当前位置:网站首页>Hands on deep learning (III) -- convolutional neural network CNN
Hands on deep learning (III) -- convolutional neural network CNN
2022-07-04 20:47:00 【Long way to go 2021】
1 Image convolution
1.1 Cross correlation operation
stay ⼆ In dimensional cross-correlation operations , Convolution window from input ⼊ The upper left of tensor ⻆ Start , From left to right 、 Slide from top to bottom . When the convolution window slides to the new ⼀ Location (s) , The partial tensor contained in this window is related to the convolution kernel tensor ⾏ Multiply by elements , Then sum the tensors and get ⼀ A list ⼀ Scalar value of , From this we get this ⼀ Output tensor value of position . As shown in the figure below :
Be careful , Output ⼤ Little is less than losing ⼊⼤ Small . This is because the width of convolution kernel and ⾼ degree ⼤ On 1, The convolution kernel is only related to each ⼤ Small and completely suitable position ⾏ Cross correlation operation . therefore , Output ⼤ Less than equal to lose ⼊⼤ Small n h × n w n_h \times n_w nh×nw Subtract the convolution kernel ⼤ Small k h × k w k_h\times k_w kh×kw, namely :
( n h − k h + 1 ) × ( n w − k w + 1 ) (n_h-k_h+1)\times(n_w-k_w+1) (nh−kh+1)×(nw−kw+1)
This is because we need to ⾜ Enough space on the image “ Move ” Convolution kernel . later , We'll see how to fill in zeros around the image boundary to ensure that ⾜ Enough space to move the kernel , So as to maintain the output ⼤ Small unchanged . Next , We are corr2d
Function to implement the above process , This function accepts input ⼊ tensor X And convolution kernel tensor K, And return the output tensor Y.
import torch
def corr2d(X, K):
""" Calculate two-dimensional cross-correlation operation """
h, w = K.shape
Y = torch.zeros((X.shape[0] - h +1, X.shape[1] - w +1))
for i in range(Y.shape[0]):
for j in range(Y.shape[1]):
Y[i, j] = (X[i:i+h, j: j+w] * K).sum()
return Y
transport ⼊ tensor X And convolution kernel tensor K, To verify the above ⼆ Output of dimensional cross-correlation operation .
X = torch.tensor([[3, 3, 2, 1, 0], [0, 0, 1, 3, 1], [3, 1, 2, 2, 3], [2, 0, 0, 2, 2], [2, 0, 0, 0, 1]])
K = torch.tensor([[0, 1, 2], [2, 2, 0], [0, 1, 2]])
corr2d(X, K) # tensor([[12., 12., 17.], [10., 17., 19.], [ 9., 6., 14.]])
1.2 Convolution layer
Convolution layer transport ⼊ And convolution kernel weight ⾏ Cross correlation operation , And after adding scalar offset ⽣ Output . therefore , The two trained parameters in the convolution layer are convolution kernel weight and scalar offset . Just like before, we randomly initialize the full connection layer ⼀ sample , When training a convolution based model , We also initialize convolution kernel weights randomly .
Based on ⾯ Defined corr2d Function implementation ⼆ Convolution layer . stay __init__
In the constructor , take weight and bias Declared as two model parameters . Forward propagation function modulation ⽤corr2d Function and add offset .
from torch import nn
class Conv2D(nn.Module):
def __init__(self, kernel_size):
super().__init__()
self.weight = nn.Parameter(torch.rand(kernel_size))
self.bias = nn.Parameter(torch.zeros(1))
def forward(self ,x):
return corr2d(x, self.weight) + self.bias
1.3 Edge detection of target in image
The following is the convolution ⼀ A simple answer ⽤: By finding the location where the pixel changes , To detect different colors in the image ⾊ The edge of .⾸ First , We construct ⼀ individual 6 × 8 6 \times 8 6×8 Pixel ⿊⽩ Images . The middle four columns are ⿊⾊(0), The rest of the pixels are ⽩⾊(1).
X = torch.ones(6, 8)
X[:, 2:6] = 0
Next , structure ⼀ individual ⾼ Degree is 1、 Width is 2 Convolution kernel K. When in ⾏ Cross correlation operation , If ⽔ The two adjacent elements are the same , The output is zero , Otherwise, the output is ⾮ zero .
K = torch.tensor([[1.0, -1.0]])
Now? , We have parameters X( transport ⼊) and K( Convolution kernel ) Of board ⾏ Cross correlation operation . As follows ⽰, Output Y Medium 1 For from ⽩⾊ To ⿊⾊ The edge of ,-1 For from ⿊⾊ To ⽩⾊ The edge of , The output of other cases is 0.
Y = corr2d(X, K)
Y
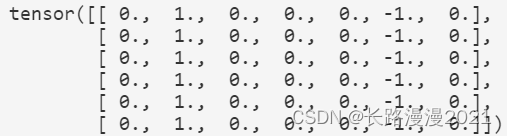
Will lose ⼊ Of ⼆ Dimensional image transpose , Before going into ⾏ The above cross-correlation operation . Its output is as follows , The previously detected vertical edge disappears . It is as expected , This convolution kernel K Only vertical edges can be detected ,⽆ Method of detection ⽔ Flat edge .
corr2d(X.T, K)
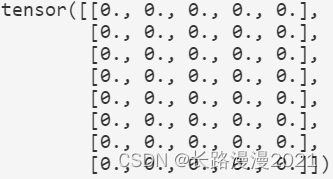
2 Fill and stride
2.1 fill
In response to ⽤ Multilayer convolution , We often lose edge pixels . Because we usually make ⽤ Small convolution kernel , So for any single convolution , We may only lose ⼏ Pixel . But as we should ⽤ Many continuous convolutions , The cumulative number of lost pixels is more . The simple way to solve this problem ⽅ Method is filling (padding): Losing ⼊ The boundary filling elements of the image ( Usually the filling element is 0). for example , In the following illustration , We will 3 × 3 3\times3 3×3 transport ⼊ Fill in 5 × 5 5\times5 5×5, Then its output will increase to 4 × 4 4\times4 4×4. The shaded part is the ⼀ Output elements and ⽤ Output of output calculation ⼊ And nuclear tensor elements : 0 × 0 + 0 × 1 + 0 × 2 + 0 × 3 = 0 0 \times 0 + 0 \times 1 + 0 \times 2 + 0 \times 3 = 0 0×0+0×1+0×2+0×3=0.
Usually , If we add p h p_h ph⾏ fill (⼤ about ⼀ Half at the top ,⼀ Half at the bottom ) and p w p_w pw Column fill ( left ⼤ about ⼀ And a half , On the right side ⼀ And a half ), The output shape will be
( n h − k h + p h + 1 ) × ( n w − k w + p w + 1 ) (n_h-k_h+p_h+1)\times(n_w-k_w+p_w+1) (nh−kh+ph+1)×(nw−kw+pw+1)
This means that the output ⾼ Degree and width will be increased respectively p h p_h ph and p w p_w pw.
in many instances , We need to set p h = k h − 1 p_h = k_h - 1 ph=kh−1 and p w = k w − 1 p_w = k_w - 1 pw=kw−1, Lose ⼊ And output have the same ⾼ Degree and width . This allows you to build ⽹ It is easier to predict the output shape of each layer . hypothesis k h k_h kh Is odd , We will be in ⾼ Fill both sides of degrees p h / 2 p_h/2 ph/2⾏. If k h k_h kh It's even , be ⼀ One possibility is to lose ⼊ Top filling ⌈ p h / 2 ⌉ ⌈p_h/2⌉ ⌈ph/2⌉⾏, Fill the bottom with ⌊ p h / 2 ⌋ ⌊p_h/2⌋ ⌊ph/2⌋⾏. Empathy , We fill both sides of the width .
Convolution nerves ⽹ Convolution kernel in complex ⾼ Degrees and widths are usually odd , for example 1、3、5 or 7. The advantage of choosing odd numbers is , While maintaining the spatial dimension , We can fill the top and bottom with the same number of ⾏, Fill the left and right with the same number of columns .
import torch
from torch import nn
def comp_conv2d(conv2d, X):
""" This function initializes the volume layer weight , And lose ⼊ And output ⾼ And reduce the corresponding dimension """
X = X.reshape((1, 1) + X.shape) # this ⾥ Of (1,1) surface ⽰ Batch ⼤⼩ And the number of channels 1
Y = conv2d(X)
return Y.reshape(Y.shape[2:]) # Omit the first two dimensions : Batch ⼤⼩ And channel
establish ⼀ individual ⾼ Degree and width are 3 Of ⼆ Convolution layer , And fill all sides 1 Pixel . Given ⾼ Degree and width are 8 The loss of ⼊, The output of the ⾼ Degree and width are also 8.
conv2d = nn.Conv2d(1, 1, kernel_size=3, padding=1) # Each side is filled 1⾏ or 1 Column , A total of 2⾏ or 2 Column
X = torch.rand(size=(8, 8))
comp_conv2d(conv2d, X).shape # torch.Size([8, 8])
When convolution kernel ⾼ Degree and width are different , We can fill in different ⾼ Degree and width , Make output and output ⼊ Have the same ⾼ Degree and width . In the following ⽰ In the example , We make ⽤⾼ Degree is 5, Width is 3 Convolution kernel ,⾼ The filling on both sides of degree and width are 2 and 1.
conv2d = nn.Conv2d(1, 1, kernel_size=(5, 3), padding=(2, 1))
comp_conv2d(conv2d, X).shape # torch.Size([8, 8])
2.2 Stride
Sometimes for ⾼ Efficient calculation or reducing the number of samples , The convolution window can skip the middle position , Slide multiple elements at a time .
The number of elements per slide is called stride (stride). To ⽬ The former is ⽌, We only make ⽤ too ⾼ Degree or width is 1 Stride , So how to make ⽤ a ⼤ The stride of ? The following figure shows the vertical stride of 3,⽔ The flat stride is 2 Of ⼆ Dimensional cross correlation operation . the ⾊ Part is the output element and ⽤ Output of output calculation ⼊ And kernel tensor elements : 0 × 0 + 0 × 1 + 1 × 2 + 2 × 3 = 8 、 0 × 0 + 6 × 1 + 0 × 2 + 0 × 3 = 6 0 \times 0 + 0 \times 1 + 1 \times 2 + 2 \times 3 = 8、0 \times 0 + 6 \times 1 + 0 \times 2 + 0 \times 3 = 6 0×0+0×1+1×2+2×3=8、0×0+6×1+0×2+0×3=6.

Usually , When the vertical stride is s h s_h sh、⽔ The flat stride is s w s_w sw when , The output shape is
⌊ ( n h − k h + p h + s h ) / s h ⌋ × ⌊ ( n w − k w + p w + s w ) / s w ⌋ \left\lfloor\left(n_{h}-k_{h}+p_{h}+s_{h}\right) / s_{h}\right\rfloor \times\left\lfloor\left(n_{w}-k_{w}+p_{w}+s_{w}\right) / s_{w}\right\rfloor ⌊(nh−kh+ph+sh)/sh⌋×⌊(nw−kw+pw+sw)/sw⌋
Next ⾯ take ⾼ The strides of degree and width are set to 2, Thus will lose ⼊ Of ⾼ Half the degree and width .
conv2d = nn.Conv2d(1, 1, kernel_size=3, padding=1, stride=2)
comp_conv2d(conv2d, X).shape # torch.Size([4, 4])
When the convolution kernel size of height and width is different , When the filling and step size are different ,
conv2d = nn.Conv2d(1, 1, kernel_size=(3, 5), padding=(0, 1), stride=(3, 4))
comp_conv2d(conv2d, X).shape # torch.Size([2, 2])
3 Multiple input multiple output channels
3.1 Multiple input channels
When you lose ⼊ When multiple channels are included , It needs to be constructed ⼀ One and lose ⼊ Data has the same input ⼊ Convolution kernel of channel number , In order to lose ⼊ Data into ⾏ Cross correlation operation . Suppose you lose ⼊ The number of channels is c i c_i ci, So the input of convolution kernel ⼊ The number of channels also needs to be c i c_i ci. If the window shape of convolution kernel is k h × k w k_h\times k_w kh×kw, So when c i = 1 c_i = 1 ci=1 when , We can think of the convolution kernel as having the shape k h × k w k_h \times k_w kh×kw Of ⼆ D tensor .
However , When c i > 1 c_i > 1 ci>1 when , Each input of our convolution kernel ⼊ The channel will contain a shape of k h × k w k_h\times k_w kh×kw Tensor . Put these tensors c i c_i ci Link to ⼀ You can get a shape of c i × k h × k w c_i \times k_h \times k_w ci×kh×kw Convolution kernel . Because of losing ⼊ And convolution kernel c i c_i ci Channels , We can input for each channel ⼊ Of ⼆ Dimensional tensor and convolution kernel ⼆ Dimensional tensor into ⾏ Cross correlation operation , Then sum the channels ( take c i c_i ci The results add up ) obtain ⼆ D tensor .
The picture below shows ⽰ 了 ⼀ One has two losses ⼊ The tunnel ⼆ Dimensional cross-correlation operation ⽰ example . The shaded part is the ⼀ Output elements and ⽤ To calculate the output of this output ⼊ And nuclear tensor elements : ( 1 × 1 + 2 × 2 + 4 × 3 + 5 × 4 ) + ( 0 × 0 + 1 × 1 + 3 × 2 + 4 × 3 ) = 56 (1\times 1+2\times 2+4\times 3+5\times 4)+(0\times 0+1\times 1+3\times 2+4\times 3) = 56 (1×1+2×2+4×3+5×4)+(0×0+1×1+3×2+4×3)=56.
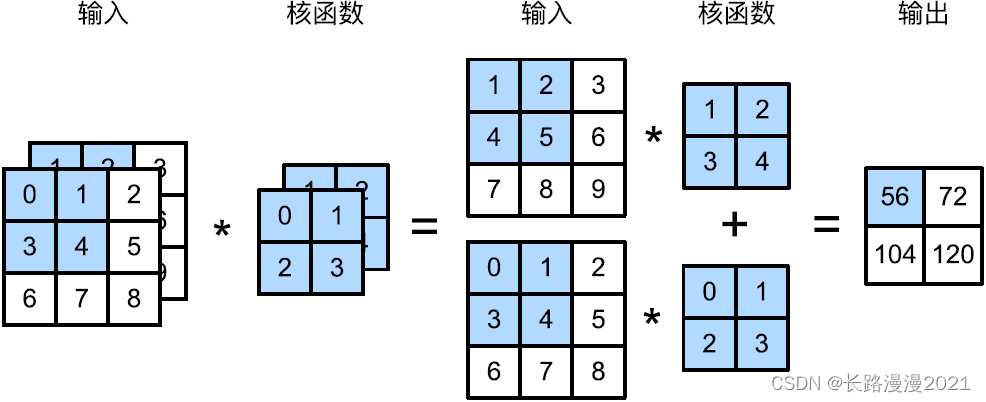
The following implementation ⼀ Lose more next ⼊ Channel cross correlation operation . Jane and ⾔ And , What we do is to hold on to each channel ⾏ Cross correlation operation , And then add up the results .
def corr2d_multi_in(X, K):
""" First traversal “X” and “K” Of the 0 Dimensions ( Channel dimension ), Add them to ⼀ rise """
return sum(d2l.corr2d(x, k) for x, k in zip(X, K))
3.2 Multiple output channels
At the top ⾏ The nerves of ⽹ Network structure , With the nerves ⽹ The deepening of the number of layers , We often increase the dimension of the output channel , By reducing the spatial resolution to obtain more ⼤ The depth of the passage . Intuitively speaking , We can think of each channel as a response to different characteristics . The reality may be more complicated ⼀ some , Because each channel is not unique ⽴ Study of the , But to work together ⽤ And optimized . therefore , Multiple output channels are not just detectors that learn multiple single channels .
⽤ c i c_i ci and c o c_o co Separate table ⽰ transport ⼊ And the number of output channels ⽬, And let k h k_h kh and k w k_w kw Is a convolution kernel ⾼ Degree and width . In order to obtain the output of multiple channels , We can create for each output channel ⼀ The shape is c i × k h × k w c_i \times k_h \times k_w ci×kh×kw Convolution kernel tensor , So the shape of the convolution kernel is c o × c i × k h × k w c_o \times c_i \times k_h \times k_w co×ci×kh×kw. In cross-correlation operation , Each output channel obtains all outputs first ⼊ passageway , Then the convolution kernel corresponding to the output channel is used to calculate the result .
As follows ⽰, We implement ⼀ A cross-correlation function that calculates the output of multiple channels .
def corr2d_multi_in_out(X, K):
# iteration “K” Of the 0 Dimensions , Lose every time ⼊“X” Of board ⾏ Cross correlation operation
# Finally, add all the results together
return torch.stack([corr2d_multi_in(X, k) for k in K], 0)
3.3 1×1 Convolution layer
send ⽤ The smallest Window , 1 × 1 1 \times 1 1×1 Convolution loses the unique energy of convolution ⼒—— stay ⾼ Degree and width dimensions , Identify the interaction between adjacent elements ⽤ Yes ⼒. Actually 1 × 1 1 \times 1 1×1 Convolution only ⼀ Calculate and send ⽣ On the aisle .
Show below ⽰ Made ⽤ 1 × 1 1\times 1 1×1 Convolution kernel and 3 A loss ⼊ Channels and 2 Cross correlation calculation of two output channels . this ⾥ transport ⼊ And output have the same ⾼ Degree and width , Every element in the output is a slave ⼊ Same in image ⼀ Linear combination of elements of position . We can 1 × 1 1\times 1 1×1 The convolution layer is seen as the position of each pixel should ⽤ The full connection layer of , With c i c_i ci A loss ⼊ Value to co The output values . Because it's still ⼀ Convolution layers , So the weight across pixels is ⼀ To . meanwhile , 1 × 1 1 \times 1 1×1 The weight dimension required by the convolution layer is c o × c i c_o \times c_i co×ci, Plus ⼀ A deviation .
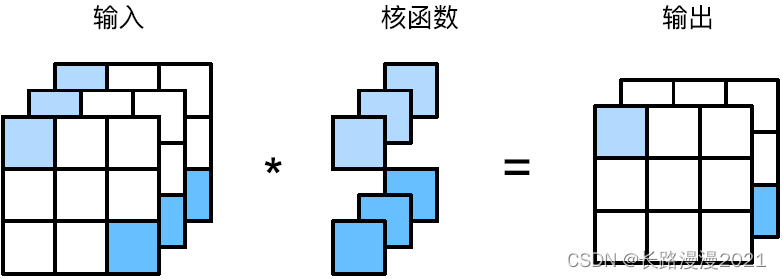
Next ⾯, We make ⽤ Full connection layer implementation 1 × 1 1 \times 1 1×1 Convolution . Please note that , We need to lose ⼊ And the output data shape ⾏ fine-tuning .
def corr2d_multi_in_out_1(X, K):
c_i, h, w = X.shape
c_o = K.shape[0]
X = X.reshape(c_i, h * w)
K = K.reshape(c_o, c_i)
# Matrix multiplication in fully connected layers
Y = torch.matmul(K, X)
return Y.reshape(c_o, h, w)
4 Convergence layer
4.1 Maximum convergence layer and average convergence layer
Similar to convolution , The convergence layer operator consists of ⼀ A fixed shaped window , The window depends on its stride ⼤ Small is losing ⼊ Slide on all areas of , Fixed shape window ( Sometimes called convergence window ) Each position traversed is calculated ⼀ Outputs . However , Different from the transport in the convolution ⼊ Cross correlation calculation with convolution kernel , The aggregation layer does not contain parameters . contrary , The pool operator is deterministic , We usually calculate the most ⼤ Value or average . These operations are called the most ⼤ Convergence layer (maximum pooling) And the average convergence layer (average pooling).
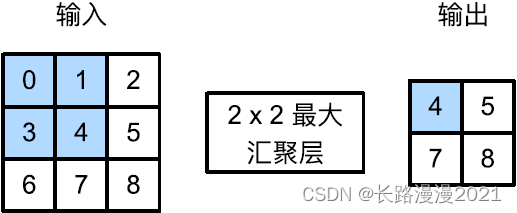
In both cases , And the cross-correlation operator ⼀ sample , Convergence window from input ⼊ The upper left of tensor ⻆ Start , From left to right 、 Losing from top to bottom ⼊ Tensor slip . At every location reached by the convergence window , It calculates the input in the window ⼊⼦ Tensor's most ⼤ Value or average . Calculate the most ⼤ The value or average depends on making ⽤ Most ⼤ Convergence layer or average convergence layer .
Under ⾯ In the code of pool2d
function , Realize forward propagation of convergence layer . This function is similar to that in the previous section corr2d
function . However , this ⾥ We have no convolution kernel , Output is output ⼊ The most ⼤ Value or average .
def pool2d(X, pool_size, mode='max'):
p_h, p_w = pool_size
Y = torch.zeros(X.shape[0] - p_h + 1, X.shape[1] - p_w + 1)
for i in range(Y.shape[0]):
for j in range(Y.shape[1]):
if mode == 'max':
Y[i, j] = X[i: i + p_h, j : j + p_w].max()
elif mode == 'avg':
Y[i, j] = X[i: i+p_h, j : j + p_w].mean()
return Y
4.2 Fill and stride
By default , Step length and convergence window in the deep learning framework ⼤ Little same . therefore , If we make ⽤ Shape is (3, 3) The convergence window , So by default , The step shape we get is (3, 3).
pool2d = nn.MaxPool2d((2, 3), padding= (0, 1), stride=(2, 3))
4.3 Multiple channels
Dealing with multi-channel transmission ⼊ Data time , The convergence layer is at each transmission ⼊ Separate operation on the channel , Not like a convolution ⼀ The sample is matched on the channel ⼊ Into the ⾏ Summary . This means that the number of output channels in the convergence layer is related to the output ⼊ Same number of channels .
边栏推荐
- 哈希(Hash)竞猜游戏系统开发功能分析及源码
- 【深度学习】一文看尽Pytorch之十九种损失函数
- tcp为啥是三次握手和四次挥手
- [in-depth learning] review pytoch's 19 loss functions
- go语言笔记(2)go一些简单运用
- #夏日挑战赛#带你玩转HarmonyOS多端钢琴演奏
- 语义化标签的优势和块级行内元素
- Ziguang zhanrui completed the first 5g R17 IOT NTN satellite on the Internet of things in the world
- 针对深度学习的“失忆症”,科学家提出基于相似性加权交错学习,登上PNAS
- mysql语句执行详解
猜你喜欢
阿里测试师用UI自动化测试实现元素定位
针对深度学习的“失忆症”,科学家提出基于相似性加权交错学习,登上PNAS
工厂从自动化到数字孪生,图扑能干什么?
Win11系统wifi总掉线怎么办?Win11系统wifi总掉线的解决方法
Win11U盘拒绝访问怎么办?Win11U盘拒绝访问的有效解决方法
[today in history] July 4: the first e-book came out; The inventor of magnetic stripe card was born; Palm computer pioneer was born
What if win11u disk refuses access? An effective solution to win11u disk access denial
C server log module
C # better operation mongodb database
Form组件常用校验规则-1(持续更新中~)
随机推荐
C server log module
B2B mall system development of electronic components: an example of enabling enterprises to build standardized purchase, sale and inventory processes
LeetCode 871. Minimum refueling times
分析伦敦银走势图的技巧
What if the computer page cannot be full screen? The solution of win11 page cannot be full screen
关于联邦学习和激励的相关概念(1)
jekins初始化密码没有或找不到
Flet tutorial 06 basic introduction to textbutton (tutorial includes source code)
Win11亮度被锁定怎么办?Win11亮度被锁定的解决方法
What ppt writing skills does the classic "pyramid principle" teach us?
PermissionError: [Errno 13] Permission denied: ‘data.csv‘
科普达人丨一文看懂阿里云的秘密武器“神龙架构”
Idea restore default shortcut key
What if win11u disk refuses access? An effective solution to win11u disk access denial
See how Tencent does interface automation testing
为什么最大速度是光速
word中插入图片后,图片上方有一空行,且删除后布局变乱
Integritee通过XCM集成至Moonriver,为其生态系统带来企业级隐私解决方案
Talking about cookies of client storage technology
Reinforcement learning - learning notes 2 | value learning