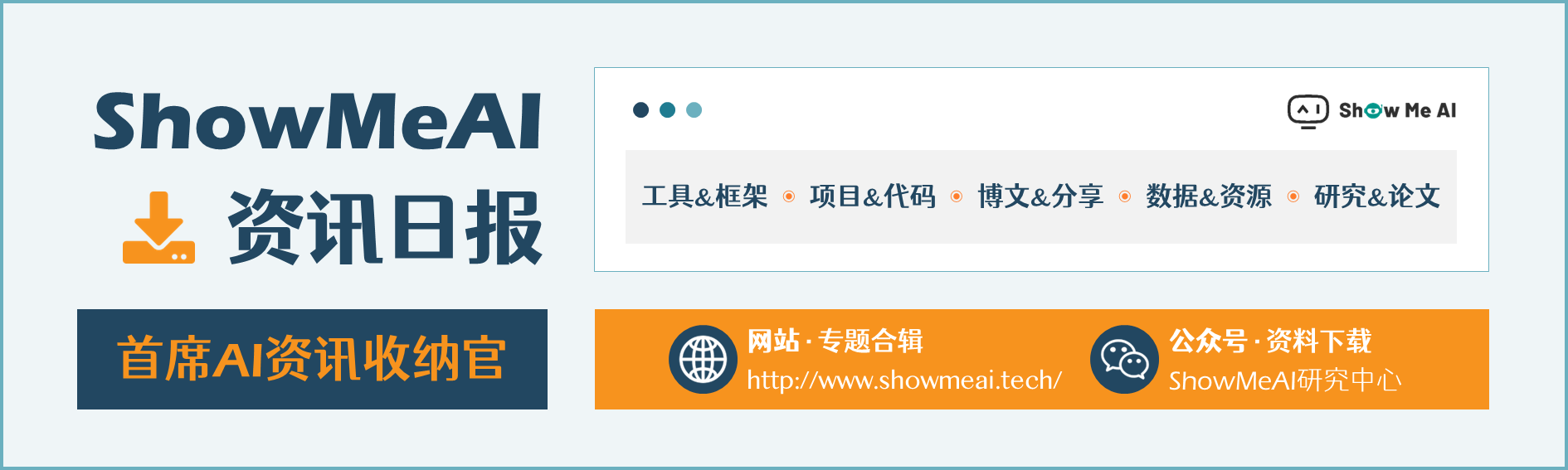
ShowMeAI日报系列全新升级!覆盖AI人工智能 工具&框架 | 项目&代码 | 博文&分享 | 数据&资源 | 研究&论文 等方向。点击查看 历史文章列表,在公众号内订阅话题 #ShowMeAI资讯日报,可接收每日最新推送。点击 专题合辑&电子月刊 快速浏览各专题全集。点击 这里 回复关键字 日报 免费获取AI电子月刊与资料包。
AI修复黄家驹31年前经典画面
7月3日晚,利用AI技术修复的Beyond『1991生命接触演唱会』,时隔31年在抖音平台重映。此次超清修复重映,将分辨率不足540p提升到接近4K,皮肤、笑容等细节全都清晰可见。
![]() ![]() |
工具&框架

【DiscoArt】一行代码使用Disco Diffusion生成艺术图
GitHub: https://github.com/jina-ai/discoart
Disco Diffusion 是一款 Google Colab Notebook 免费工具,使用 Python 语言由文本创建精美的图像和视频。DiscoArt 简化了这个过程,使得结果可复现、持久性、gRPC/HTTP服务、后期分析等更加简洁,帮助艺术工作者和AI爱好者等更优雅地创作。
![]() ![]() |
【Versatile Data Kit (VDK) 】使用SQL或Python知识构建数据流水线
GitHub: https://github.com/vmware/versatile-data-kit
VDK(多功能数据工具包)是一个开源框架,由 Data SDK 和 Control Service 两个套件组成。数据工程师可以同时使用SQL或Python语言,进行开发、部署、运行和管理数据作业。下图展示了数据流程及VDK发挥的作用。
![]() |
【The Nettle Magic Project】扑克条码扫描器,通过扫描扑克侧边组成的二维码确定每张牌的位置
GitHub: https://github.com/nettlep/magichttps://github.com/nettlep/magic
找到只能在特定红外条件下才能被识别的墨水,并对一副扑克牌的边缘进行标记。使用带有NoIR摄像机的树莓派Zero W可以检测到这些标记,配合iPad上运行的iOS客户端应用程序,就可以对扑克牌和红外标记进行清晰地识别。
![]() ![]() ![]() |
【wenet_trt8】用TRT8部署开源语音识别工具包
GitHub: https://github.com/huismiling/wenet_trt8
WeNet 是一款面向工业落地应用的语音识别工具包,模型由 encoder 和 decoder 两部分组成。本项目使用FP32模型(Pytorch直接导出的),使用ORT(ONNX Run Time)和PPQ两种量化方法对WeNet模型进行量化。
- 使用ORT量化:模型测试结果WER:6.06%,耗时:Encoder 7.34ms,Decoder 3.32ms。数据集效果下降1.46%,而Encoder加速2.5倍,Decoder加速6.3倍。
- 使用PPQ量化:模型测试结果WER:5.71%,耗时:Encoder 12.14ms,Decoder 2.68ms。数据集效果下降1.11%,而Encoder加速1.5倍,Decoder加速7.7倍。
![]() ![]() |
博文&分享

纽约大学《深度学习(2021) 》课程总结与学习笔记
笔记: https://dimgeo.com/posts/NYU-2021-DL-course-overview-and-notes/
课程: https://atcold.github.io/NYU-DLSP21/
NYU 2021《DEEP LEARNING》的讲师,一位是大名鼎鼎的 Yann LeCun,另一位是纽约大学计算机科学系的助理教授 Alfredo。课程约 50 小时,信息非常密集、涵盖广泛、并且包含了 Facebook AI 研究实验室客座讲师展示的CV和NLP方面的行业最新进展。
不过!要做好准备哦!LeCun 的课程不像 Andrew NG 吴恩达那样友好,且需要具备基本的机器学习知识和线性代数知识。
![]() ![]() |
《面向程序员的马尔可夫链》免费电子书
Github: https://github.com/czekster/markov
pdf: https://czekster.github.io/markov/MC-for-programmers2022.pdf
本书作者 Ricardo M. Czekster,讲解马尔可夫链及基本求解方法,包含Markov Chains、DTMC、CTMC等章节(目录见下方图片)。可以在GitHub页面找到本书的基础材料,例如C编程代码和解决方案、MATLAB脚本、本书提供的示例的棱柱模型(CTMC/DTMC)等。
![]() ![]() ![]() |
2022中国未来独角兽TOP100榜单
在第六届万物生长大会·中国未来独角兽大会上,《2022中国未来独角兽TOP100榜单》正式发布,聚焦企业服务、人工智能、新消费、先进制造、元宇宙5大领域,并在各领域精选出20家优质企业。榜单放在这里啦!供各位AI小可爱们参考~
![]() ![]() ![]() ![]() ![]() |
数据&资源

瑞士日内瓦大学《深度学习》课程视频及资料
UNIGE 14x050《Deep Learning》课程对深度学习进行了全面介绍,并附有 PyTorch 框架中的示例:
- 机器学习目标和主要挑战
- 张量运算
- 自动微分,梯度下降
- 深度学习特定技术
- 生成的、循环的、注意力模型
![]() ![]() |
人脸超分辨率 / hallucination 相关工作列表
GitHub: https://github.com/junjun-jiang/Face-Hallucination-Benchmark
Face-Hallucination-Benchmark:A collection of state-of-the-art face super-resolution/hallucination methods by Junjun Jiang
![]() ![]() |
研究&论文

可以点击 这里 回复关键字 日报,免费获取整理好的论文合辑。
公众号后台回复关键字 日报,免费获取整理好的论文合辑。
科研进展
2022年6月27日【深度学习】| Benchopt: Reproducible, efficient and collaborative optimization benchmarks
ICLR 2020【图神经网络】| Measuring and Improving the Use of Graph Information in Graph Neural Networks
2022年6月27日【自然语言处理】| Center-Embedding and Constituency in the Brain and a New Characterization of Context-Free Languages
2022年6月27日【自然语言处理】| Endowing Language Models with Multimodal Knowledge Graph Representations
2022年6月27日【机器学习】| Fast sequence to graph alignment using the graph wavefront algorithm
2022年6月27日【计算机视觉】| CLTS-GAN: Color-Lighting-Texture-Specular Reflection Augmentation for Colonoscopy
论文:Benchopt: Reproducible, efficient and collaborative optimization benchmarks
论文标题:Benchopt: Reproducible, efficient and collaborative optimization benchmarks
论文时间:27 Jun 2022
所属领域:深度学习
对应任务:Image Classification,Stochastic Optimization,图像分类,随机优化
论文地址:https://arxiv.org/abs/2206.13424
代码实现:https://github.com/benchopt/benchopt
论文作者:Thomas Moreau, Mathurin Massias, Alexandre Gramfort, Pierre Ablin, Pierre-Antoine Bannier, Benjamin Charlier, Mathieu Dagréou, Tom Dupré La Tour, Ghislain Durif, Cassio F. Dantas, Quentin Klopfenstein, Johan Larsson, En Lai, Tanguy Lefort, Benoit Malézieux, Badr Moufad, Binh T. Nguyen, Alain Rakotomamonjy, Zaccharie Ramzi, Joseph Salmon, Samuel Vaiter
论文简介:Numerical validation is at the core of machine learning research as it allows to assess the actual impact of new methods, and to confirm the agreement between theory and practice./数值验证是机器学习研究的核心,因为它可以评估新方法的实际影响,并确认理论和实践之间的一致性。
论文摘要:Numerical validation is at the core of machine learning research as it allows to assess the actual impact of new methods, and to confirm the agreement between theory and practice. Yet, the rapid development of the field poses several challenges: researchers are confronted with a profusion of methods to compare, limited transparency and consensus on best practices, as well as tedious re-implementation work. As a result, validation is often very partial, which can lead to wrong conclusions that slow down the progress of research. We propose Benchopt, a collaborative framework to automate, reproduce and publish optimization benchmarks in machine learning across programming languages and hardware architectures. Benchopt simplifies benchmarking for the community by providing an off-the-shelf tool for running, sharing and extending experiments. To demonstrate its broad usability, we showcase benchmarks on three standard learning tasks: ℓ2-regularized logistic regression, Lasso, and ResNet18 training for image classification. These benchmarks highlight key practical findings that give a more nuanced view of the state-of-the-art for these problems, showing that for practical evaluation, the devil is in the details. We hope that Benchopt will foster collaborative work in the community hence improving the reproducibility of research findings.
数值验证是机器学习研究的核心,因为它可以评估新方法的实际影响,并确认理论和实践之间的一致性。然而,该领域的快速发展带来了一些挑战:研究人员面临着大量需要比较的方法、有限的透明度和关于最佳实践的共识,以及繁琐的重新实施工作。因此,验证往往是非常片面的,这可能导致错误的结论,减缓研究的进展。我们提出了Benchopt,这是一个合作框架,用于自动化、复制和发布跨编程语言和硬件架构的机器学习的优化基准。Benchopt通过提供一个现成的工具来运行、分享和扩展实验,从而简化了社区的基准测试。为了证明其广泛的可用性,我们展示了三个标准学习任务的基准:ℓ2-regularized logistic regression, Lasso, 和ResNet18图像分类训练。这些基准突出了关键的实际发现,对这些问题的最先进水平给出了更细致的看法,表明对于实际评估来说,关键在细节中。我们希望Benchopt能够促进社区的合作,从而提高研究结果的可重复性。
![]() ![]() |
论文:Measuring and Improving the Use of Graph Information in Graph Neural Networks
论文标题:Measuring and Improving the Use of Graph Information in Graph Neural Networks
论文时间:ICLR 2020
所属领域:图神经网络
对应任务:Representation Learning,表征学习
论文地址:https://arxiv.org/abs/2206.13170
代码实现:https://github.com/yifan-h/CS-GNN
论文作者:Yifan Hou, Jian Zhang, James Cheng, Kaili Ma, Richard T. B. Ma, Hongzhi Chen, Ming-Chang Yang
论文简介:Graph neural networks (GNNs) have been widely used for representation learning on graph data./图神经网络(GNNs)已被广泛用于图数据的表示学习。
论文摘要:Graph neural networks (GNNs) have been widely used for representation learning on graph data. However, there is limited understanding on how much performance GNNs actually gain from graph data. This paper introduces a context-surrounding GNN framework and proposes two smoothness metrics to measure the quantity and quality of information obtained from graph data. A new GNN model, called CS-GNN, is then designed to improve the use of graph information based on the smoothness values of a graph. CS-GNN is shown to achieve better performance than existing methods in different types of real graphs.
图神经网络(GNNs)已被广泛用于图数据的表示学习。然而,人们对GNNs从图数据中实际获得多少性能了解有限。本文介绍了一个基于上下文的GNN框架,并提出了两个平滑度指标来衡量从图数据中获得信息的数量和质量。然后设计了一个新的GNN模型,称为CS-GNN,以改善基于图平滑度值的图信息的使用。CS-GNN被证明在不同类型的真实图中取得了比现有方法更好的性能。
![]() ![]() |
论文:Center-Embedding and Constituency in the Brain and a New Characterization of Context-Free Languages
论文标题:Center-Embedding and Constituency in the Brain and a New Characterization of Context-Free Languages
论文时间:27 Jun 2022
所属领域:自然语言处理
对应任务:Dependency Parsing,依存解析
论文地址:https://arxiv.org/abs/2206.13217
代码实现:https://github.com/dmitropolsky/assemblies
论文作者:Daniel Mitropolsky, Adiba Ejaz, Mirah Shi, Mihalis Yannakakis, Christos H. Papadimitriou
论文简介:We address two of the most important questions left open by that work: constituency (the identification of key parts of the sentence such as the verb phrase) and the processing of dependent sentences, especially center-embedded ones./我们讨论了这项工作留下的两个最重要的问题:构词法(识别句子的关键部分,如动词短语)和从属句的处理,特别是中心嵌入的句子。
论文摘要:A computational system implemented exclusively through the spiking of neurons was recently shown capable of syntax, that is, of carrying out the dependency parsing of simple English sentences. We address two of the most important questions left open by that work: constituency (the identification of key parts of the sentence such as the verb phrase) and the processing of dependent sentences, especially center-embedded ones. We show that these two aspects of language can also be implemented by neurons and synapses in a way that is compatible with what is known, or widely believed, about the structure and function of the language organ. Surprisingly, the way we implement center embedding points to a new characterization of context-free languages.
最近,一个完全通过神经元实现的计算系统被证明能够进行语法,即进行简单英语句子的依存分析。我们讨论了这项工作留下的两个最重要的问题:构词法(识别句子的关键部分,如动词短语)和从属句的处理,特别是中心嵌入的句子。我们表明,语言的这两个方面也可以由神经元和突触来实现,其方式与已知的或广泛认为的语言器官的结构和功能相一致。令人惊讶的是,我们实现中心嵌入的方式指向了无语境语言的新特征。
![]() |
论文:Endowing Language Models with Multimodal Knowledge Graph Representations
论文标题:Endowing Language Models with Multimodal Knowledge Graph Representations
论文时间:27 Jun 2022
所属领域:自然语言处理
对应任务:Multilingual Named Entity Recognition,named-entity-recognition,+1
论文地址:https://arxiv.org/abs/2206.13163
代码实现:https://github.com/iacercalixto/visualsem
论文作者:Ningyuan Huang, Yash R. Deshpande, Yibo Liu, Houda Alberts, Kyunghyun Cho, Clara Vania, Iacer Calixto
论文简介:We use the recently released VisualSem KG as our external knowledge repository, which covers a subset of Wikipedia and WordNet entities, and compare a mix of tuple-based and graph-based algorithms to learn entity and relation representations that are grounded on the KG multimodal information./我们使用最近发布的VisualSem KG作为我们的外部知识库,它涵盖了维基百科和WordNet实体的一个子集,并比较了基于元组和基于图的混合算法来学习基于KG多模态信息的实体和关系表示。
论文摘要:We propose a method to make natural language understanding models more parameter efficient by storing knowledge in an external knowledge graph (KG) and retrieving from this KG using a dense index. Given (possibly multilingual) downstream task data, e.g., sentences in German, we retrieve entities from the KG and use their multimodal representations to improve downstream task performance. We use the recently released VisualSem KG as our external knowledge repository, which covers a subset of Wikipedia and WordNet entities, and compare a mix of tuple-based and graph-based algorithms to learn entity and relation representations that are grounded on the KG multimodal information. We demonstrate the usefulness of the learned entity representations on two downstream tasks, and show improved performance on the multilingual named entity recognition task by 0.3%–0.7% F1, while we achieve up to 2.5% improvement in accuracy on the visual sense disambiguation task. All our code and data are available in https://github.com/iacercalixto/visualsem-kg
我们提出了一种方法,通过在外部知识图谱(KG)中存储知识并使用密集索引从该KG中检索,使自然语言理解模型的参数效率更高。鉴于下游任务数据(可能是多语言的),例如德语句子,我们从KG中检索实体,并使用它们的多模态表示来提高下游任务的性能。我们使用最近发布的VisualSem KG作为我们的外部知识库,它涵盖了维基百科和WordNet实体的一个子集,并比较了基于元组和基于图的混合算法来学习基于KG多模态信息的实体和关系表示。我们在两个下游任务中证明了所学实体表征的有用性,并显示在多语言命名实体识别任务中的性能提高了0.3%-0.7% F1,而在视觉意义上的歧义任务中,我们实现了高达2.5%的准确性提高。我们所有的代码和数据都可以在以下网站找到 https://github.com/iacercalixto/visualsem-kg
![]() ![]() |
论文:Fast sequence to graph alignment using the graph wavefront algorithm
论文标题:Fast sequence to graph alignment using the graph wavefront algorithm
论文时间:27 Jun 2022
所属领域:机器学习
论文地址:https://arxiv.org/abs/2206.13574
代码实现:https://github.com/lh3/gwfa
论文作者:Haowen Zhang, Shiqi Wu, Srinivas Aluru, Heng Li
论文简介:Motivation: A pan-genome graph represents a collection of genomes and encodes sequence variations between them./一个泛基因组图表示一个基因组的集合,并对它们之间的序列变化进行编码。
论文摘要:Motivation: A pan-genome graph represents a collection of genomes and encodes sequence variations between them. It is a powerful data structure for studying multiple similar genomes. Sequence-to-graph alignment is an essential step for the construction and the analysis of pan-genome graphs. However, existing algorithms incur runtime proportional to the product of sequence length and graph size, making them inefficient for aligning long sequences against large graphs. Results: We propose the graph wavefront alignment algorithm (Gwfa), a new method for aligning a sequence to a sequence graph. Although the worst-case time complexity of Gwfa is the same as the existing algorithms, it is designed to run faster for closely matching sequences, and its runtime in practice often increases only moderately with the edit distance of the optimal alignment. On four real datasets, Gwfa is up to four orders of magnitude faster than other exact sequence-to-graph alignment algorithms. We also propose a graph pruning heuristic on top of Gwfa, which can achieve an additional ∼10-fold speedup on large graphs. Availability: Gwfa code is accessible at https://github.com/lh3/gwfa .
泛基因组图表示基因组的集合,并对它们之间的序列变化进行编码。它是一个强大的数据结构,用于研究多个相似的基因组。序列与图的比对是构建和分析泛基因组图的一个重要步骤。然而,现有的算法产生的运行时间与序列长度和图的大小的乘积成正比,使得它们在对大图进行长序列比对时效率低下。结果。我们提出了图形波前对齐算法(Gwfa),这是一种将序列与序列图对齐的新方法。尽管Gwfa的最坏情况下的时间复杂度与现有的算法相同,但它被设计为对紧密匹配的序列运行得更快,而且它在实践中的运行时间往往只随着最佳对齐的编辑距离而适度增加。在四个真实的数据集上,Gwfa比其他精确的序列到图的排列算法快四个数量级。我们还在Gwfa的基础上提出了一个启发式图修剪,它可以在大图上实现额外的∼10倍的速度提升。Gwfa代码可在 https://github.com/lh3/gwfa 获取。
![]() |
论文:CLTS-GAN: Color-Lighting-Texture-Specular Reflection Augmentation for Colonoscopy
论文标题:CLTS-GAN: Color-Lighting-Texture-Specular Reflection Augmentation for Colonoscopy
论文时间:29 Jun 2022
所属领域:计算机视觉
论文地址:https://arxiv.org/abs/2206.14951
代码实现:https://github.com/nadeemlab/CEP
论文作者:Shawn Mathew, Saad Nadeem, Arie Kaufman
论文简介:Automated analysis of optical colonoscopy (OC) video frames (to assist endoscopists during OC) is challenging due to variations in color, lighting, texture, and specular reflections./由于颜色、照明、纹理和镜面反射的变化,光学结肠镜(OC)视频帧的自动分析(在OC期间协助内窥镜医师)具有挑战性。
论文摘要:Automated analysis of optical colonoscopy (OC) video frames (to assist endoscopists during OC) is challenging due to variations in color, lighting, texture, and specular reflections. Previous methods either remove some of these variations via preprocessing (making pipelines cumbersome) or add diverse training data with annotations (but expensive and time-consuming). We present CLTS-GAN, a new deep learning model that gives fine control over color, lighting, texture, and specular reflection synthesis for OC video frames. We show that adding these colonoscopy-specific augmentations to the training data can improve state-of-the-art polyp detection/segmentation methods as well as drive next generation of OC simulators for training medical students. The code and pre-trained models for CLTS-GAN are available on Computational Endoscopy Platform GitHub https://github.com/nadeemlab/CEP .
由于颜色、照明、纹理和镜面反射的变化,光学结肠镜(OC)视频帧的自动分析(在OC期间协助内窥镜医生)具有挑战性。以前的方法要么通过预处理去除其中的一些变化(使处理流水线变得繁琐),要么增加带有注释的不同训练数据(但昂贵且耗时)。我们提出了CLTS-GAN,一个新的深度学习模型,对OC视频帧的颜色、照明、纹理和镜面反射合成进行精细控制。我们表明,在训练数据中加入这些结肠镜特有的增强功能,可以改善最先进的息肉检测/分割方法,并推动下一代结肠镜模拟器用于培训医学生。CLTS-GAN的代码和预训练模型可在计算内窥镜平台GitHub https://github.com/nadeemlab/CEP 上获得。
![]() |
我们是 ShowMeAI,致力于传播AI优质内容,分享行业解决方案,用知识加速每一次技术成长!点击查看 历史文章列表,在公众号内订阅话题 #ShowMeAI资讯日报,可接收每日最新推送。点击 专题合辑&电子月刊 快速浏览各专题全集。点击 这里 回复关键字 日报 免费获取AI电子月刊与资料包。

边栏推荐
- DAY FOUR
- Why should a complete knapsack be traversed in sequence? Briefly explain
- X.509 certificate based on go language
- Everyone is always talking about EQ, so what is EQ?
- GPIO简介
- The programmer resigned and was sentenced to 10 months for deleting the code. Jingdong came home and said that it took 30000 to restore the database. Netizen: This is really a revenge
- DAY FIVE
- 谷歌百度雅虎都是中国公司开发的通用搜索引擎_百度搜索引擎url
- PostgreSQL使用Pgpool-II实现读写分离+负载均衡
- Leecode brushes questions and records interview questions 01.02 Determine whether it is character rearrangement for each other
猜你喜欢
基于GO语言实现的X.509证书
DAY ONE
The programmer resigned and was sentenced to 10 months for deleting the code. Jingdong came home and said that it took 30000 to restore the database. Netizen: This is really a revenge
工程师如何对待开源 --- 一个老工程师的肺腑之言
2021 SASE integration strategic roadmap (I)
智能运维应用之道,告别企业数字化转型危机
What can the interactive slide screen demonstration bring to the enterprise exhibition hall
MVC and MVVM
准备好在CI/CD中自动化持续部署了吗?
37页数字乡村振兴智慧农业整体规划建设方案
随机推荐
在Docker中分分钟拥有Oracle EMCC 13.5环境
Operation test of function test basis
(leetcode) sum of two numbers
A way of writing SQL, update when matching, or insert
Wechat applet UploadFile server, wechat applet wx Uploadfile[easy to understand]
2022/2/11 summary
What is web penetration testing_ Infiltration practice
pytest多进程/多线程执行测试用例
St table
GPIO简介
Three sentences to briefly introduce subnet mask
DAY SIX
[automated testing framework] what you need to know about unittest
使用yum来安装PostgreSQL13.3数据库
DAY THREE
pinia 模块划分
X.509 certificate based on go language
GEO数据挖掘(三)使用DAVID数据库进行GO、KEGG富集分析
DAY FIVE
MIT 6.824 - Raft学生指南