当前位置:网站首页>Function of activation function
Function of activation function
2022-07-06 06:00:00 【algolearn】
The activation function can introduce non-linear factors into the linear model , So as to solve the problem that linear model is difficult to solve .
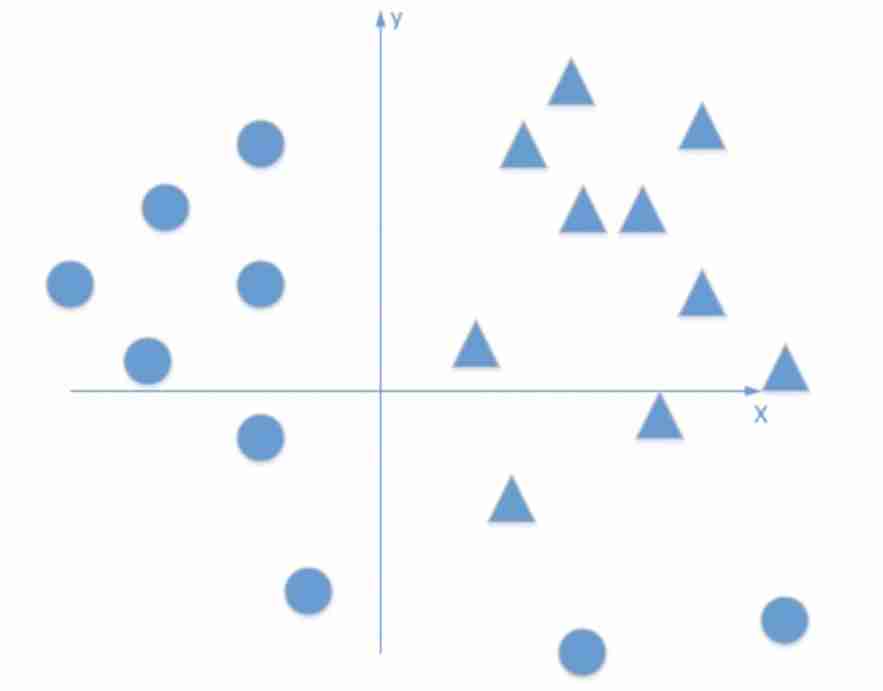
Design a classification to separate the triangle and circle above , Take perceptron as an example , Consider a few situations :
Single layer perceptron Multilayer perceptron Single layer perceptron + Activation function Multilayer perceptron + Activation function
1 Single layer perceptron
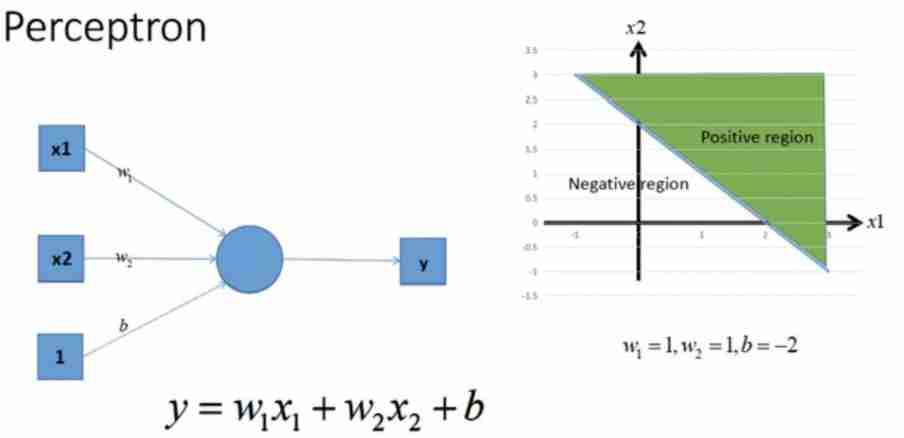
The expression of single-layer perceptron is , It can draw a line , Divide the plane . For input features And characteristics , If , Prove to be a positive class ; If , Prove to be a negative class . We won't discuss it here In special circumstances . According to this, we can draw the coordinate map on the right .
No matter how the straight line obtained by the perceptron moves, it cannot separate the triangle from the circle .
2 Multilayer perceptron

The expression of multi-layer perceptron is shown in the formula on the right of the above figure , After the expression merges similar items , You can get , It can be found that no matter how it is combined , The final result is about the input linear equation, which can't deal with non-linear classification .
The straight line obtained by the multi-layer perceptron cannot separate the triangle from the circle no matter how it moves .
3 Single layer perceptron + Activation function
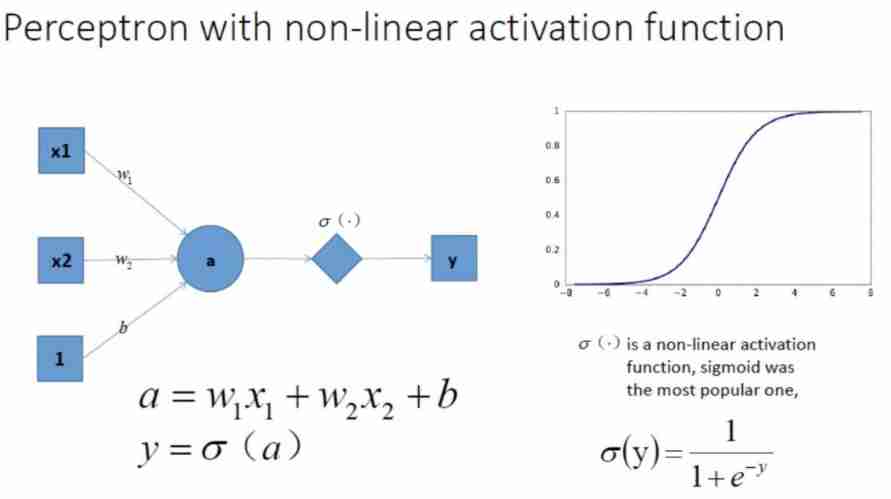
Add another one to the output of the sensor sigmoid Activation function , because sigmoid Is a non-linear function , So the output is obviously a nonlinear function , It is possible to solve the non-linear classification problem mentioned above .
Single layer perceptron + The curve obtained by activating the function may separate the triangle from the circle .
4 Multilayer perceptron + Activation function
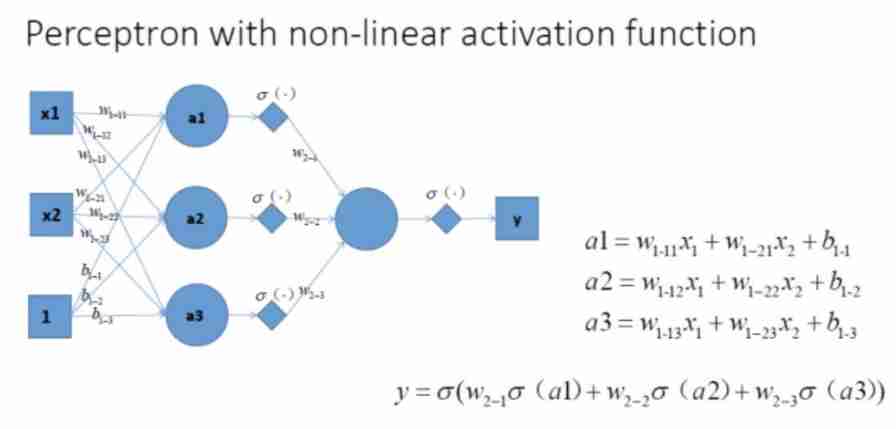
Add the activation function to the output of the multi-layer perceptron , You can get the expression on the right . Because each layer is a non-linear output , The final output will also be a non-linear function , It is also possible for the non-linear classification problem mentioned above .
Multilayer perceptron + The curve obtained by activating the function may separate the triangle from the circle .
3、4 By constantly optimizing the loss function , Can learn to correctly classify the curves of triangles and circular points , As shown in the figure below 3 Curves .
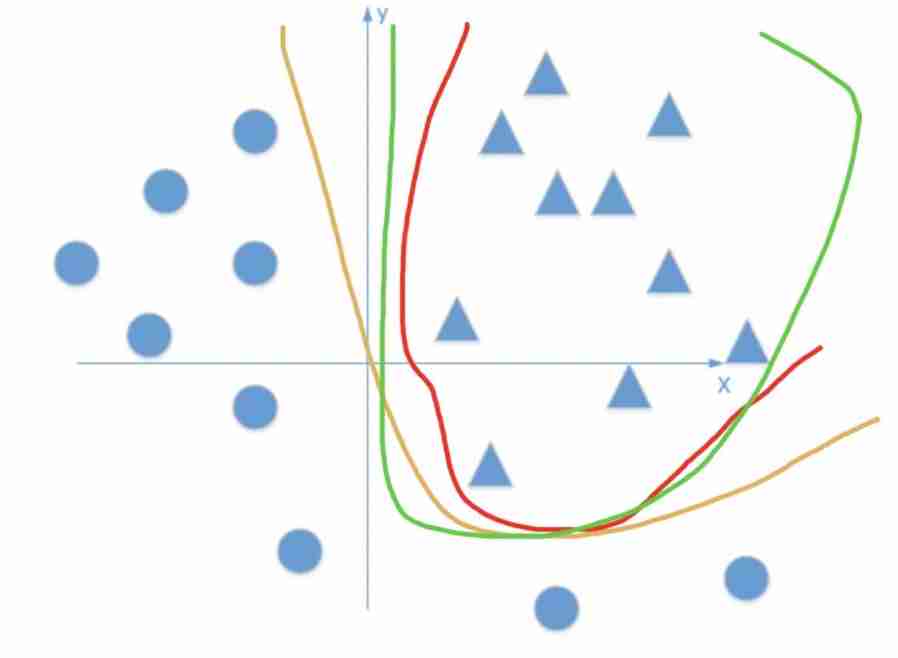
边栏推荐
- Novice entry SCM must understand those things
- [happy Spring Festival] if you feel happy, dance
- Embedded interview questions (I: process and thread)
- 【论文代码】SML部分代码阅读
- 【SQL server速成之路】——身份驗證及建立和管理用戶賬戶
- 清除浮动的方式
- Dynamic programming -- knapsack problem
- Raised a kitten
- Is it difficult for an information system project manager?
- [email protected]树莓派
猜你喜欢
关于 PHP 启动 MongoDb 找不到指定模块问题
Sqlmap tutorial (III) practical skills II
【论文代码】SML部分代码阅读
B站刘二大人-Softmx分类器及MNIST实现-Lecture 9
continue和break的区别与用法
Construction of yolox based on paste framework
初识数据库
Clock in during winter vacation
LAN communication process in the same network segment
H3C V7 switch configuration IRF
随机推荐
Analysis report on development trends and investment planning of China's methanol industry from 2022 to 2028
Sqlmap tutorial (III) practical skills II
[SQL Server Express Way] - authentification et création et gestion de comptes utilisateurs
Embedded interview questions (I: process and thread)
OSPF configuration command of Huawei equipment
Is it difficult for an information system project manager?
Station B, Mr. Liu Er - multiple logistic regression, structure 7
Analysis of grammar elements in turtle Library
Rustdesk builds its own remote desktop relay server
通讯录管理系统链表实现
Clock in during winter vacation
【SQL server速成之路】——身份驗證及建立和管理用戶賬戶
Request forwarding and redirection
LAN communication process in the same network segment
Li Chuang EDA learning notes 12: common PCB board layout constraint principles
Company video accelerated playback
nodejs实现微博第三方登录
[email protected]树莓派
Migrate Infones to stm32
The ECU of 21 Audi q5l 45tfsi brushes is upgraded to master special adjustment, and the horsepower is safely and stably increased to 305 horsepower