当前位置:网站首页>Wu Enda's refining notes on machine learning 4: basis of neural network - Zhihu
Wu Enda's refining notes on machine learning 4: basis of neural network - Zhihu
2020-11-10 07:36:00 【osc_lhwd57ou】
author :Peter
Red stone's personal website :
Red stone's personal blog - machine learning 、 The road to deep learning www.redstonewill.com
Today, I'll bring you the notes for the fourth week : Neural network basis .
- Nonlinear hypothesis
- Neurons and the brain
- Model to represent
- Features and intuitive understanding
- Multiple classification problems
Nonlinear hypothesis Non-linear Hypotheses
The disadvantages of linear regression and logical regression : When there are too many features , The computational load will be very large
Suppose we want to train a model to recognize visual objects ( For example, identify whether a car is on a picture ), How can we do this ? One way is to use a lot of pictures of cars and a lot of pictures of non cars , And then use the values of the pixels on these images ( Saturation or brightness ) As a feature .
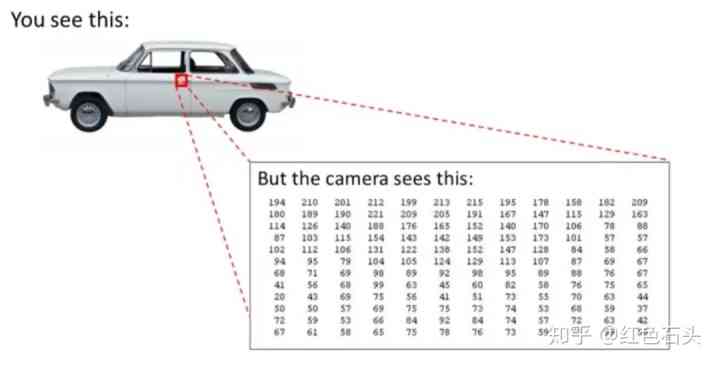
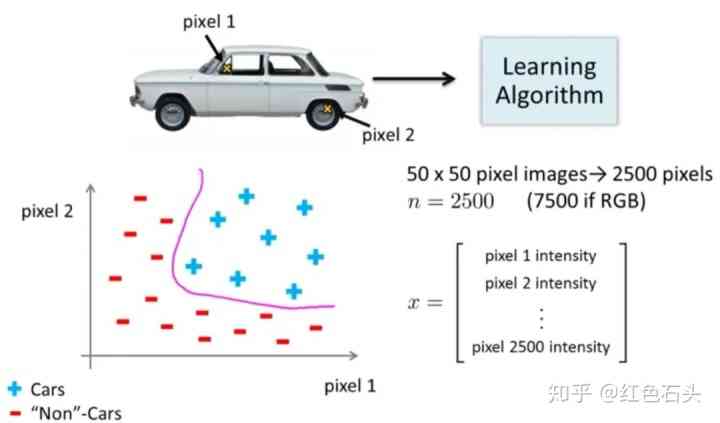
Suppose you use 50*50 A small picture of pixels , Take all pixels as features , Then there are 2500 Features . What ordinary logistic regression models can't handle , You need to use neural networks
Neurons and the brain
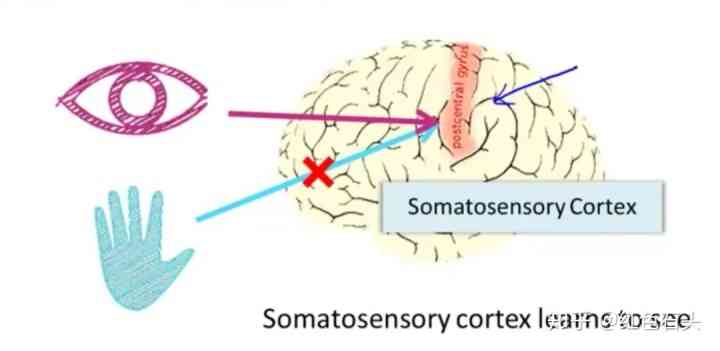
Model to represent
Model to represent 1
Each neuron can be thought of as a processing unit / Nucleus nervi processing unit/Nucleus, It mainly includes :
- Multiple inputs / Dendrites input/Dendrite
- An output / axon output/Axon
A neural network is a network in which a large number of neurons are interconnected and communicate through electrical pulses
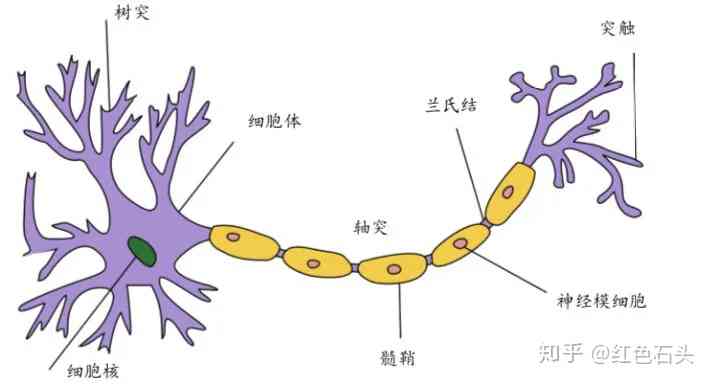
- The neural network model is based on many neurons , Each neuron is a learning model
- Neurons are called activation units activation unit; In the neural network , Parameters can also be called weights (weight)
- Neural networks like neurons
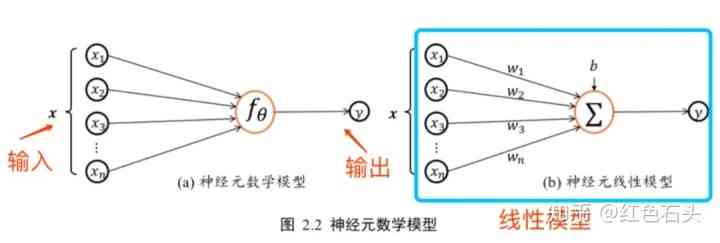
neural network
The following is an example of a logistic regression model as a neuron of its own learning model
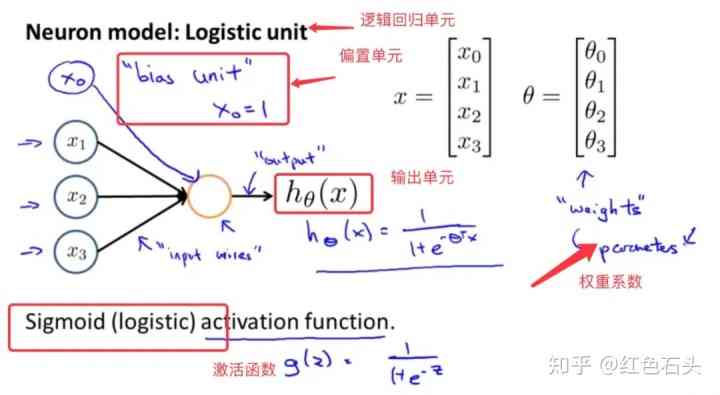
A neural network structure similar to neurons
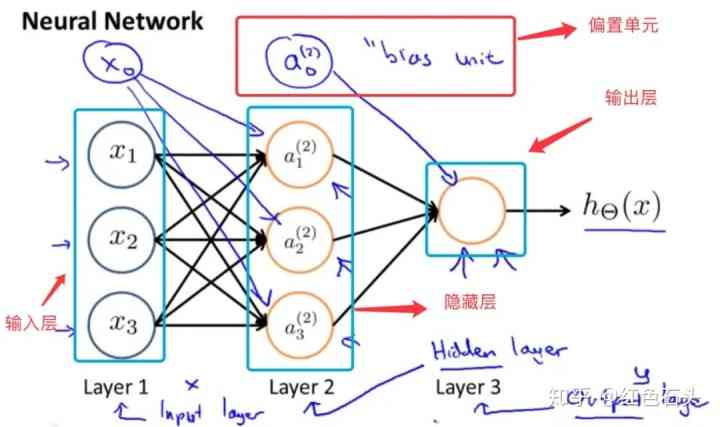
- x1,x2,x3 It's the input unit , Input raw data into them
- Several basic concepts
- Input layer : The data layer of the node
- The network layer : Output hihi Along with its network layer parameters w,bw,b
- Hidden layer : The middle layer of the network layer
- Output layer : The last layer
- Bias unit :bias unit, Add bias units to each layer
The activation unit and output of the above model are expressed as :

The expression of the three activation units :
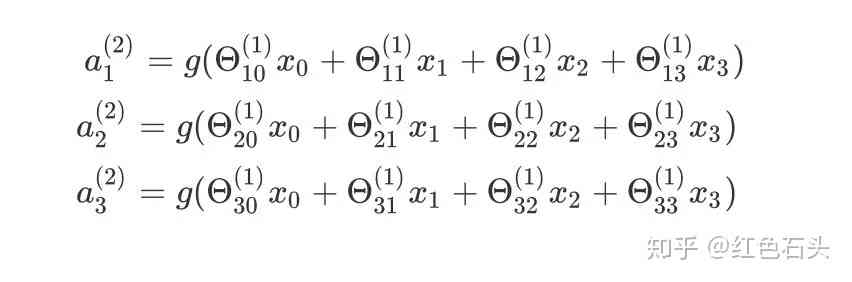
The output expression is :

Put each row of the eigenmatrix ( A training example ) Feed it to the neural network , Finally, we need to feed the whole training set to the neural network .
This left to right algorithm is called : Forward propagation FORWARD PROPAGATION
The memory method of model marking
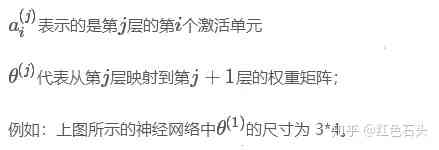
Its dimensions are specified as :
- By the end of jj The number of active cells in a layer is the number of rows
- By the end of j+1j+1 The number of active units in the layer +1 A matrix with the number of columns
Model to represent 2
FORWARD PROPAGATION Coding relative to using cycles , The vectorization method will make the calculation easier ,
If there is now :
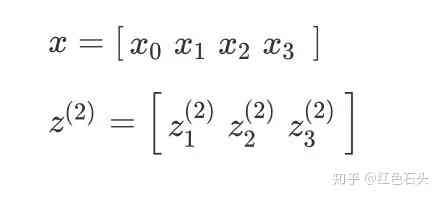
among z Satisfy :
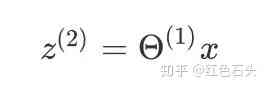
That is, in the brackets of the above three activation units , So there are :
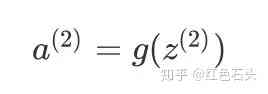

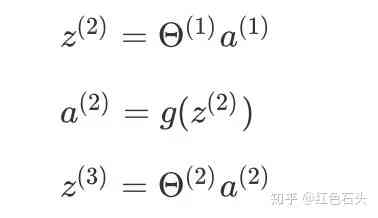
Then output h It can be expressed as :
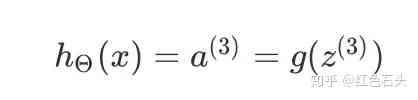
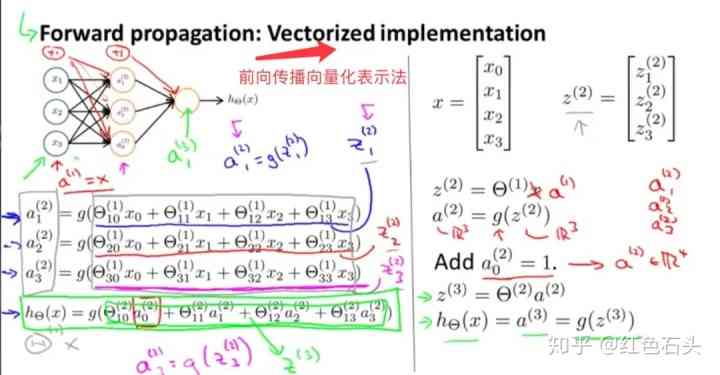
Features and intuitive understanding
Neural network , Monolayer neurons ( No middle layer ) Can be used to represent logical operations , For example, logic and (AND)、 Logic or (OR)
Implementation logic ” And AND”
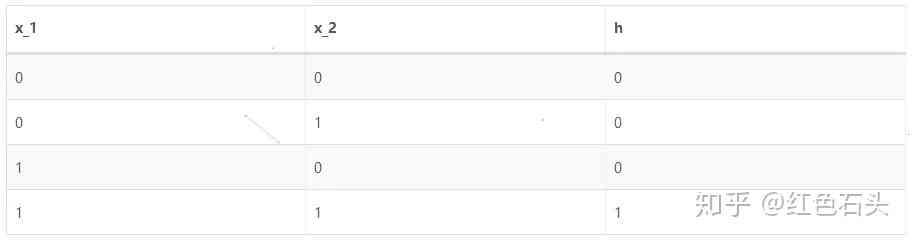
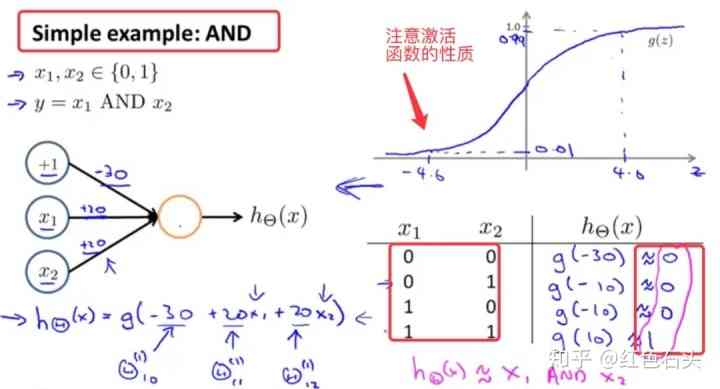
Implementation logic " or OR"
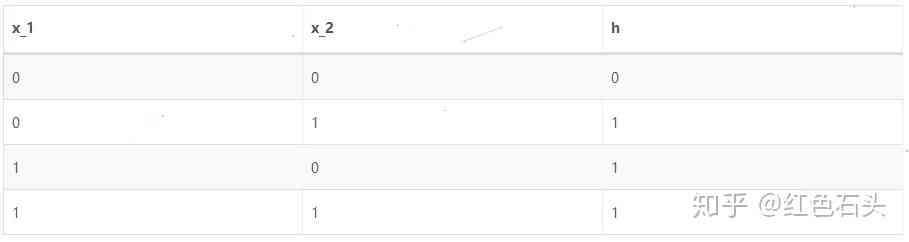

Implementation logic “ Not not”
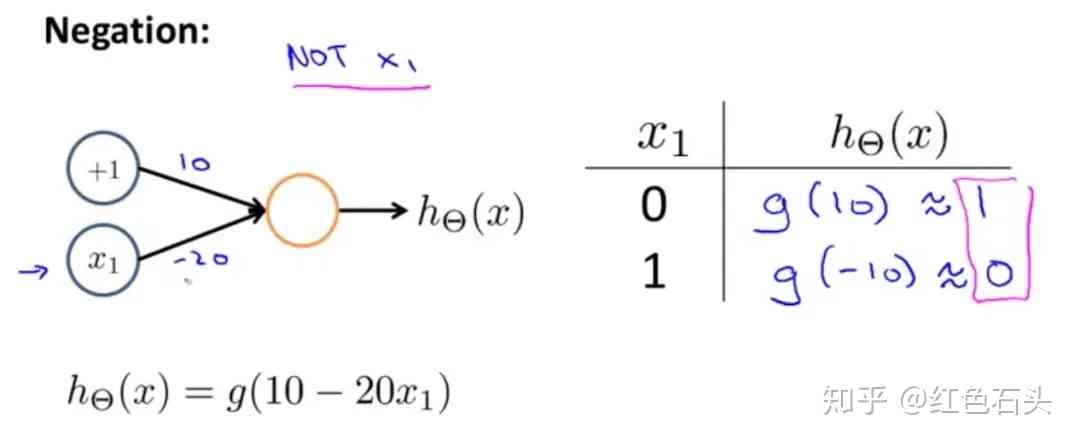
Multiple classification problems
When there are more than two categories in the output , For example, neural network algorithm is used to identify passers-by 、 automobile 、 Motorcycles, etc .
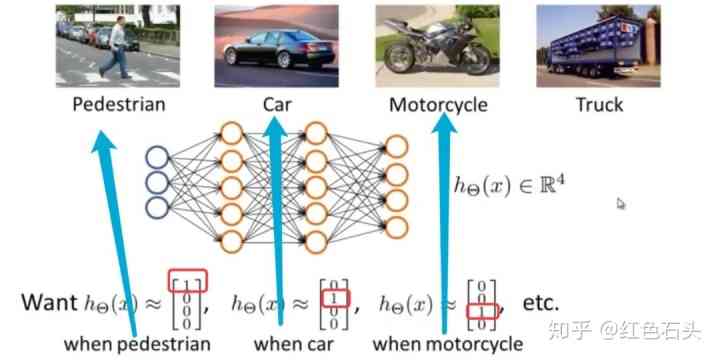
- The input vector has 3 Dimensions , Two intermediate layers
- The output layer has 4 Neurons represent 4 Kinds of classification , That is, every data will appear in the output layer [a,b,c,d]T[a,b,c,d]T, And [a,b,c,d][a,b,c,d] Only one of them is for 1, Represents the current class
TF The solution
The above multi class classification problem and TF The problem with Chinese handwritten numbers is similar to , The solution is as follows :
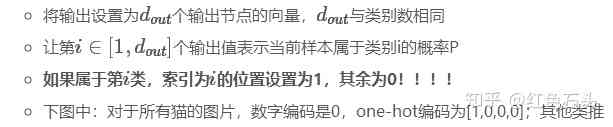
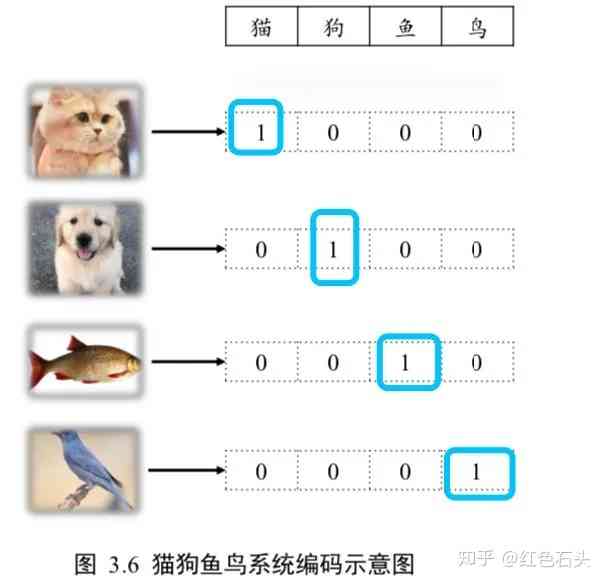
- Handwritten digital picture data
The total number of categories is 10, That is, the total output node value dout=10dout=10, Suppose the class of a sample is i, The number in the picture is ii, Need a length of 10 Vector yy, The index number is ii The position of is set to 1, The rest is 0.
- 0 Of one-hot Encoding is [1,0,0,0,….]
- 1 Of one-hot Encoding is [0,1,0,0,….]
- And so on
thus , Class notes for week 4 are over !
Series articles :
Wu enda 《Machine Learning》 Refining notes 1: Supervised learning and unsupervised learning
Wu enda 《Machine Learning》 Refining notes 2: Gradient descent and normal equation
Wu enda 《Machine Learning》 Refining notes 3: Regression problems and regularization
This article was first published on the official account :AI youdao (ID: redstonewill), Welcome to your attention !
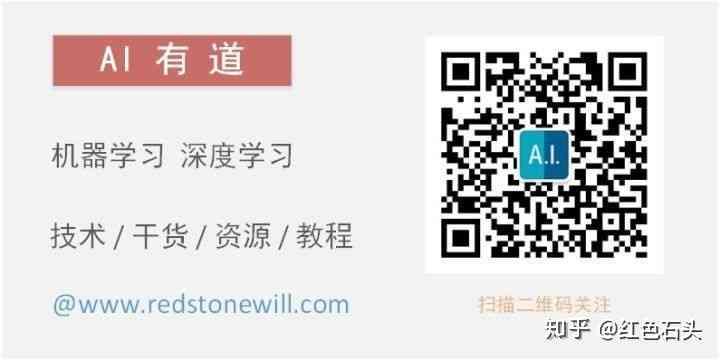
版权声明
本文为[osc_lhwd57ou]所创,转载请带上原文链接,感谢
边栏推荐
- 【LeetCode】 92 整数反转
- What's the difference between delete, truncate, and drop, and what to do if you delete data by mistake
- November 09, 2020: talk about the similarities and differences between the bulon filter and the cuckoo filter?
- Oschina: my green plants are potatoes, ginger and garlic
- CUDA_寄存器和局部存储器
- If you need a million objects
- 假如需要一百万个对象
- Unity使用transform.Rotate进行三维旋转角度出现偏差
- 分布式文档存储数据库之MongoDB索引管理
- Error running app:Default Activity not found 解决方法
猜你喜欢
推动中国制造升级,汽车装配车间生产流水线 3D 可视化
Bifrost 位点管理 之 异构中间件实现难点(1)
Coding style: SSM environment in MVC mode, code hierarchical management
An unsafe class named unsafe
Error running app:Default Activity not found 解决方法
Problems and solutions in configuring FTP server with FileZilla server
Must see! RDS database all in one
Function calculation advanced IP query tool development
初级工程师如何在职场生存
Yixian e-commerce prospectus of perfect diary parent company: focusing on marketing and ignoring R & D, with a loss of 1.1 billion in the first three quarters
随机推荐
jt-day10
Simple use of JMeter
Connection to XXX could not be established. Broker may not be available
大专学历的我工作六年了,还有机会进大厂吗?
初级工程师如何在职场生存
Assign the corresponding key and value in the map to the object
Mongodb index management of distributed document storage database
Problems and solutions in configuring FTP server with FileZilla server
Must see! RDS database all in one
CUDA_获取指定设备
Aikang Guobin denounced Guoxin Securities report as untrue and sent a lawyer's letter
[leetcode] 92 integer inversion
So what should investors do with the current market? Now a new investment outlet is coming!
Gets the property value of a column in the list collection object
【LeetCode】 92 整数反转
Top 5 Chinese cloud manufacturers in 2018: Alibaba cloud, Tencent cloud, AWS, telecom, Unicom
mac终端Iterm2支持rz和sz的解决方案
Top 5 Chinese cloud manufacturers in 2018: Alibaba cloud, Tencent cloud, AWS, telecom, Unicom
一个名为不安全的类Unsafe
proxy 的不完整polyfill