当前位置:网站首页>[deep learning] AI one click to change the sky
[deep learning] AI one click to change the sky
2022-07-08 01:00:00 【InfoQ】
1. The goal of the experiment
2. The content of the case is introduced
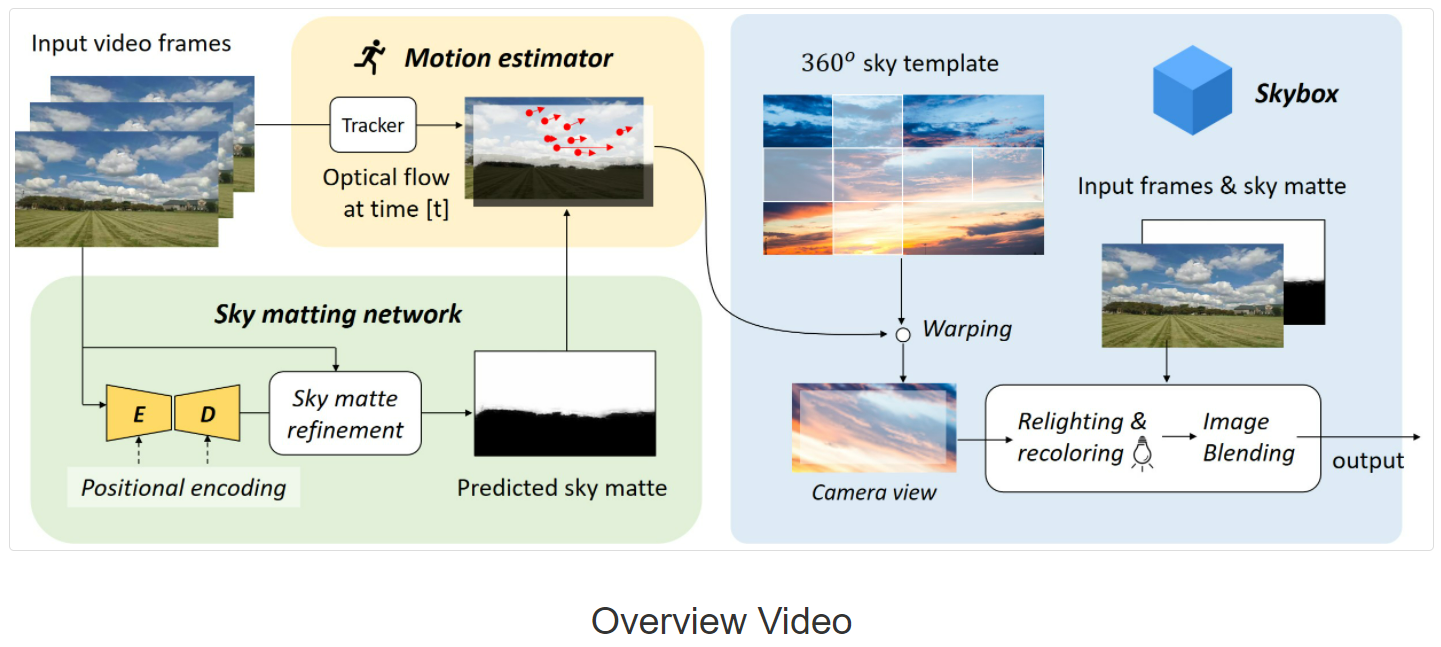
3. The experimental steps
3.1 Install and import dependent packages
import os
import moxing as mox
file_name = 'SkyAR'
if not os.path.exists(file_name):
mox.file.copy('obs://modelarts-labs-bj4-v2/case_zoo/SkyAR/SkyAR.zip', 'SkyAR.zip')
os.system('unzip SkyAR.zip')
os.system('rm SkyAR.zip')
mox.file.copy_parallel('obs://modelarts-labs-bj4-v2/case_zoo/SkyAR/resnet50-19c8e357.pth', '/home/ma-user/.cache/torch/checkpoints/resnet50-19c8e357.pth')
!pip uninstall opencv-python -y
!pip uninstall opencv-contrib-python -y
!pip install opencv-contrib-python==4.5.3.56
cd SkyAR/
import time
import json
import base64
import numpy as np
import matplotlib.pyplot as plt
import cv2
import argparse
from networks import *
from skyboxengine import *
import utils
import torch
from IPython.display import clear_output, Image, display, HTML
%matplotlib inline
# If there is GPU It's in GPU Run above
device = torch.device("cuda:0" if torch.cuda.is_available() else "cpu")
3.2 Set algorithm parameters
parameter = {
"net_G": "coord_resnet50",
"ckptdir": "./checkpoints_G_coord_resnet50",
"input_mode": "video",
"datadir": "./test_videos/sky.mp4", # The original video path to be processed
"skybox": "sky.jpg", # The path of the sky picture to be replaced
"in_size_w": 384,
"in_size_h": 384,
"out_size_w": 845,
"out_size_h": 480,
"skybox_center_crop": 0.5,
"auto_light_matching": False,
"relighting_factor": 0.8,
"recoloring_factor": 0.5,
"halo_effect": True,
"output_dir": "./jpg_output",
"save_jpgs": False
}
str_json = json.dumps(parameter)
3.3 Preview the original video
video_name = parameter['datadir']
def arrayShow(img):
img = cv2.resize(img, (0, 0), fx=0.25, fy=0.25, interpolation=cv2.INTER_NEAREST)
_,ret = cv2.imencode('.jpg', img)
return Image(data=ret)
# Open a video stream
cap = cv2.VideoCapture(video_name)
frame_id = 0
while True:
try:
clear_output(wait=True) # Clear the previous display
ret, frame = cap.read() # Read a picture
if ret:
frame_id += 1
if frame_id > 200:
break
cv2.putText(frame, str(frame_id), (5, 15), cv2.FONT_HERSHEY_SIMPLEX, 0.5, (0, 255, 0), 1) # draw frame_id
tmp = cv2.cvtColor(frame, cv2.COLOR_BGR2RGB) # Convert color mode
img = arrayShow(frame)
display(img) # display picture
time.sleep(0.05) # The thread sleeps for a period of time before processing the next picture
else:
break
except KeyboardInterrupt:
cap.release()
cap.release()
Preview the sky picture you want to replace
img= cv2.imread(os.path.join('./skybox', parameter['skybox']))
img2 = img[:, :, ::-1]
plt.imshow(img2)
3.4 Definition SkyFilter class
class Struct:
def __init__(self, **entries):
self.__dict__.update(entries)
def parse_config():
data = json.loads(str_json)
args = Struct(**data)
return args
args = parse_config()
class SkyFilter():
def __init__(self, args):
self.ckptdir = args.ckptdir
self.datadir = args.datadir
self.input_mode = args.input_mode
self.in_size_w, self.in_size_h = args.in_size_w, args.in_size_h
self.out_size_w, self.out_size_h = args.out_size_w, args.out_size_h
self.skyboxengine = SkyBox(args)
self.net_G = define_G(input_nc=3, output_nc=1, ngf=64, netG=args.net_G).to(device)
self.load_model()
self.video_writer = cv2.VideoWriter('out.avi',
cv2.VideoWriter_fourcc(*'MJPG'),
20.0,
(args.out_size_w, args.out_size_h))
self.video_writer_cat = cv2.VideoWriter('compare.avi',
cv2.VideoWriter_fourcc(*'MJPG'),
20.0,
(2*args.out_size_w, args.out_size_h))
if os.path.exists(args.output_dir) is False:
os.mkdir(args.output_dir)
self.output_img_list = []
self.save_jpgs = args.save_jpgs
def load_model(self):
# Load the pre trained sky matting model
print('loading the best checkpoint...')
checkpoint = torch.load(os.path.join(self.ckptdir, 'best_ckpt.pt'),
map_location=device)
self.net_G.load_state_dict(checkpoint['model_G_state_dict'])
self.net_G.to(device)
self.net_G.eval()
def write_video(self, img_HD, syneth):
frame = np.array(255.0 * syneth[:, :, ::-1], dtype=np.uint8)
self.video_writer.write(frame)
frame_cat = np.concatenate([img_HD, syneth], axis=1)
frame_cat = np.array(255.0 * frame_cat[:, :, ::-1], dtype=np.uint8)
self.video_writer_cat.write(frame_cat)
# Define the result buffer
self.output_img_list.append(frame_cat)
def synthesize(self, img_HD, img_HD_prev):
h, w, c = img_HD.shape
img = cv2.resize(img_HD, (self.in_size_w, self.in_size_h))
img = np.array(img, dtype=np.float32)
img = torch.tensor(img).permute([2, 0, 1]).unsqueeze(0)
with torch.no_grad():
G_pred = self.net_G(img.to(device))
G_pred = torch.nn.functional.interpolate(G_pred,
(h, w),
mode='bicubic',
align_corners=False)
G_pred = G_pred[0, :].permute([1, 2, 0])
G_pred = torch.cat([G_pred, G_pred, G_pred], dim=-1)
G_pred = np.array(G_pred.detach().cpu())
G_pred = np.clip(G_pred, a_max=1.0, a_min=0.0)
skymask = self.skyboxengine.skymask_refinement(G_pred, img_HD)
syneth = self.skyboxengine.skyblend(img_HD, img_HD_prev, skymask)
return syneth, G_pred, skymask
def cvtcolor_and_resize(self, img_HD):
img_HD = cv2.cvtColor(img_HD, cv2.COLOR_BGR2RGB)
img_HD = np.array(img_HD / 255., dtype=np.float32)
img_HD = cv2.resize(img_HD, (self.out_size_w, self.out_size_h))
return img_HD
def process_video(self):
# Process video frame by frame
cap = cv2.VideoCapture(self.datadir)
m_frames = int(cap.get(cv2.CAP_PROP_FRAME_COUNT))
img_HD_prev = None
for idx in range(m_frames):
ret, frame = cap.read()
if ret:
img_HD = self.cvtcolor_and_resize(frame)
if img_HD_prev is None:
img_HD_prev = img_HD
syneth, G_pred, skymask = self.synthesize(img_HD, img_HD_prev)
self.write_video(img_HD, syneth)
img_HD_prev = img_HD
if (idx + 1) % 50 == 0:
print(f'processing video, frame {idx + 1} / {m_frames} ... ')
else: # If you reach the last frame
break
3.5 Start processing video
sf = SkyFilter(args)
sf.process_video()
3.6 Compare the original video with the processed video
video_name = "compare.avi"
def arrayShow(img):
_,ret = cv2.imencode('.jpg', img)
return Image(data=ret)
# Open a video stream
cap = cv2.VideoCapture(video_name)
frame_id = 0
while True:
try:
clear_output(wait=True) # Clear the previous display
ret, frame = cap.read() # Read a picture
if ret:
frame_id += 1
cv2.putText(frame, str(frame_id), (5, 15), cv2.FONT_HERSHEY_SIMPLEX, 0.5, (0, 255, 0), 1) # draw frame_id
tmp = cv2.cvtColor(frame, cv2.COLOR_BGR2RGB) # Convert color mode
img = arrayShow(frame)
display(img) # display picture
time.sleep(0.05) # The thread sleeps for a period of time before processing the next picture
else:
break
except KeyboardInterrupt:
cap.release()
cap.release()
3.7 Generate your own day changing video
边栏推荐
- 新库上线 | CnOpenData中华老字号企业名录
- AI遮天传 ML-回归分析入门
- 【愚公系列】2022年7月 Go教学课程 006-自动推导类型和输入输出
- Malware detection method based on convolutional neural network
- A network composed of three convolution layers completes the image classification task of cifar10 data set
- 12. RNN is applied to handwritten digit recognition
- 接口测试进阶接口脚本使用—apipost(预/后执行脚本)
- ThinkPHP kernel work order system source code commercial open source version multi user + multi customer service + SMS + email notification
- Cve-2022-28346: Django SQL injection vulnerability
- Qt添加资源文件,为QAction添加图标,建立信号槽函数并实现
猜你喜欢
Semantic segmentation model base segmentation_ models_ Detailed introduction to pytorch
[OBS] the official configuration is use_ GPU_ Priority effect is true
【obs】官方是配置USE_GPU_PRIORITY 效果为TRUE的
What does interface testing test?
12. RNN is applied to handwritten digit recognition
Introduction to ML regression analysis of AI zhetianchuan
New library online | cnopendata China Star Hotel data
基于微信小程序开发的我最在行的小游戏
Qt添加资源文件,为QAction添加图标,建立信号槽函数并实现
Cve-2022-28346: Django SQL injection vulnerability
随机推荐
【愚公系列】2022年7月 Go教学课程 006-自动推导类型和输入输出
Binder core API
Codeforces Round #804 (Div. 2)(A~D)
Introduction to ML regression analysis of AI zhetianchuan
Image data preprocessing
[reprint] solve the problem that CONDA installs pytorch too slowly
Invalid V-for traversal element style
牛客基础语法必刷100题之基本类型
【GO记录】从零开始GO语言——用GO语言做一个示波器(一)GO语言基础
11.递归神经网络RNN
How is it most convenient to open an account for stock speculation? Is it safe to open an account on your mobile phone
Reentrantlock fair lock source code Chapter 0
Leetcode brush questions
Password recovery vulnerability of foreign public testing
国外众测之密码找回漏洞
STL -- common function replication of string class
The whole life cycle of commodity design can be included in the scope of industrial Internet
German prime minister says Ukraine will not receive "NATO style" security guarantee
New library online | information data of Chinese journalists
【obs】官方是配置USE_GPU_PRIORITY 效果为TRUE的