After Luke zettlemoyer, head of meta AI Seattle research | trillion parameters, will the large model continue to grow?
2022-07-06 00:38:00
【Zhiyuan community】
Reading guide : The pre training language model is becoming larger and larger , While surprised at its powerful ability , People can't help asking : Will the scale of language models continue to grow in the future ?Meta AI Seattle Research Director , School of computer science and engineering, University of Washington Paul G. Allen professor Luke Zettlemoyer Published the title “Large Language Models: Will they keep getting bigger? And, how will we use them if they do?” Keynote speech , The related work of the team on large-scale language model is introduced .Zettlemoyer The professor discussed the following three issues : 1) Will the scale of the language model continue to grow 2) How to make the best use of language models 3) Other supervision methods of model pre training Arrangement : Hu Xueyu 、 Xiong Yuxuan
As the scale of the language model continues to grow ,「 Will the scale of the language model continue to grow 」、「 How to use language models 」 It has become the focus of researchers . The following figure shows the relationship between parameter size and time of the pre training language model ,x Axis represents time ,y The axis represents the parameter scale , Models are getting bigger .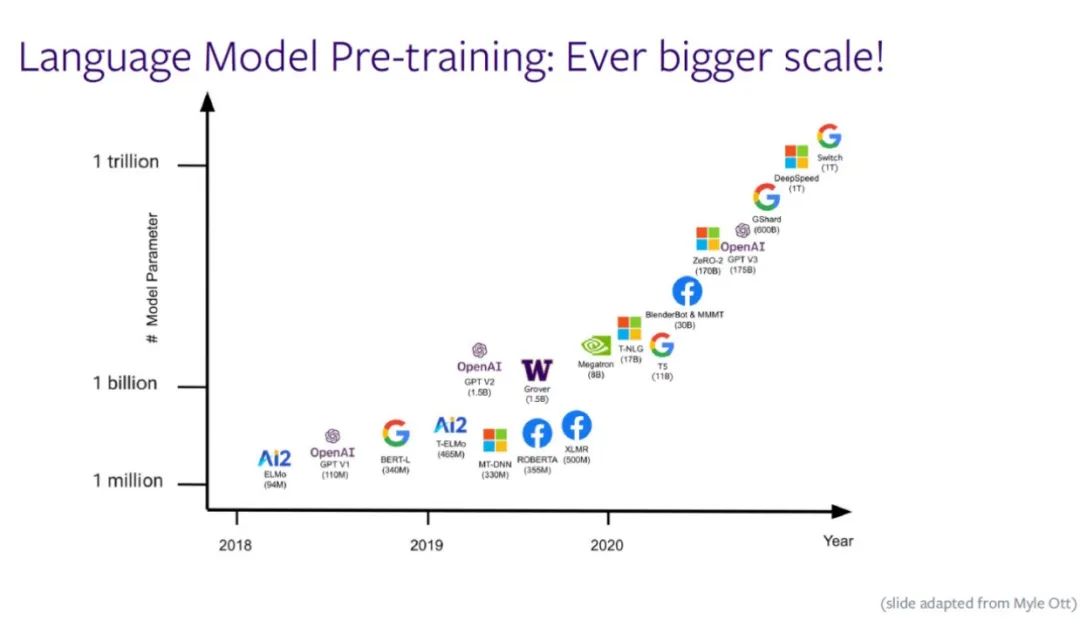
The language model contains the distribution of sentences , When people have language models , You can use the model to predict the possible words that may appear later according to the existing words . Despite the existence of such as BERT Such a two-way language model , This article only discusses the language model of processing words from left to right . With Open AI Of GPT Take a series of models as an example , The ability of zero sample learning enables the language model to handle any natural language processing (NLP) Mission . The model will NLP Tasks are converted into sequence to sequence tasks . for example , Enter a description of the task , The model classifies texts according to the knowledge learned before .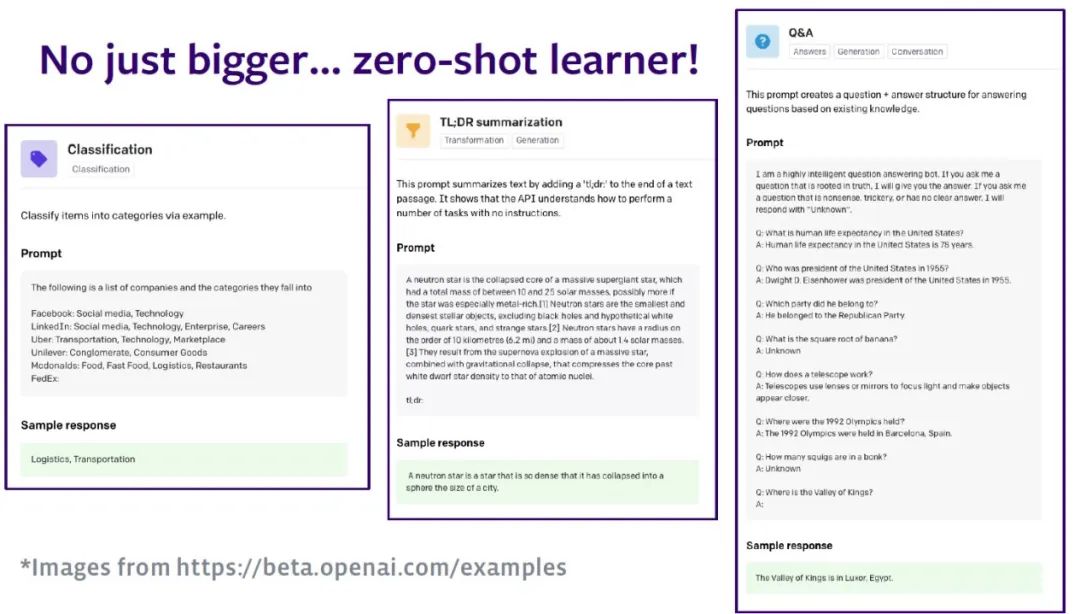
Will the scale of the language model continue to grow
As shown in the figure below , The parameters of the language model are approaching one trillion , It is more and more difficult for people to train all parameters at the same time . Because there is not enough data or computing power to support , The model is difficult to be fully trained . In the near future , Such as PaLM、Chinchilla Wait for the model to try to use less parameters 、 More data and arithmetic training models , Control the scale of the model .
Zettlemoyer Professor pointed out , If people really want to make the model bigger , Finally, we have to make some compromises : No longer choose to use large and dense Neural Networks , Instead, it adopts the idea of sparseness , Use different parts of the model to handle different inputs ( for example , Google's Switch Model ). Adopt even the most advanced GPU colony , The demand for computing power is still approaching the limit of computing equipment , Innovation must be achieved on the top-level architecture .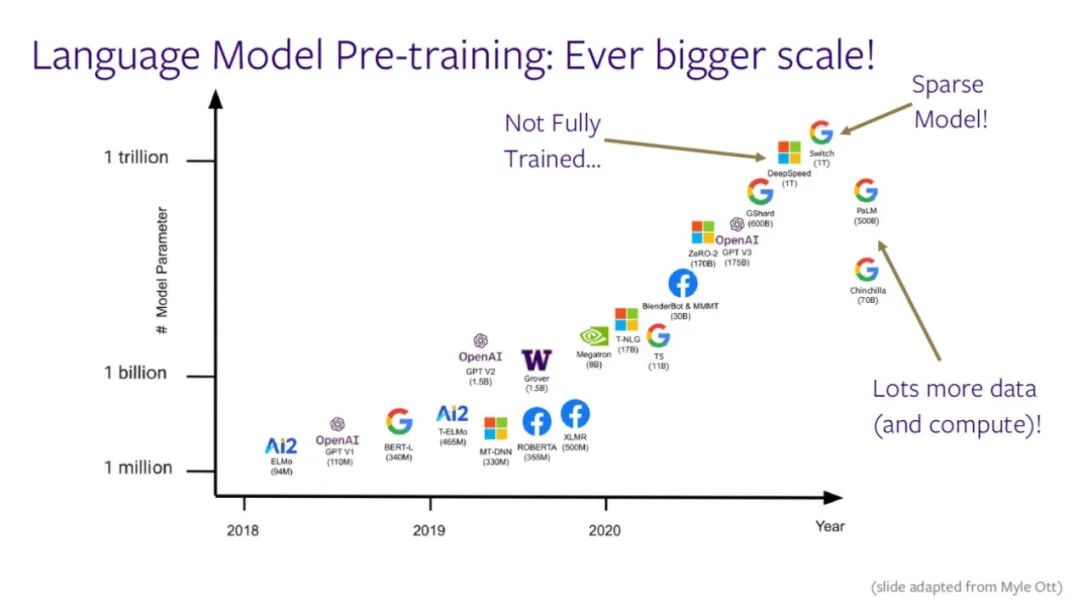
When training dense models , Any input will be thrown into the whole model , Every parameter will participate in the calculation . Researchers have proposed data parallelism 、 Model parallelism and pipeline parallelism to speed up computation , These calculations are deployed to GPU On different nodes of the cluster . For all that , For each input , The model still needs a lot of calculations , Especially for those large-scale models .Zettlemoyer Professor pointed out , Using sparse model for conditional calculation can only use some parameters of the model to participate in the calculation , Save a lot of computing resources .Transformer The structure includes self attention layer and full connection layer , Input is input into the full connection layer after completing the calculation in the self attention layer , When the scale of the language model expands , exceed 95% All parameters of are from the full connection layer ,Zettlemoyer The professor team tried to sparse the full connection layer . In adopting methods such as ReLU When the activation function of , In fact, the neural network is thinned , similarly , When training large-scale language models , People have made conditional calculations to some extent . But in practice , We cannot know in advance what part should be calculated , Nor can we use efficient matrix multiplication . So ,Zettlemoyer The professor team tried to cut part of the neural network , Divide the whole connection layer into several parts , Allocate different parts to different processors , And designed a specific routing mechanism .
The mixed expert model shown in the figure below (mixture of experts) in ,“Dogs bark” and “Cats purr” It will be input to the model during training . The model will route different words to different expert networks , After calculation, it will be rearranged into the original order .Zettlemoyer Professor pointed out , The real challenge is how to ensure performance , Improve the efficiency of this process . So ,Zettlemoyer The teaching team adopts expert specialization and expert equalization technology , Make different expert networks do different calculations , And realize the uniform distribution of calculation , Reach the saturation order of performance .
The standard practice is to add some loss functions in the training process to ensure balanced routing , Use capacity factor (capacity factor) Make sure there is no overload . This method introduces additional loss functions and hyperparameters , You need to make sure that the settings are stable .Zettlemoyer Where is the professor team 2021 It was proposed that BASE Layers, Its main characteristics are as follows :(1) No additional loss functions or hyperparameters are introduced (2) It can achieve data parallelism 90% The calculation speed of (3) Each word case is assigned to an expert (4) All experts are balanced and saturated . In the part of achieving expert balance , This model replaces the trainable equilibrium with the algorithm based equilibrium , Formalized as a linear assignment problem , And use auction algorithm to solve .
The figure above shows three different calculations , The effect of the pre training model measured by confusion . The lower the degree of confusion, the better the model training effect . The blue line in the figure represents BASE Layers The job of , It is equivalent to other dense models , When the model is larger, the effect is even better . Besides , The work and Sparse MoE The effect is quite , Slightly better than Switch.
Next ,Zettlemoyer The professor introduced Gururangan Et al. 2021 Years of work DEMix Layers. Researchers have collected a lot of documents , Let it be RoBERTa The obtained characterization is clustered according to similarity , The visualization results show that the language data is heterogeneous . As shown in the figure , Medical literature is mostly clustered in red clusters , But computer science literature and news can also be gathered , This shows that the data has its own structure . This inspires us to : The calculation process can be split based on the characteristics of data , So as to realize expert specialization .
DEMix Design domain specific experts , Fixed the routing process . Specific areas are handled by specific experts , And can be mixed when needed 、 Add or remove specific experts , Emphasize rapid adaptation . Model training , Suppose there is corresponding metadata indicating the field of data , There is no need for other forms of equalization . Through conditional calculation , Domain experts can be trained in parallel , Although there are more parameters than dense models , But training is faster . In order to verify the effect of the model , Researchers have collected data on some new fields . Confusion degree is also used as the evaluation index in the evaluation , The calculation time of all models is limited to 48 Within hours , The models for comparison include GTP-3 small, medium, large, XL Four kinds of .
Researchers assume that the fields to which the data belongs are known during training and testing . Experimental results show that ,DEMix It is more effective when the scale of the model is small , As the number of model parameters increases , Dense models and DEMix The gap is narrowing . Further observation of the experimental results on the largest model shows ,DEMix In the news and Reddit Performance on datasets is not as good as dense models , But how to divide the field is based on subjective judgment , Researchers use metadata here , We need to further explore how to divide the data field .- characteristic 1: Mixed experts
Researchers assume that they do not know the specific field of input , adopt DEMix Mix experts . One method is to take small-scale data and calculate it on all experts , Get the posterior distribution of the field , In this way, no additional parameters are needed to mix experts . Yes 100 Input sequence , Researchers visualized the distribution of domain experts in all fields , Every field expert is closely related to his field , But there are some more heterogeneous areas ( Such as WebText Data sets ) It also has a certain relationship with other fields . When researchers analyze the relevance of domain experts in new fields , It is found that this correlation is more scattered than those in the training set . It means that models tend to get information from different experts when they encounter new fields . Experimental results show that , Using mixed expert DEMix The model achieves the best results in new fields .- characteristic 2: Add experts
DEMix You can freeze other parts of the model , A posteriori distribution in the field of computing new data , Add a new expert and input some new data , Continue training from the parameters of the expert with the highest a posteriori probability in the existing field .- characteristic 3: Remove experts
Some experts in specific fields ( Such as hate speech ) It may not be necessary during testing , By removing these experts, in a sense, the model will not be exposed to these specific areas . Researchers replaced the full connection layer with a hybrid expert model , Such as BaseLayers and DeMix Layers, Carry out more effective training on a small and medium-sized scale . These simple methods eliminate fine-tuning .DEMix It has the ability to add additional modules , But it is not clear which method can better expand the scale of the model . How can we best use language models
Input... To the model 「What would you put meat on top of to cook it?」 Such a question about common sense ,GPT-3 Will answer :“hot dog bun”, But this is not the answer we expected , We hope the answer is similar to “A hot pan”. Although this example looks silly , But it can help us understand the problems encountered by the language model . When researchers adopt methods such as Commonsense QA When testing the model with common sense problems in the data set , The model may make the wrong choice . At this time, the model is making multiple choices ,“frying pan” Is one of the options , Although this option is correct , But it can be expressed in many ways . For the same thing , Language models can generate many different representations .
Zettlemoyer The professor showed that language models are most likely to address this problem 10 Outputs , It is found that some of them are completely wrong , But the other part of the answer is right or at least related to the right answer . The four blue options in the figure express the same concept , It's just a different expression of the pot . Notice that when there are multiple statements about the same thing , These same expressions compete with each other , Finally, it leads to formal competition (Surface Form Competition) problem . Because the language model is a probability distribution for words , When stove The probability of is 0.9 when ,frying pan The probability cannot exceed 0.1.
With COPA Take language modeling on data sets as an example . Assume that 「 The bar closed because 」, We need to start from the assumption 「 It's too crowded 」 and 「 It's three in the morning 」 Choose a more correct answer . If you directly input the premise into the model , Get the hypothesis 1 And assumptions 2 Probability , You will find assumptions 1 Higher probability , Because crowding and bars are often more relevant , But this is wrong . To prevent such mistakes , Some adjustments need to be made . For this purpose, conditional point mutual information (PMI), Choose a broader concept as the premise of the field , For example, choose “ because ” As the premise of the field (Domain Premise), Readjust the answer by calculating the conditional probability under the premise of the field .
Researchers tested models of different sizes on different data sets , The maximum number of model parameters reaches 175B. The experiment compares different adjustment schemes ,PMI In most cases, the program has achieved the best results .Zettlemoyer The professor introduced the learning scheme of noise channel language model . The noise channel model appeared in the pre deep learning era , Output the answer to a given question , The noise channel model calculates the probability of the problem based on the answer . When people fine tune the language model , Considering that the amount of calculation for fine tuning all parameters is too large , Often only part of the model is fine tuned . At present, there are many fine-tuning schemes , Such as prompt tuning,head tuning and transformation tuning. stay prompt tuning The channel model can be introduced . Experimental results show that , stay prompt tuning Under the scheme of adopting channel model , Compared with direct fine-tuning, the effect is significantly better ,head tuning Become the most competitive baseline , Tip tuning based on noise channel achieves better results on the whole , Especially in Yahoo and DBPedia On dataset .Zettlemoyer Professor pointed out :1) Although language models can calculate the probability of statements , But formal competition may lead to the wrong answer ;2)PMI By evaluating how much information the answer encodes , To adjust the probability of the answer ;3) Direct learning Noisy channel prompting model Even better results can be achieved . Other monitoring methods of pre training model Zettlemoyer The professor briefly reviewed the multilingual and multimodal pre training models , At the same time, it emphasizes the importance of open science . Today's models accept different supervisory signals , Recent work includes XGLM, This is a generative multilingual model ;HTLM, This is a model applied to document level formatted text ;CM3, Multimodal models ;InCoder Model for generating or populating code . Besides ,OPT It is the first public achievement GPT-3 Scale language model . stay HTLM In the model , Use document structure for pre training and cue models , For example, when performing summary tasks , It can be used <title> Label as a reminder . We can also get massive HTML Documentation for pre training .HTLM It can even automatically complete the prompt , For example, the model in the following figure is based on the text content , Automatically generated HTML Labels like <title> and <body>. stay CM3 In the model , The researchers will html Of documents <img> The image in the tag is discretized into a sequence , This forms a multimodal sequence, mixing text and images token, Then it can be treated as a general sequence using a language model , And carry out zero sample learning such as cross modal .InCoder It is a model that can generate missing code or comments , It trains on large-scale code datasets with open source licenses .Zettlemoyer Says Professor , The pre training model can receive a variety of monitoring signals , Images 、 Words, etc. can be discretized for pre training . Open science and model sharing are of great significance . Conclusion :
Last ,Zettlemoyer The professor returned to the three questions raised at the beginning of the speech :Zettlemoyer The professor said that at present, the scale of the model continues to grow, and researchers will still invest more computing power , In the future, the calculation of conditions may make a major breakthrough ; We still haven't found a way to make full use of language models , Zero learning and small sample learning are both worthy of research , However, it also depends on the performance pursued and the degree of open source of the code ;Zettlemoyer Professor pointed out , Text data is not required , Researchers can also try to use other structures or modes to provide monitoring signals .
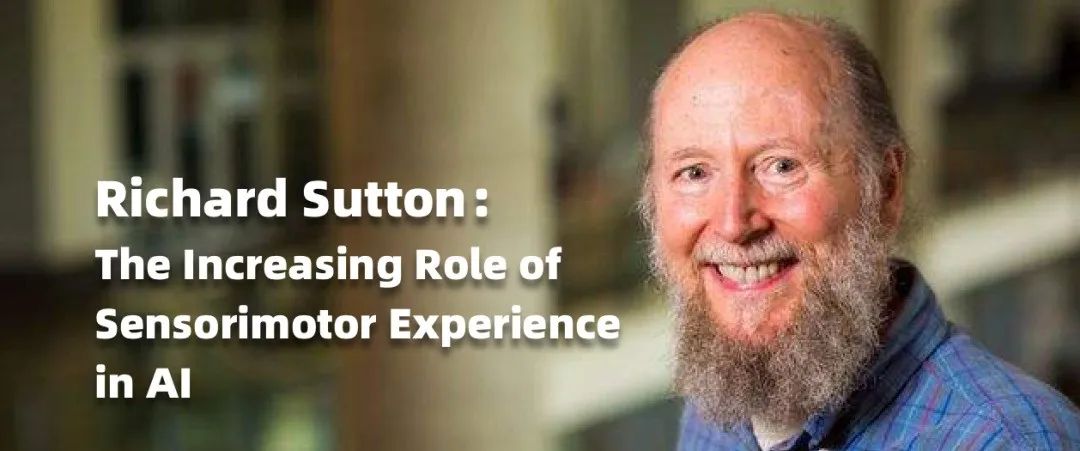
Richard Sutton: The experience is AI The ultimate data , Four stages lead to real AI The road to development Academician Mei Hong : How to construct artificial swarm intelligence ?| Review of the specially invited report of Zhiyuan Conference Turing award winner Adi Shamir The latest theory , Uncover the mystery of antagonistic samples | Review of the specially invited report of Zhiyuan Conference 原网站版权声明
本文为[Zhiyuan community]所创,转载请带上原文链接,感谢
https://yzsam.com/2022/187/202207060032470717.html