当前位置:网站首页>tensorflow之tf.tile\tf.slice等函数的基本用法解读
tensorflow之tf.tile\tf.slice等函数的基本用法解读
2020-11-06 01:27:00 【IT界的小小小学生】
tf.tile
解读:
tensorflow中的tile()函数是用来对张量(Tensor)进行扩展的,其特点是对当前张量内的数据进行一定规则的复制。最终的输出张量维度不变。
函数定义:
tf.tile(
input,
multiples,
name=None)
input是待扩展的张量,multiples是扩展方法。
假如input是一个3维的张量。那么mutiples就必须是一个1x3的1维张量。这个张量的三个值依次表示input的第1,第2,第3维数据扩展几倍。
具体举一个例子:
import tensorflow as tf
a = tf.constant([[1, 2], [3, 4], [5, 6]], dtype=tf.float32)
a1 = tf.tile(a, [2, 3])
with tf.Session() as sess:
print(sess.run(a))
print(sess.run(a1))
tf.tile()具体的操作过程如下:
请注意:上面绘图中第一次扩展后第一维由三个数据变成两行六个数据,多一行并不是多了一维,数据扔为顺序排列,只是为了方便绘制而已。
每一维数据的扩展都是将前面的数据进行复制然后直接接在原数据后面。
如果multiples的某一个数据为1,则表示该维数据保持不变。
示例:
import tensorflow as tf
def test12():
a = tf.constant([[1, 2], [3, 4], [5, 6]], dtype=tf.float32)
print(a)
doc_y = tf.tile(a, [1, 1])
print(doc_y)
with tf.Session() as sess:
print(sess.run(a))
print(sess.run(doc_y))
[[ 1. 2.]
[ 3. 4.]
[ 5. 6.]]
[[ 1. 2.]
[ 3. 4.]
[ 5. 6.]]
tf.slice
tf.slice(input_, begin, size, name = None)
解释 :
这个函数的作用是从输入数据input中提取出一块切片
切片的尺寸是size,切片的开始位置是begin。
切片的尺寸size表示输出tensor的数据维度,其中size[i]表示在第i维度上面的元素个数。
开始位置begin表示切片相对于输入数据input_的每一个偏移量,比如数据input是
[[[1, 1, 1], [2, 2, 2]],
[[33, 3, 3], [4, 4, 4]],
[[5, 5, 5], [6, 6, 6]]],
begin为[1, 0, 0],那么数据的开始位置是33。因为,第一维偏移了1,其余几位都没有偏移,所以开始位置是33。
操作满足:
size[i] = input.dim_size(i) - begin[i]
0 <= begin[i] <= begin[i] + size[i] <= Di for i in [0, n]
这里解释一下tf.slice()的作用和用法;
silce_1,此时切片的起点是[0,0,0],切片的大小是[1,1,3];于是从原点开始切一个[1,1,3]的数据,也就是一个批次的(1,3)
slice_2,此时切片的起点是[1,0,0],切片的大小是[1,2,3];意思就是从第二个批次的数据开始进行切片,切下一个批次的(2,3)的数据
slice_3,此时切片的起点仍然是[1,0,0],切片的大小是[2,1,3];就是从第二个批次开始,切一个两个批次的(1,3)的数据
示例:
import tensorflow as tf
sess = tf.Session()
input = tf.constant([[[1, 1, 1], [2, 2, 2]],
[[3, 3, 3], [4, 4, 4]],
[[5, 5, 5], [6, 6, 6]]])
data = tf.slice(input, [1, 0, 0], [1, 1, 3])
print(sess.run(data))
"""[1,0,0]表示第一维偏移了1
则是从[[[3, 3, 3], [4, 4, 4]],[[5, 5, 5], [6, 6, 6]]]中选取数据
然后选取第一维的第一个,第二维的第一个数据,第三维的三个数据"""
# [[[3 3 3]]]
data = tf.slice(input, [1, 0, 0], [1, 2, 3])
print(sess.run(data))
# [[[3 3 3]
# [4 4 4]]]
data = tf.slice(input, [1, 0, 0], [2, 1, 3])
print(sess.run(data))
# [[[3 3 3]]
#
# [[5 5 5]]]
data = tf.slice(input, [1, 0, 0], [2, 2, 2])
print(sess.run(data))
# [[[3 3]
# [4 4]]
#
# [[5 5]
# [6 6]]]
"""输入参数:
● input_: 一个Tensor。
● begin: 一个Tensor,数据类型是int32或者int64。
● size: 一个Tensor,数据类型是int32或者int64。
● name:(可选)为这个操作取一个名字。
输出参数:
● 一个Tensor,数据类型和input_相同。"""
示例二:多应用与求概率
prob = tf.constant([[1, 2], [3, 4], [5, 6]], dtype=tf.float32)
hit_prob = tf.slice(prob, [0, 0], [-1, 1])
sess = tf.Session()
print(sess.run(hit_prob))
[[ 1.]
[ 3.]
[ 5.]]
tf.square()
tf.math.square(
x,
name=None
)
功能:计算元素x的平方
Args:
x: A Tensor or SparseTensor. Must be one of the following types: half, float32, float64, int32, int64, complex64, complex128.
name: A name for the operation (optional).
prob = tf.constant([[1, 2], [3, 4], [5, 6]], dtype=tf.float32)
sess = tf.Session()
print(sess.run(tf.square(prob)))
[[ 1. 4.]
[ 9. 16.]
[ 25. 36.]]
tf.reduce_sum
reduce_sum(input_tensor, axis=None, keepdims=False, name=None)
For example:
x = tf.constant([[1, 1, 1], [1, 1, 1]])
tf.reduce_sum(x) # 6
tf.reduce_sum(x, 0) # [2, 2, 2]
tf.reduce_sum(x, 1) # [3, 3]
tf.reduce_sum(x, 1, keepdims=True) # [[3], [3]]
tf.reduce_sum(x, [0, 1]) # 6
Args:
input_tensor: The tensor to reduce. Should have numeric type.
axis: The dimensions to reduce. If None
(the default), reduces all
dimensions. Must be in the range [-rank(input_tensor), rank(input_tensor))
.
keepdims: If true, retains reduced dimensions with length 1.
name: A name for the operation (optional).
Returns:
The reduced tensor, of the same dtype as the input_tensor.
tf.multiply()
两个矩阵中对应元素各自相乘
格式: tf.multiply(x, y, name=None)
参数:
x: 一个类型为:half, float32, float64, uint8, int8, uint16, int16, int32, int64, complex64, complex128的张量。
y: 一个类型跟张量x相同的张量。
返回值: x * y element-wise.
注意:
(1)multiply这个函数实现的是元素级别的相乘,也就是两个相乘的数元素各自相乘,而不是矩阵乘法,注意和tf.matmul区别。
(2)两个相乘的数必须有相同的数据类型,不然就会报错。
tf.matmul()
将矩阵a乘以矩阵b,生成a * b。
格式: tf.matmul(a, b, transpose_a=False, transpose_b=False, adjoint_a=False, adjoint_b=False, a_is_sparse=False, b_is_sparse=False, name=None)
参数:
a: 一个类型为 float16, float32, float64, int32, complex64, complex128 且张量秩 > 1 的张量。
b: 一个类型跟张量a相同的张量。
transpose_a: 如果为真, a则在进行乘法计算前进行转置。
transpose_b: 如果为真, b则在进行乘法计算前进行转置。
adjoint_a: 如果为真, a则在进行乘法计算前进行共轭和转置。
adjoint_b: 如果为真, b则在进行乘法计算前进行共轭和转置。
a_is_sparse: 如果为真, a会被处理为稀疏矩阵。
b_is_sparse: 如果为真, b会被处理为稀疏矩阵。
name: 操作的名字(可选参数)
返回值: 一个跟张量a和张量b类型一样的张量且最内部矩阵是a和b中的相应矩阵的乘积。
注意:
(1)输入必须是矩阵(或者是张量秩 >2的张量,表示成批的矩阵),并且其在转置之后有相匹配的矩阵尺寸。
(2)两个矩阵必须都是同样的类型,支持的类型如下:float16, float32, float64, int32, complex64, complex128。
引发错误:
ValueError: 如果transpose_a 和 adjoint_a, 或 transpose_b 和 adjoint_b 都被设置为真
tf.truediv
tf.truediv(
x,
y,
name=None
)
参数说明
x:张量。数值类型,作为分子。
y: 张量。数值类型,作为分母。
name:操作的名字,可选。
返回值:x/y,浮点型。
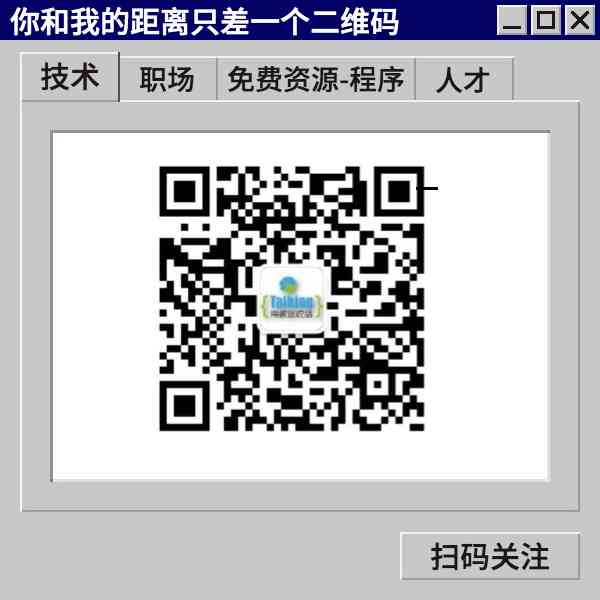
版权声明
本文为[IT界的小小小学生]所创,转载请带上原文链接,感谢
https://vip01.blog.csdn.net/article/details/93755743
边栏推荐
猜你喜欢
随机推荐
How do the general bottom buried points do?
至联云分享:IPFS/Filecoin值不值得投资?
PLC模拟量输入和数字量输入是什么
Jmeter——ForEach Controller&Loop Controller
微服務 - 如何解決鏈路追蹤問題
DTU连接经常遇到的问题有哪些
WeihanLi.Npoi 1.11.0/1.12.0 Release Notes
基于深度学习的推荐系统
Don't go! Here is a note: picture and text to explain AQS, let's have a look at the source code of AQS (long text)
如何对Pandas DataFrame进行自定义排序
Kitty中的动态线程池支持Nacos,Apollo多配置中心了
3分钟读懂Wi-Fi 6于Wi-Fi 5的优势
ThreadLocal原理大解析
mac 下常用快捷键,mac启动ftp
Flink on paasta: yelp's new stream processing platform running on kubernetes
Troubleshooting and summary of JVM Metaspace memory overflow
50 + open source projects are officially assembled, and millions of developers are voting
速看!互联网、电商离线大数据分析最佳实践!(附网盘链接)
Aprelu: cross border application, adaptive relu | IEEE tie 2020 for machine fault detection
Ubuntu18.04上安裝NS-3