当前位置:网站首页>AI人脸编辑让Lena微笑
AI人脸编辑让Lena微笑
2022-07-06 23:42:00 【InfoQ】
1.进入AI人脸编辑页面案例页面,并完成基础配置
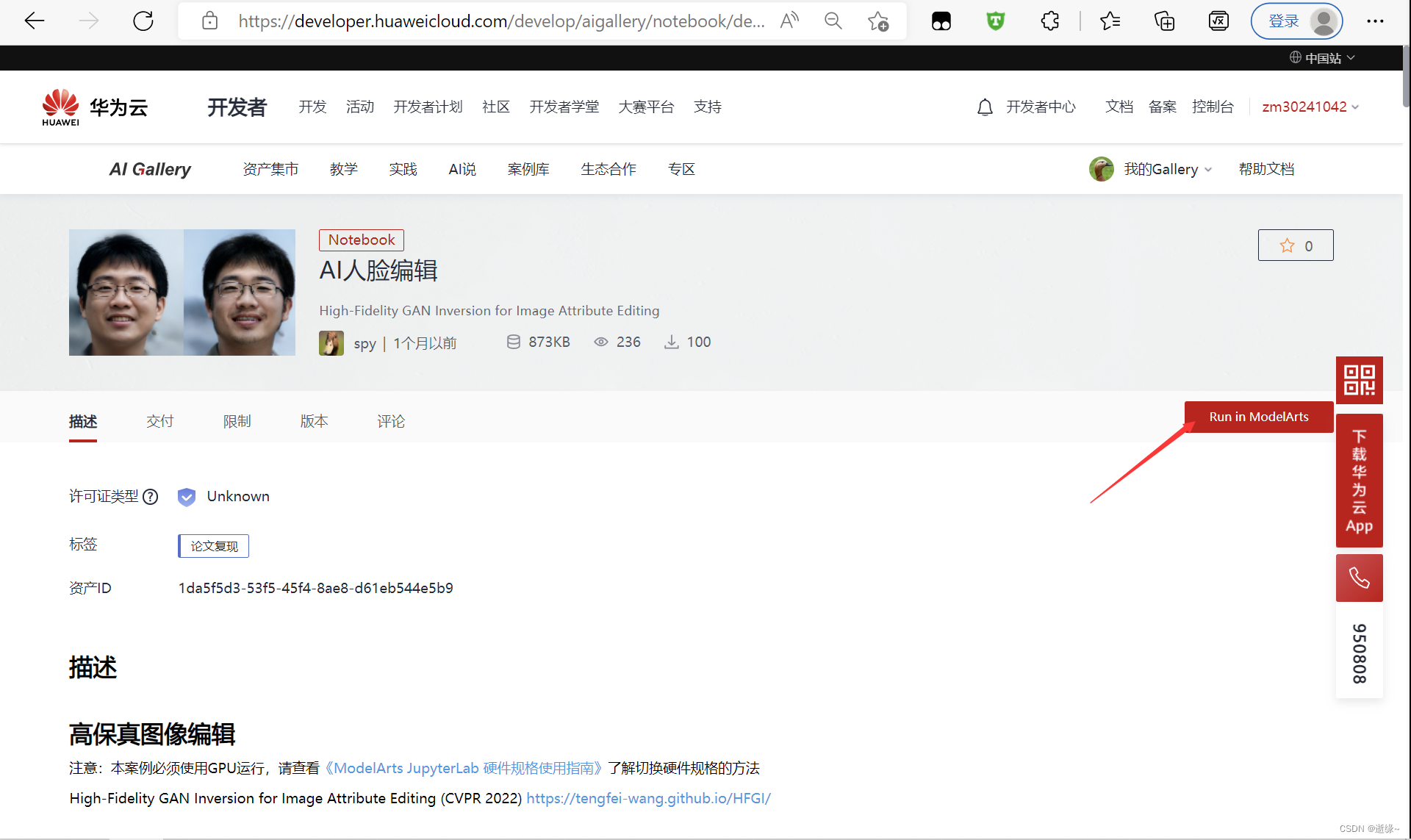
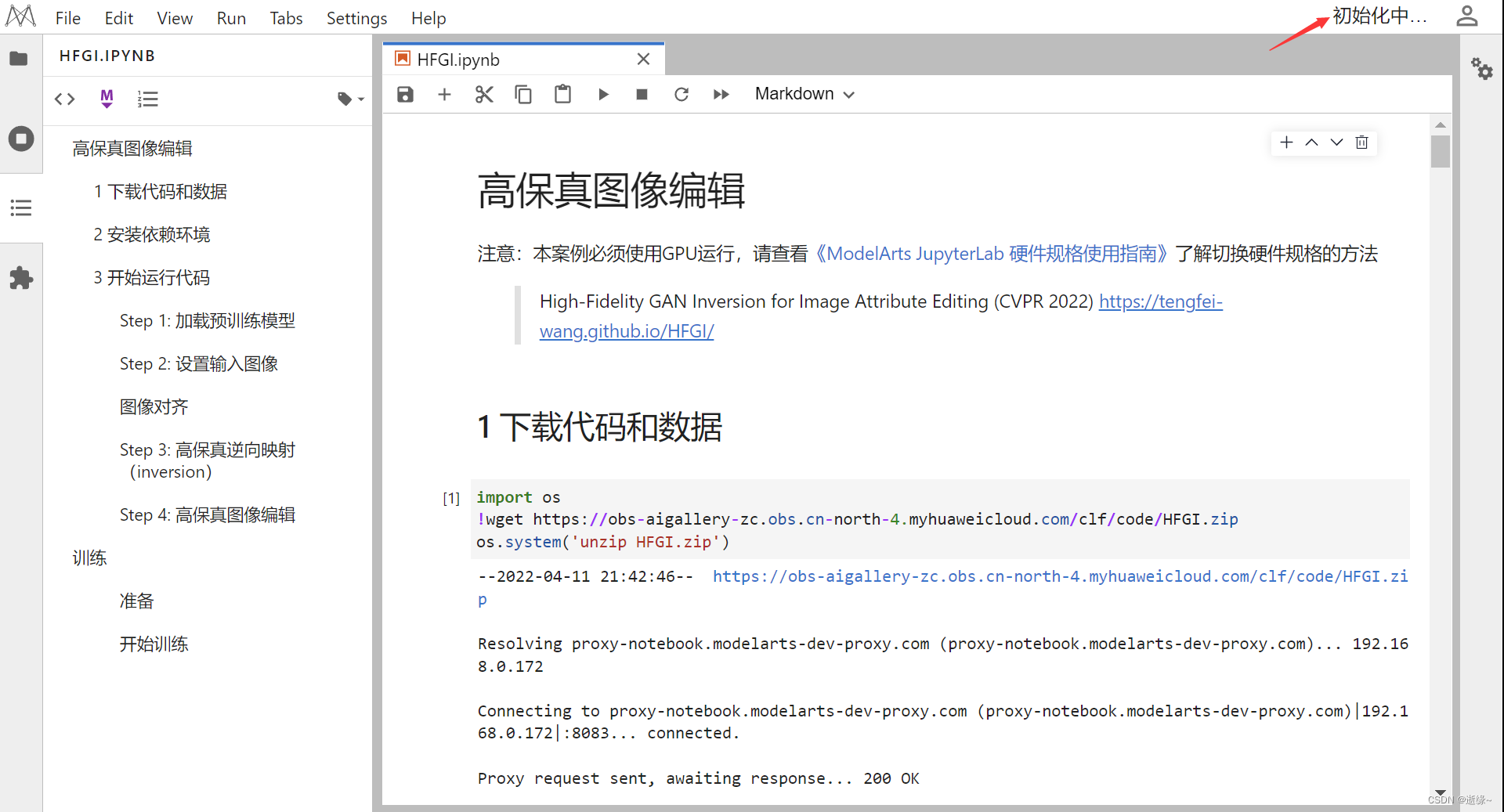
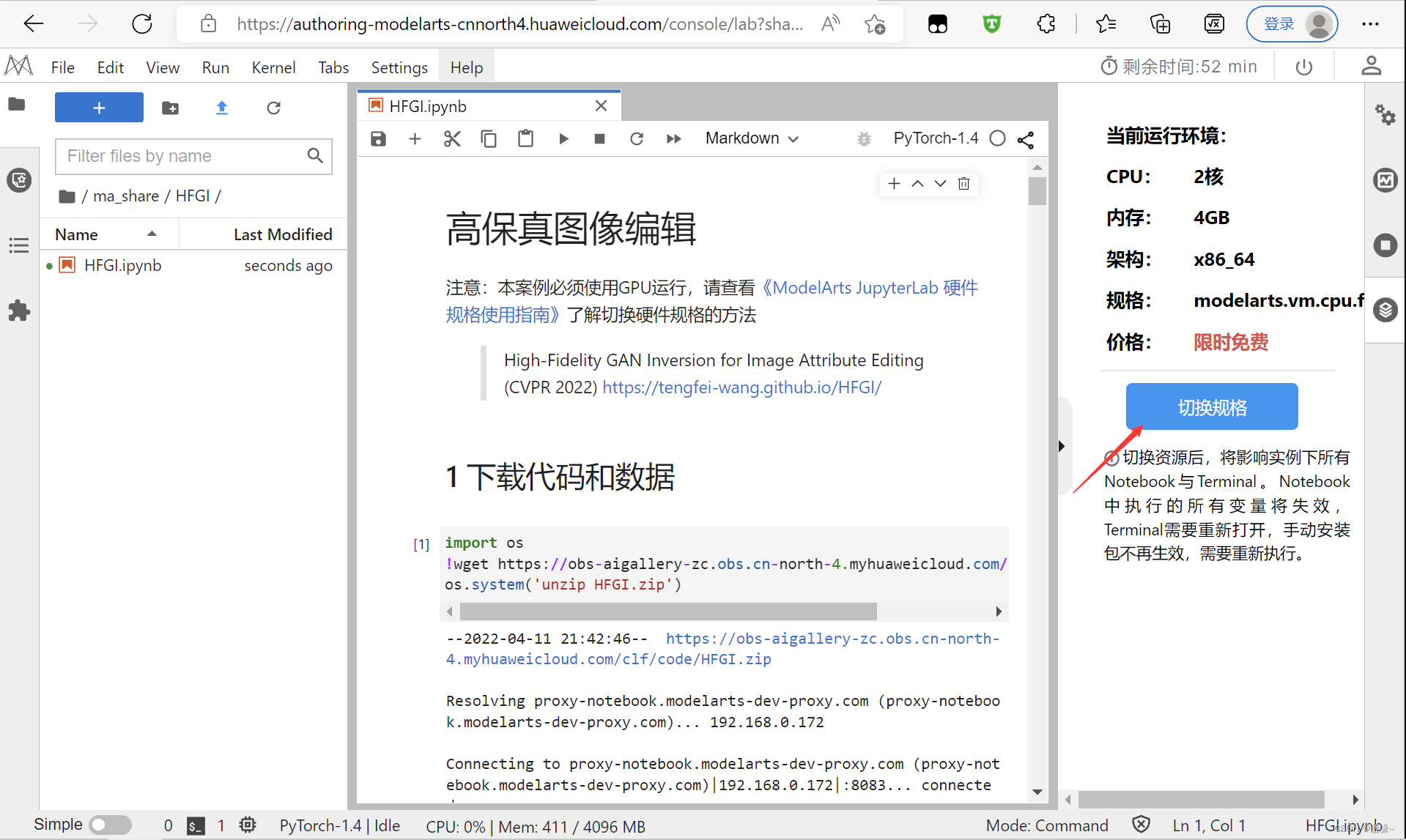

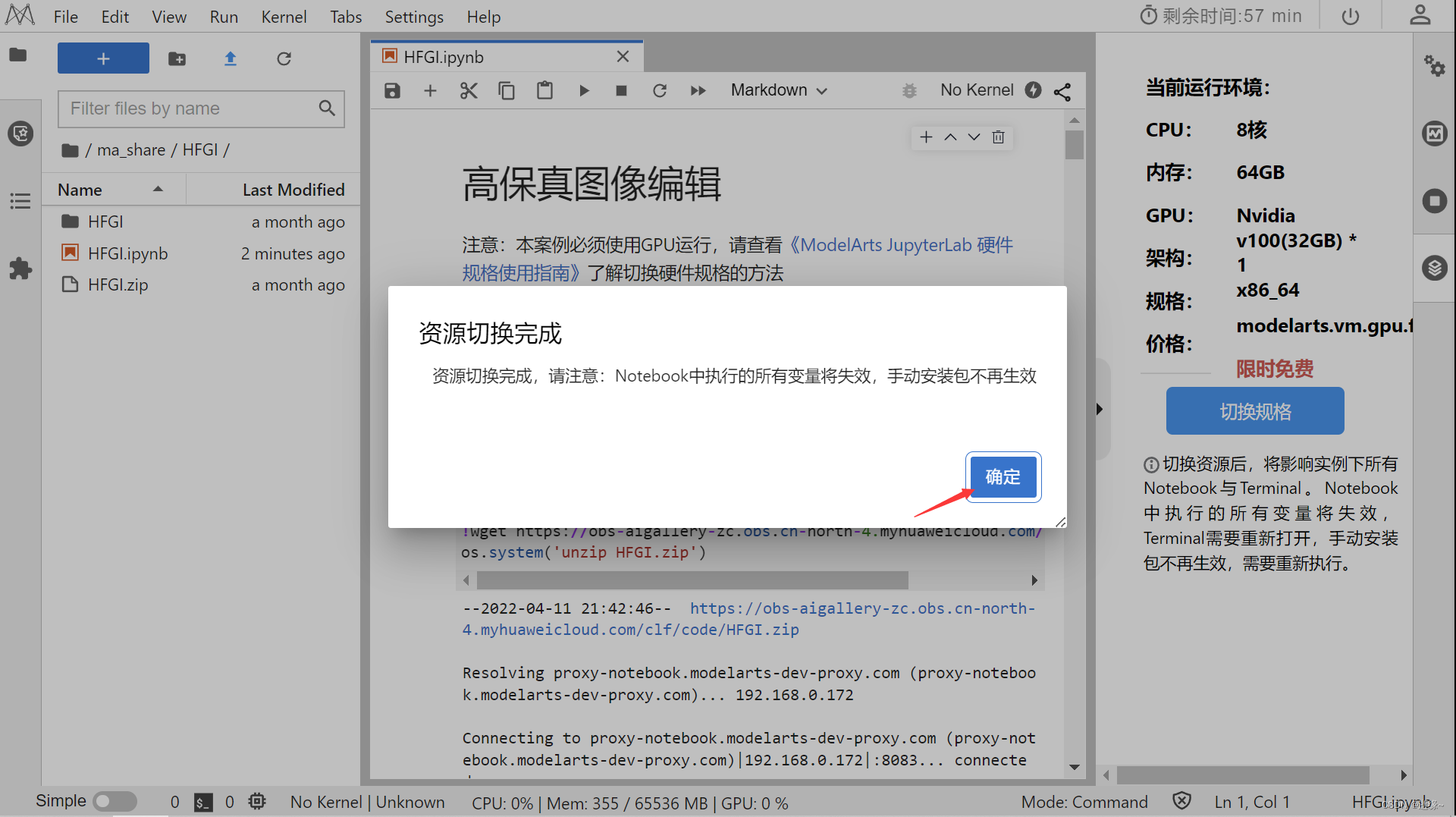
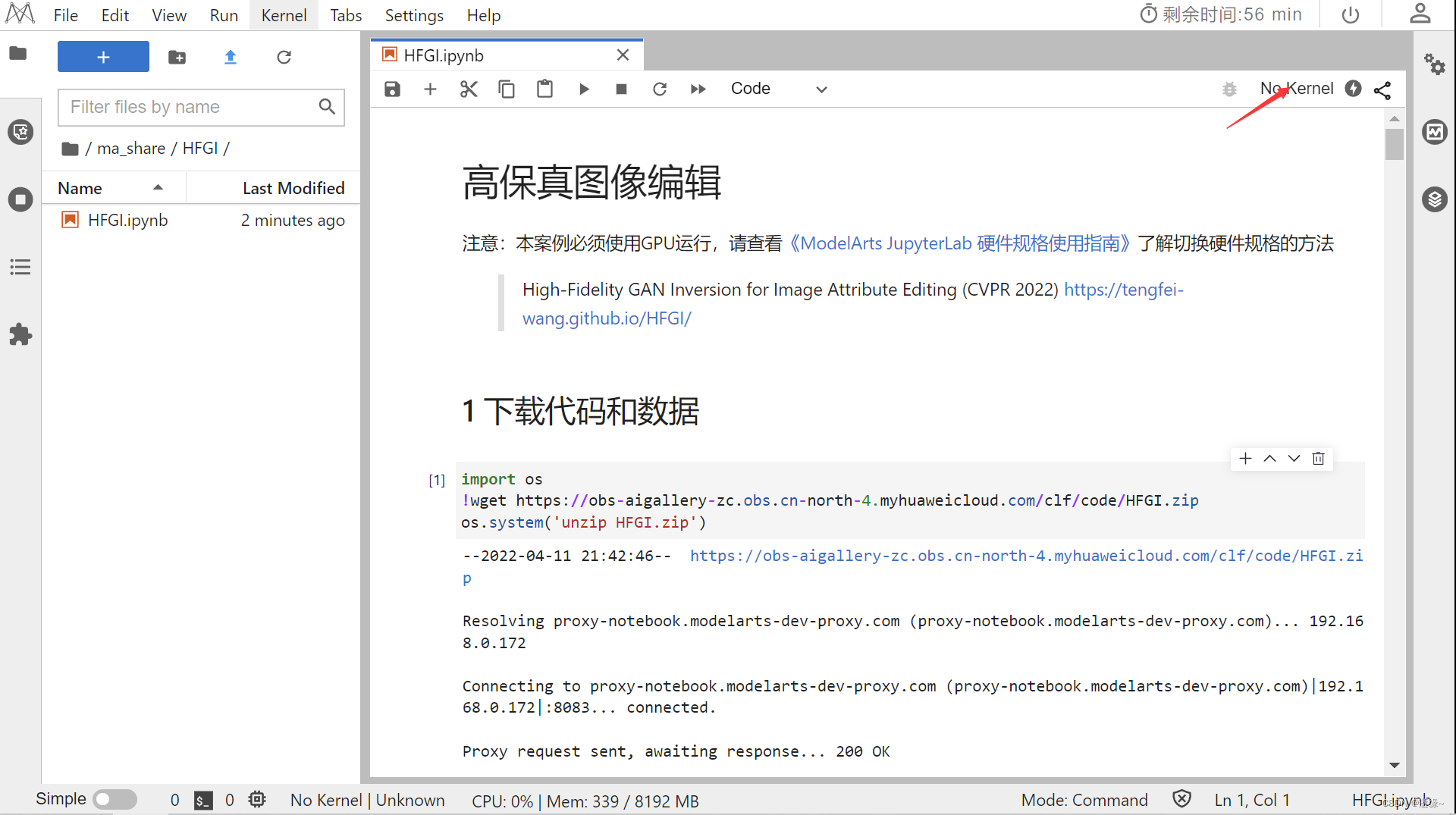
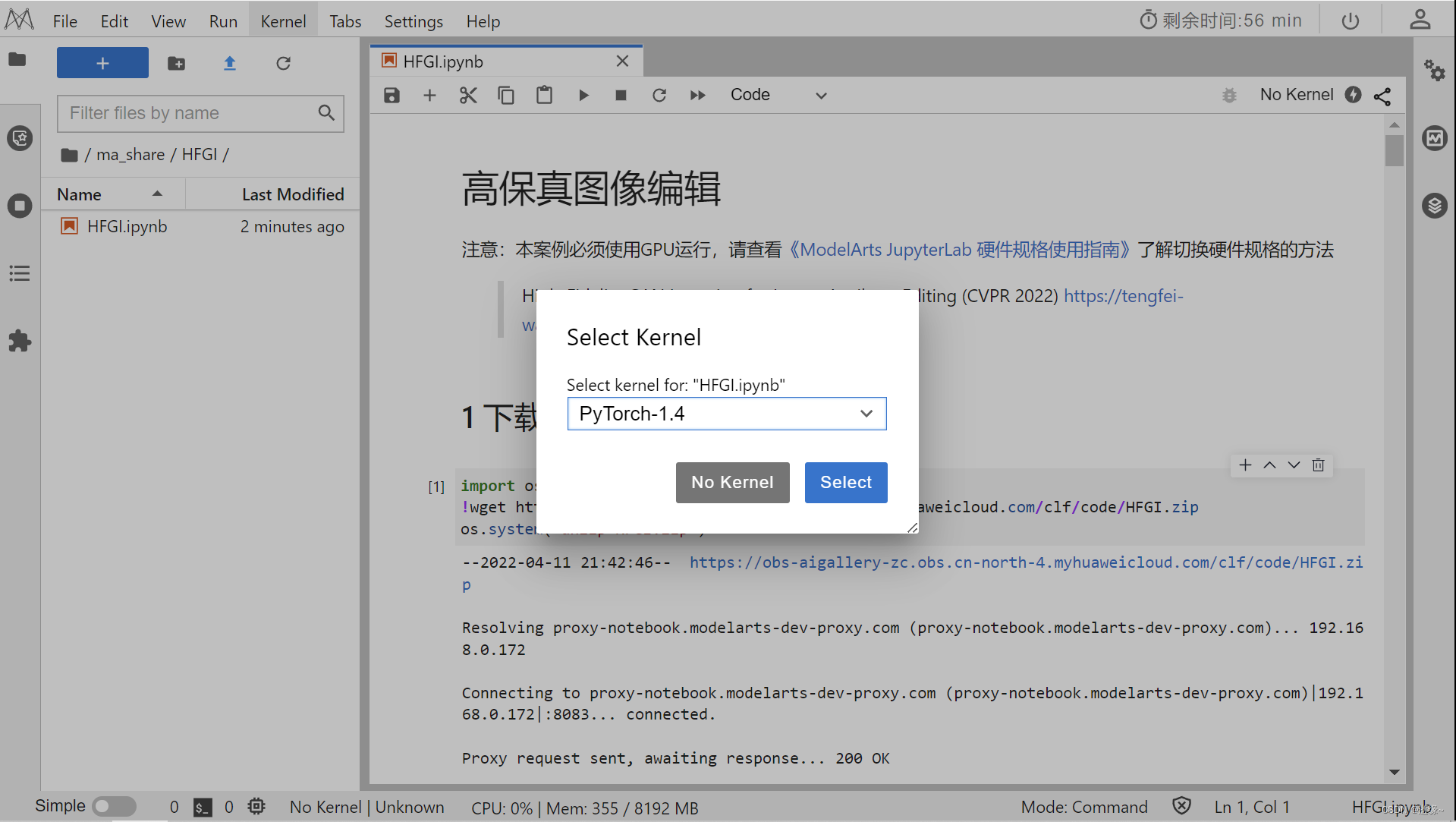
2.下载代码和数据并安装依赖

!pip install ninja
!pip install dlib
!pip uninstall -y torch
!pip uninstall -y torchvision
!pip install torch==1.6.0
!pip install torchvision==0.7.0
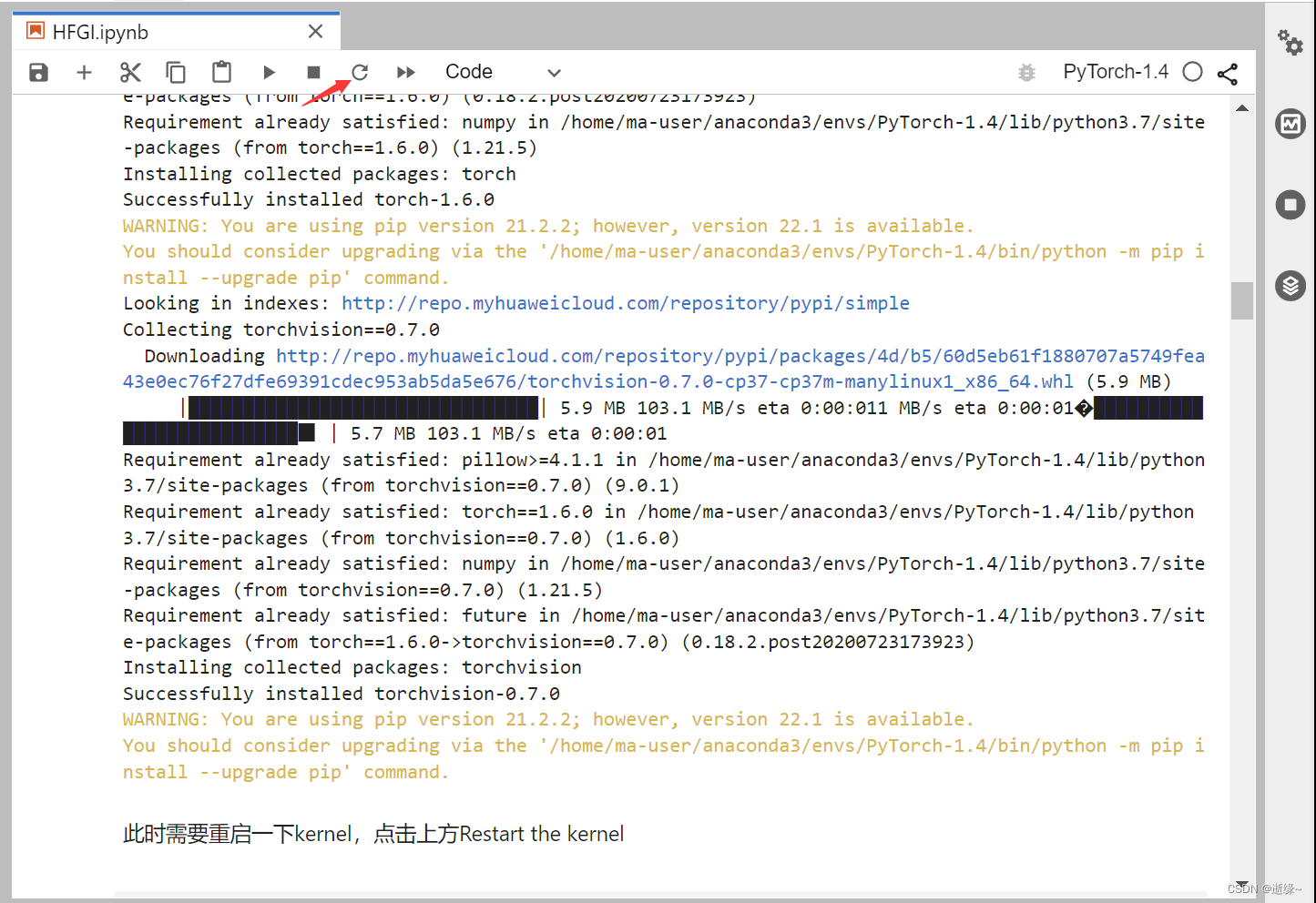
%cd HFGI
3.开始运行代码
#@title Setup Repository
import os
from argparse import Namespace
import time
import os
import sys
import numpy as np
from PIL import Image
import torch
import torchvision.transforms as transforms
# from utils.common import tensor2im
from models.psp import pSp # we use the pSp framework to load the e4e encoder.
%load_ext autoreload
%autoreload 2
def tensor2im(var):
# var shape: (3, H, W)
var = var.cpu().detach().transpose(0, 2).transpose(0, 1).numpy()
var = ((var + 1) / 2)
var[var < 0] = 0
var[var > 1] = 1
var = var * 255
return Image.fromarray(var.astype('uint8'))
model_path = "checkpoint/ckpt.pt"
ckpt = torch.load(model_path, map_location='cpu')
opts = ckpt['opts']
opts['is_train'] = False
opts['checkpoint_path'] = model_path
opts= Namespace(**opts)
net = pSp(opts)
net.eval()
net.cuda()
print('Model successfully loaded!')
# Setup required image transformations
EXPERIMENT_ARGS = {
"image_path": "test_imgs/Lina.jpg"
}
EXPERIMENT_ARGS['transform'] = transforms.Compose([
transforms.Resize((256, 256)),
transforms.ToTensor(),
transforms.Normalize([0.5, 0.5, 0.5], [0.5, 0.5, 0.5])])
resize_dims = (256, 256)
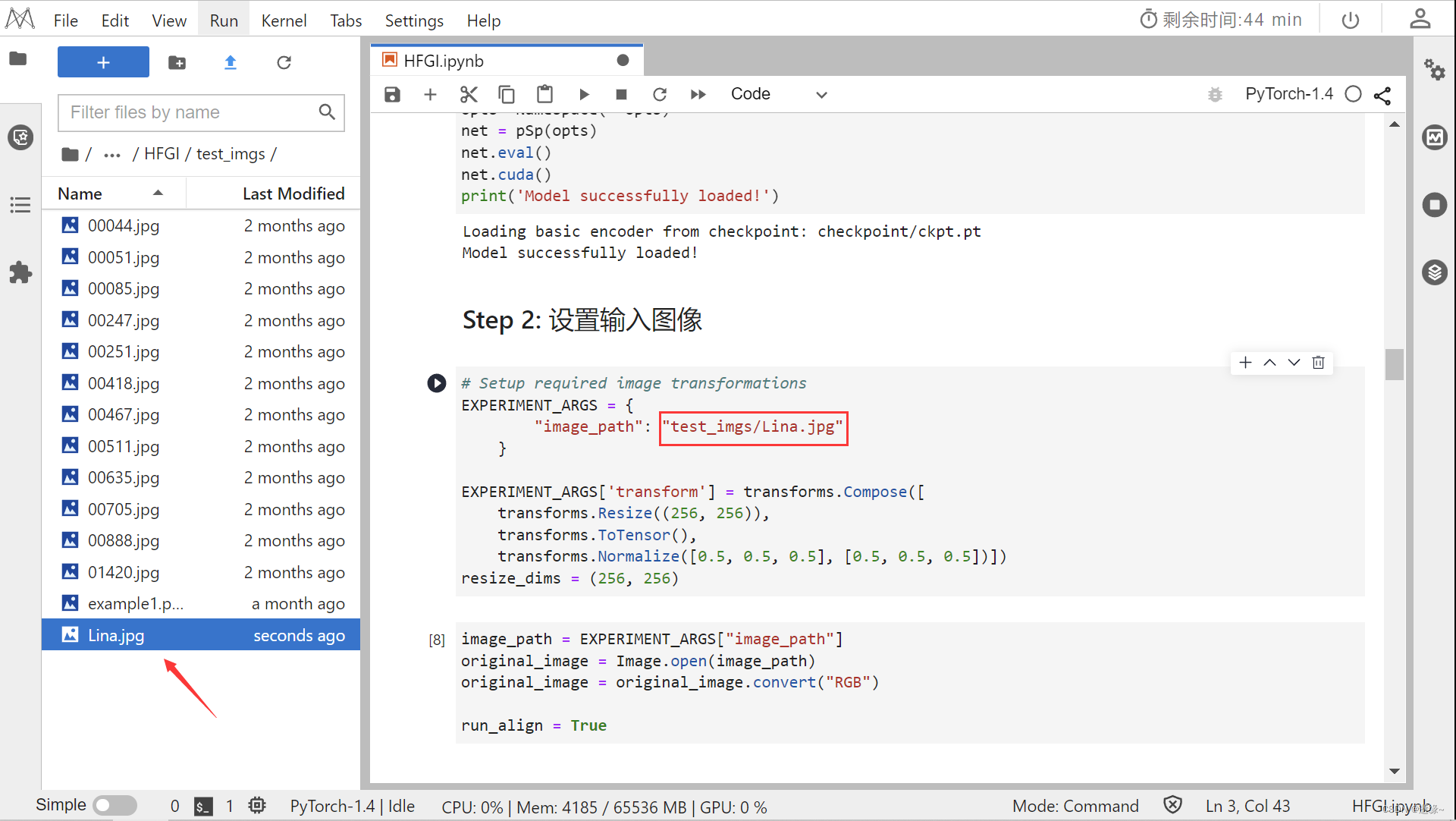
image_path = EXPERIMENT_ARGS["image_path"]
original_image = Image.open(image_path)
original_image = original_image.convert("RGB")
run_align = True
import numpy as np
import PIL
import PIL.Image
import scipy
import scipy.ndimage
import dlib
def get_landmark(filepath, predictor):
"""get landmark with dlib
:return: np.array shape=(68, 2)
"""
detector = dlib.get_frontal_face_detector()
img = dlib.load_rgb_image(filepath)
dets = detector(img, 1)
for k, d in enumerate(dets):
shape = predictor(img, d)
t = list(shape.parts())
a = []
for tt in t:
a.append([tt.x, tt.y])
lm = np.array(a)
return lm
def align_face(filepath, predictor):
"""
:param filepath: str
:return: PIL Image
"""
lm = get_landmark(filepath, predictor)
lm_chin = lm[0: 17] # left-right
lm_eyebrow_left = lm[17: 22] # left-right
lm_eyebrow_right = lm[22: 27] # left-right
lm_nose = lm[27: 31] # top-down
lm_nostrils = lm[31: 36] # top-down
lm_eye_left = lm[36: 42] # left-clockwise
lm_eye_right = lm[42: 48] # left-clockwise
lm_mouth_outer = lm[48: 60] # left-clockwise
lm_mouth_inner = lm[60: 68] # left-clockwise
# Calculate auxiliary vectors.
eye_left = np.mean(lm_eye_left, axis=0)
eye_right = np.mean(lm_eye_right, axis=0)
eye_avg = (eye_left + eye_right) * 0.5
eye_to_eye = eye_right - eye_left
mouth_left = lm_mouth_outer[0]
mouth_right = lm_mouth_outer[6]
mouth_avg = (mouth_left + mouth_right) * 0.5
eye_to_mouth = mouth_avg - eye_avg
# Choose oriented crop rectangle.
x = eye_to_eye - np.flipud(eye_to_mouth) * [-1, 1]
x /= np.hypot(*x)
x *= max(np.hypot(*eye_to_eye) * 2.0, np.hypot(*eye_to_mouth) * 1.8)
y = np.flipud(x) * [-1, 1]
c = eye_avg + eye_to_mouth * 0.1
quad = np.stack([c - x - y, c - x + y, c + x + y, c + x - y])
qsize = np.hypot(*x) * 2
# read image
img = PIL.Image.open(filepath)
output_size = 256
transform_size = 256
enable_padding = True
# Shrink.
shrink = int(np.floor(qsize / output_size * 0.5))
if shrink > 1:
rsize = (int(np.rint(float(img.size[0]) / shrink)), int(np.rint(float(img.size[1]) / shrink)))
img = img.resize(rsize, PIL.Image.ANTIALIAS)
quad /= shrink
qsize /= shrink
# Crop.
border = max(int(np.rint(qsize * 0.1)), 3)
crop = (int(np.floor(min(quad[:, 0]))), int(np.floor(min(quad[:, 1]))), int(np.ceil(max(quad[:, 0]))),
int(np.ceil(max(quad[:, 1]))))
crop = (max(crop[0] - border, 0), max(crop[1] - border, 0), min(crop[2] + border, img.size[0]),
min(crop[3] + border, img.size[1]))
if crop[2] - crop[0] < img.size[0] or crop[3] - crop[1] < img.size[1]:
img = img.crop(crop)
quad -= crop[0:2]
# Pad.
pad = (int(np.floor(min(quad[:, 0]))), int(np.floor(min(quad[:, 1]))), int(np.ceil(max(quad[:, 0]))),
int(np.ceil(max(quad[:, 1]))))
pad = (max(-pad[0] + border, 0), max(-pad[1] + border, 0), max(pad[2] - img.size[0] + border, 0),
max(pad[3] - img.size[1] + border, 0))
if enable_padding and max(pad) > border - 4:
pad = np.maximum(pad, int(np.rint(qsize * 0.3)))
img = np.pad(np.float32(img), ((pad[1], pad[3]), (pad[0], pad[2]), (0, 0)), 'reflect')
h, w, _ = img.shape
y, x, _ = np.ogrid[:h, :w, :1]
mask = np.maximum(1.0 - np.minimum(np.float32(x) / pad[0], np.float32(w - 1 - x) / pad[2]),
1.0 - np.minimum(np.float32(y) / pad[1], np.float32(h - 1 - y) / pad[3]))
blur = qsize * 0.02
img += (scipy.ndimage.gaussian_filter(img, [blur, blur, 0]) - img) * np.clip(mask * 3.0 + 1.0, 0.0, 1.0)
img += (np.median(img, axis=(0, 1)) - img) * np.clip(mask, 0.0, 1.0)
img = PIL.Image.fromarray(np.uint8(np.clip(np.rint(img), 0, 255)), 'RGB')
quad += pad[:2]
# Transform.
img = img.transform((transform_size, transform_size), PIL.Image.QUAD, (quad + 0.5).flatten(), PIL.Image.BILINEAR)
if output_size < transform_size:
img = img.resize((output_size, output_size), PIL.Image.ANTIALIAS)
# Return aligned image.
return img
if 'shape_predictor_68_face_landmarks.dat' not in os.listdir():
# !wget http://dlib.net/files/shape_predictor_68_face_landmarks.dat.bz2
!bzip2 -dk shape_predictor_68_face_landmarks.dat.bz2
def run_alignment(image_path):
import dlib
predictor = dlib.shape_predictor("shape_predictor_68_face_landmarks.dat")
aligned_image = align_face(filepath=image_path, predictor=predictor)
print("Aligned image has shape: {}".format(aligned_image.size))
return aligned_image
if run_align:
input_image = run_alignment(image_path)
else:
input_image = original_image
input_image.resize(resize_dims)
def display_alongside_source_image(result_image, source_image):
res = np.concatenate([np.array(source_image.resize(resize_dims)),
np.array(result_image.resize(resize_dims))], axis=1)
return Image.fromarray(res)
def get_latents(net, x, is_cars=False):
codes = net.encoder(x)
if net.opts.start_from_latent_avg:
if codes.ndim == 2:
codes = codes + net.latent_avg.repeat(codes.shape[0], 1, 1)[:, 0, :]
else:
codes = codes + net.latent_avg.repeat(codes.shape[0], 1, 1)
if codes.shape[1] == 18 and is_cars:
codes = codes[:, :16, :]
return codes
with torch.no_grad():
x = transformed_image.unsqueeze(0).cuda()
tic = time.time()
latent_codes = get_latents(net, x)
# calculate the distortion map
imgs, _ = net.decoder([latent_codes[0].unsqueeze(0).cuda()],None, input_is_latent=True, randomize_noise=False, return_latents=True)
res = x - torch.nn.functional.interpolate(torch.clamp(imgs, -1., 1.), size=(256,256) , mode='bilinear')
# ADA
img_edit = torch.nn.functional.interpolate(torch.clamp(imgs, -1., 1.), size=(256,256) , mode='bilinear')
res_align = net.grid_align(torch.cat((res, img_edit ), 1))
# consultation fusion
conditions = net.residue(res_align)
result_image, _ = net.decoder([latent_codes],conditions, input_is_latent=True, randomize_noise=False, return_latents=True)
toc = time.time()
print('Inference took {:.4f} seconds.'.format(toc - tic))
# Display inversion:
display_alongside_source_image(tensor2im(result_image[0]), input_image)
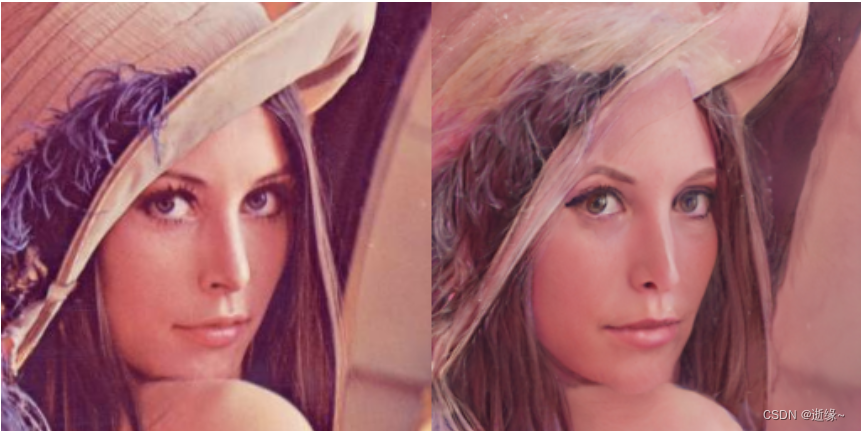
from editings import latent_editor
editor = latent_editor.LatentEditor(net.decoder)
# interface-GAN
interfacegan_directions = {
'age': './editings/interfacegan_directions/age.pt',
'smile': './editings/interfacegan_directions/smile.pt' }
edit_direction = torch.load(interfacegan_directions['smile']).cuda()
edit_degree = 1.5 # 设置微笑幅度
img_edit, edit_latents = editor.apply_interfacegan(latent_codes[0].unsqueeze(0).cuda(), edit_direction, factor=edit_degree) # 设置微笑
# align the distortion map
img_edit = torch.nn.functional.interpolate(torch.clamp(img_edit, -1., 1.), size=(256,256) , mode='bilinear')
res_align = net.grid_align(torch.cat((res, img_edit ), 1))
# fusion
conditions = net.residue(res_align)
result, _ = net.decoder([edit_latents],conditions, input_is_latent=True, randomize_noise=False, return_latents=True)
result = torch.nn.functional.interpolate(result, size=(256,256) , mode='bilinear')
display_alongside_source_image(tensor2im(result[0]), input_image)
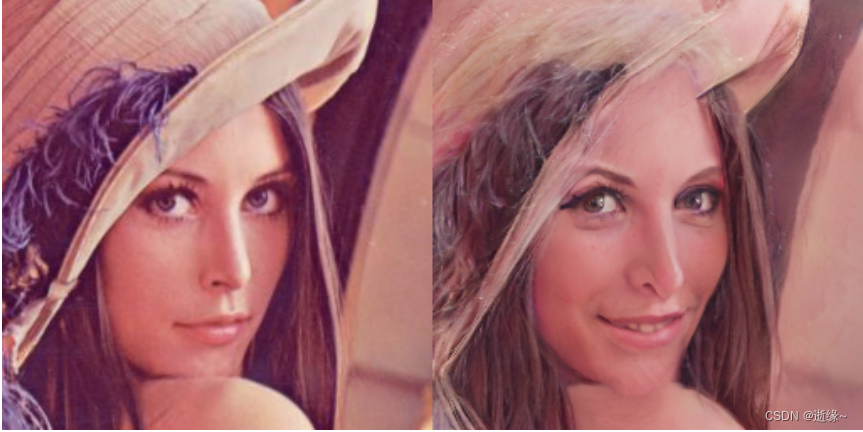
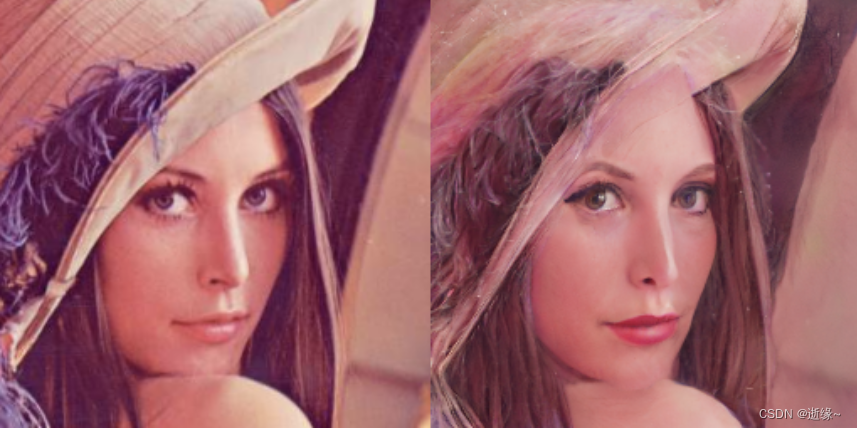
边栏推荐
- JVM (19) -- bytecode and class loading (4) -- talk about class loader again
- 利用OPNET进行网络仿真时网络层协议(以QoS为例)的使用、配置及注意点
- JVM(二十) -- 性能监控与调优(一) -- 概述
- 【oracle】简单的日期时间的格式化与排序问题
- 导航栏根据路由变换颜色
- Flink SQL 实现读写redis,并动态生成Hset key
- Design, configuration and points for attention of network unicast (one server, multiple clients) simulation using OPNET
- Jhok-zbl1 leakage relay
- Cve-2021-3156 vulnerability recurrence notes
- 阿里云的神龙架构是怎么工作的 | 科普图解
猜你喜欢
随机推荐
In memory, I moved from CSDN to blog park!
消息队列:重复消息如何处理?
MySQL数据库学习(8) -- mysql 内容补充
Batch size setting skills
Pinduoduo product details interface, pinduoduo product basic information, pinduoduo product attribute interface
Paper reading [open book video captioning with retrieve copy generate network]
K6el-100 leakage relay
Use, configuration and points for attention of network layer protocol (taking QoS as an example) when using OPNET for network simulation
分布式事务解决方案之2PC
Is the human body sensor easy to use? How to use it? Which do you buy between aqara green rice and Xiaomi
Flink SQL realizes reading and writing redis and dynamically generates hset key
Addressable pre Download
Let f (x) = Σ x^n/n^2, prove that f (x) + F (1-x) + lnxln (1-x) = Σ 1/n^2
实现网页内容可编辑
How digitalization affects workflow automation
做自媒体视频剪辑,专业的人会怎么寻找背景音乐素材?
How Alibaba cloud's DPCA architecture works | popular science diagram
MySQL数据库学习(7) -- pymysql简单介绍
基于 hugging face 预训练模型的实体识别智能标注方案:生成doccano要求json格式
Jhok-zbl1 leakage relay