当前位置:网站首页>有趣的opencv-记录图片二值化和相似度实现
有趣的opencv-记录图片二值化和相似度实现
2022-08-03 20:31:00 【公众号:arigeweixin】
一、背景:
前面的文章提到,要实现两个功能
实现数字识别 实现图标识别
前面的文章已经已经实现了数字的识别,但是发现识别率比较低,并且识别的错误率也比较高。考虑是因为背景比较复杂影响了识别效果,本文主要解决复杂背景的简化,以及图片的对比
二、目标:
通过opencv对图片处理,使背景和内容有更加明显的差异,即二值化 通过opencv对比二值化后的图片的相似度,来识别图标
三、实现过程:
opencv的集成
1. 下载opencv对应的版本 https://opencv.org/releases/
我使用了最新的4.6.0 的版本
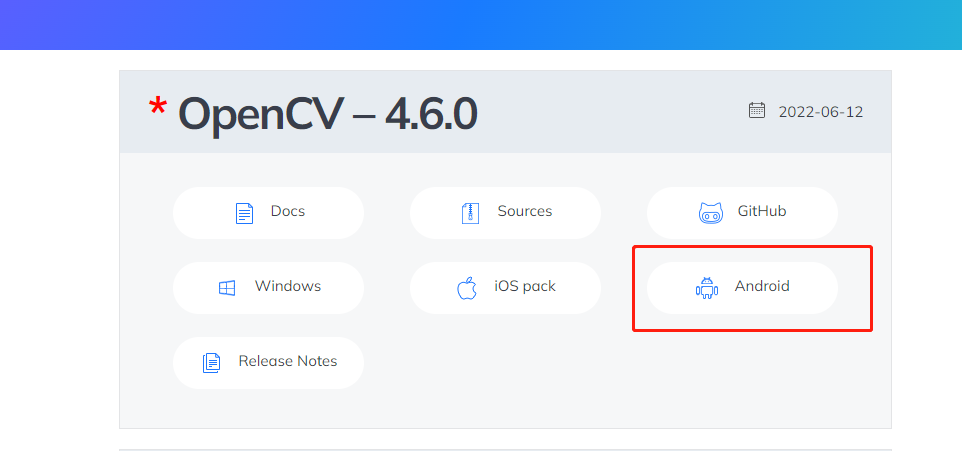
2. 解压下载的zip得到demo和需要集成的module
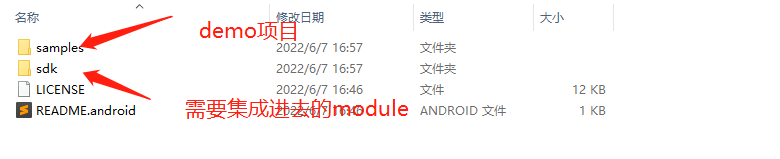
3. 接入自己的项目
3.1、将2中得到的sdk作为一个独立module放到项目中
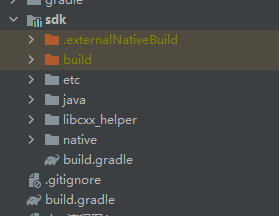
3.2、在项目的setting.gradle中引入sdk module
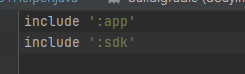
3.3、 在使用opencv的module中引入sdk module
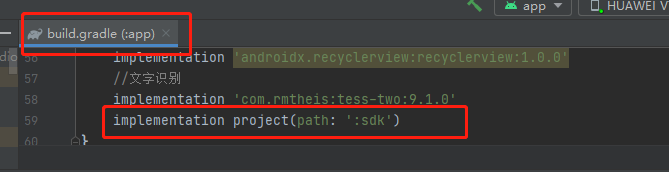
4. 编译使用
至此集成算是完成了,但是因为这个使用了ndk相关的功能,如果本地没有ndk环境的话,可能需要处理下环境问题,这里不再赘述
二值化处理
1. 先上代码
public static Bitmap createBitmap(Bitmap bitmap) {
Mat src = new Mat();
Utils.bitmapToMat(bitmap, src); //将bitmap转换为Mat
Mat thresholdImage = new Mat(src.size(), src.type()); //这个二值图像用于找出关键信息的图像
//将图像转换为灰度图像
Imgproc.cvtColor(src, thresholdImage, Imgproc.COLOR_RGBA2GRAY);
//将图像转换为边缘二值图像
Imgproc.threshold(thresholdImage,thresholdImage,10.0,255.0, Imgproc.THRESH_BINARY_INV|Imgproc.THRESH_OTSU);
Bitmap binaryBitmap = Bitmap.createBitmap(bitmap.getWidth(), bitmap.getHeight(), bitmap.getConfig());
Utils.matToBitmap(thresholdImage, binaryBitmap);
return binaryBitmap;
}
2. 步骤拆解
2.1、先将bitmap转为 Mat方便opencv进行操作
2.2、 调用 Imgproc.cvtColor(src, thresholdImage, Imgproc.COLOR_RGBA2GRAY);
将图像转为灰度图像
2.3、 调用 ` Imgproc.threshold(thresholdImage,thresholdImage,10.0,255.0, Imgproc.THRESH_BINARY_INV|Imgproc.THRESH_OTSU);
` 将图像转为二值图像。 这个方法非常的重要,在这里专门说一下
1. 第一个参数是图像来源
2. 第二个参数是输出的图像
3. 是一个标准。每个像素点会和这个标准比较。这个会和最后一个参数关联在一起使用。
4. 在符合第三个参数的标准的情况下,要赋予的值
5. 第五参数控制第三、第四个参数的使用情况
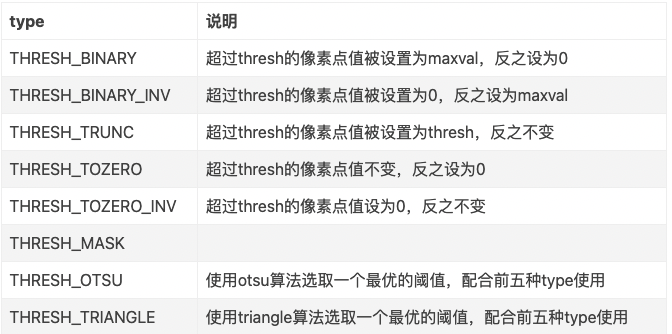
3. 效果展示


相似度处理
1. 上代码
public static Double similarity(Bitmap bitmap1, Bitmap bitmap2){
// 计算每张图片的特征点
MatOfKeyPoint descriptors1 = computeDescriptors(bitmap1);
MatOfKeyPoint descriptors2 = computeDescriptors(bitmap2);
// 比较两张图片的特征点
DescriptorMatcher descriptorMatcher = DescriptorMatcher.create(DescriptorMatcher.FLANNBASED);
List<MatOfDMatch> matches =new ArrayList();
// 计算大图中包含多少小图的特征点。
// 如果计算小图中包含多少大图的特征点,结果会不准确。
// 比如:若小图中的 50 个点都包含在大图中的 100 个特征点中,则计算出的相似度为 100%,显然不符合我们的预期
if (bitmap1.getByteCount() > bitmap2.getByteCount() ) {
descriptorMatcher.knnMatch(descriptors1, descriptors2, matches, 2);
} else {
descriptorMatcher.knnMatch(descriptors2, descriptors1, matches, 2);
}
Log.i("~~~", "matches.size: ${matches.size}");
if (matches.isEmpty()) return 0.00;
// 获取匹配的特征点数量
int matchCount = 0;
// 邻近距离阀值,这里设置为 0.7,该值可自行调整
float nndrRatio = 0.7f;
for (MatOfDMatch match:matches) {
DMatch[] array = match.toArray();
// 用邻近距离比值法(NNDR)计算匹配点数
if (array[0].distance <= array[1].distance * nndrRatio) {
matchCount++;
}
}
Log.i("~~~", "matchCount: $matchCount");
return Double.valueOf(matchCount/ matches.size());
}
2. 步骤拆解
2.1、 找到两个图片的特征点
private static MatOfKeyPoint computeDescriptors(Bitmap bitmap){
Mat mat = new Mat();
Utils.bitmapToMat(bitmap, mat);
MatOfKeyPoint keyPoints = new MatOfKeyPoint();
siftDetector.detect(mat, keyPoints);
MatOfKeyPoint descriptors = new MatOfKeyPoint();
// 计算图片的特征点
siftDetector.compute(mat, keyPoints, descriptors);
return descriptors;
}
2.2、 选取合适的匹配模式
2.3、避免 大图中包含小图导致认为小图完全匹配大图的场景,所以对大小进行判断,用大图和小图比。
2.4、 选取一个邻近距离阈值
2.5、 比较两个图的特征值的差异,,将差值符合阈值的个数记录
2.6、 符合阈值的个数,比上总特征数,得到匹配率
总结
opencv提供了一整套非常完善的api,可以解决我们遇到的绝大部分场景,大家可以多看文档,学习起来。
关注公众号: arigeweixin ,取得更多联系

边栏推荐
猜你喜欢
【HiFlow】经常忘记签到怎么办?使用腾讯云场景连接器每天提醒你。
高并发,你真的理解透彻了吗?
Lecture topics and guest blockbuster, TDengine developers conference to promote data technology "broken"
为什么 BI 软件都搞不定关联分析
RNA核糖核酸修饰荧光染料|HiLyte Fluor 488/555/594/647/680/750标记RNA核糖核酸
Power button - 203 - remove the list elements linked list
(十六)51单片机——红外遥控
【飞控开发高级教程3】疯壳·开源编队无人机-定高、定点、悬停
NAACL 2022 | 具有元重加权的鲁棒自增强命名实体识别技术
双线性插值公式推导及Matlab实现
随机推荐
ES6-箭头函数
leetcode 1837. The sum of the digits in the K-base representation
2022 年值得尝试的 7 个 MQTT 客户端工具
Leetcode sword refers to Offer 15. 1 in the binary number
ES6 - Arrow Functions
力扣707-设计链表——链表
Kubernetes资源编排系列之三: Kustomize篇 作者 艄公(杨京华) 雪尧(郭耀星)
5 款漏洞扫描工具:实用、强力、全面(含开源)
信使mRNA甲基化偶联3-甲基胞嘧啶(m3C)|mRNA-m3C
Alexa染料标记RNA核糖核酸|RNA-Alexa 514|RNA-Alexa 488|RNA-Alexa 430
glusterfs build and use
leetcode refers to Offer 58 - II. Left Rotate String
调用EasyCVR云台控制接口时,因网络延迟导致云台操作异常该如何解决?
Go语言为任意类型添加方法
一种能有效缓解环境噪声对音频质量干扰的方案
CLIP论文解读
leetcode 899. 有序队列
leetcode 072. Finding Square Roots
消除对特权账户的依赖使用Kaniko构建镜像
Why BI software can't handle correlation analysis