当前位置:网站首页>The difference between gbdt and XGB, and the mathematical derivation of gradient descent method and Newton method
The difference between gbdt and XGB, and the mathematical derivation of gradient descent method and Newton method
2020-11-06 01:28:00 【Elementary school students in IT field】
Why the gradient descent method and Newton method are introduced ?
Two algorithms are mentioned here GBDT and XGBoost, All two are boosting Model .
GBDT and xgb The objective function of is different , At the same time, aiming at the error function in the objective function L(θ) There are also differences in the way of fitting :
- GBDT Let's use the first order Taylor expansion to expand two terms , Make an approximation
- xgboost Let's use the second order Taylor expansion to expand the three terms , Make an approximation
Words mean , - GBDT The gradient descent method is used to optimize in function space
- XGBoost Using Newton's method to optimize in function space
The final objective function only depends on the first and second derivatives of each data point on the error function .
The error function can be customized , Let's say the square loss function : ( y i , y i ) = ( y i − y i ) 2 (yi,y^i) = (yi−y^i)2 (yi,yi)=(yi−yi)2, or logistic Loss function
More introductions and suggestions to listen to :https://study.163.com/course/courseMain.htm?courseId=1006401020&share=2&shareId=400000000645014
1. The derivation of the gradient descent method
Gradient descent method is widely used in machine learning and deep learning , The general course or textbook will explain the gradient descent method in a visualized way ( Quadratic curve 、 Lower convex surface, etc ), I think we all know how to use visualization to illustrate the effectiveness of gradient descent method . here , I'm not going to repeat this kind of figurative explanation . I use mathematical derivation to prove the effectiveness of the gradient descent method .
We should all know the Taylor expansion of function of one variable , The formula is as follows :
f ( x ) = f ( x 0 ) + f ’ ( x 0 ) 1 ! ( x − x 0 ) + f ’ ’ ( x 0 ) 2 ! ( x − x 0 ) 2 + f ’ ’ ’ ( x 0 ) 3 ! ( x − x 0 ) 3 + … f(x)=f(x_0)+\frac{f’(x_0)}{1!}(x-x_0)+\frac{f’’(x_0)}{2!}(x-x_0)^2+\frac{f’’’(x_0)}{3!}(x-x_0)^3+… f(x)=f(x0)+1!f’(x0)(x−x0)+2!f’’(x0)(x−x0)2+3!f’’’(x0)(x−x0)3+…
Let's just take the first two terms of the formula on the right , That's one “ About equal to ” Result :
f ( x ) = f ( x 0 ) + f ’ ( x 0 ) 1 ! ( x − x 0 ) f(x)=f(x_0)+\frac{f’(x_0)}{1!}(x-x_0) f(x)=f(x0)+1!f’(x0)(x−x0)
remember : Δ x = x − x 0 \Delta x=x-x_0 Δx=x−x0, The above formula becomes :
f ( x ) = f ( x 0 ) + f ’ ( x 0 ) 1 ! Δ x f(x)=f(x_0)+\frac{f’(x_0)}{1!}\Delta x f(x)=f(x0)+1!f’(x0)Δx
Our goal is to make the following equation hold in the iterative process , In other words, it is hoped that the function value will gradually decrease during the iteration process , To describe in mathematical language is : f ( x n + 1 ) ≤ f ( x n ) f(x_{n+1})\leq f(x_{n}) f(xn+1)≤f(xn)
Easy to think of , It should be constructed :
Δ x = − f ’ ( x 0 ) \Delta x=-f’(x_0) Δx=−f’(x0)
here :
f ( x ) = f ( x 0 ) − f ’ ( x 0 ) 2 f(x)=f(x_0)-f’(x_0)^2 f(x)=f(x0)−f’(x0)2
Write it in iterative form :
f ( x n + 1 ) = f ( x n ) − f ’ ( x n ) 2 f(x_{n+1})=f(x_{n})-f’(x_{n})^2 f(xn+1)=f(xn)−f’(xn)2
because f ’ ( x ) 2 ≥ 0 f’(x)^2\geq 0 f’(x)2≥0, We have completed the proof of the effectiveness of gradient descent . The formula of parameter iterative updating summarized from the above steps is as follows :
f ( x n + 1 ) = f ( x n ) − f ’ ( x n ) 2 f(x_{n+1})=f(x_{n})-f’(x_{n})^2 f(xn+1)=f(xn)−f’(xn)2
The above steps prove the effectiveness of gradient descent on a function of one variable . It is easy to generalize to multivariate functions . in addition , In multivariate functions , It can also be added that the gradient direction is the fastest direction of descent .
See : You know Why does gradient descent find a minimum ?
2. Newton method
That's the gradient descent method , By the way, the derivation of Newton's method . Because Newton's method is also derived from Taylor expansion .
Keep watching Taylor unfold :
f ( x ) = f ( x 0 ) + f ’ ( x 0 ) 1 ! ( x − x 0 ) + f ’ ’ ( x 0 ) 2 ! ( x − x 0 ) 2 + f ’ ’ ’ ( x 0 ) 3 ! ( x − x 0 ) 3 + … f(x)=f(x_0)+\frac{f’(x_0)}{1!}(x-x_0)+\frac{f’’(x_0)}{2!}(x-x_0)^2+\frac{f’’’(x_0)}{3!}(x-x_0)^3+… f(x)=f(x0)+1!f’(x0)(x−x0)+2!f’’(x0)(x−x0)2+3!f’’’(x0)(x−x0)3+…
still , Let's take the right front 2 term :
f ( x ) = f ( x 0 ) + f ’ ( x 0 ) 1 ! ( x − x 0 ) f(x)=f(x_0)+\frac{f’(x_0)}{1!}(x-x_0) f(x)=f(x0)+1!f’(x0)(x−x0)
Take derivatives on both sides of the equation :
f ’ ( x ) = f ’ ( x 0 ) + f ’ ’ ( x 0 ) 1 ! ( x − x 0 ) f’(x)=f’(x_0)+\frac{f’’(x_0)}{1!}(x-x_0) f’(x)=f’(x0)+1!f’’(x0)(x−x0)
f ’ ( x ) = f ’ ( x 0 ) + f ’ ’ ( x 0 ) 1 ! Δ x f’(x)=f’(x_0)+\frac{f’’(x_0)}{1!}\Delta x f’(x)=f’(x0)+1!f’’(x0)Δx
According to the nature of calculus , f ( x ) f(x) f(x) When you take the minimum , Yes f ’ ( x ) = 0 f’(x)=0 f’(x)=0, Let's put this property into the equation above , Yes :
0 = f ’ ( x 0 ) + f ’ ’ ( x 0 ) 1 ! Δ x 0=f’(x_0)+\frac{f’’(x_0)}{1!}\Delta x 0=f’(x0)+1!f’’(x0)Δx
Δ x = − f ’ ( x 0 ) f ’ ’ ( x 0 ) \Delta x=-\frac{f’(x_0)}{f’’(x_0)} Δx=−f’’(x0)f’(x0)
In this way, we get the parameter iterative updating formula of Newton method as follows :
x n + 1 = x n − f ’ ( x n ) f ’ ’ ( x n ) x_{n+1}=x_{n}-\frac{f’(x_n)}{f’’(x_n)} xn+1=xn−f’’(xn)f’(xn)
3. The difference between the gradient descent method and Newton's method
From the above proof process, we can see that , Although both gradient descent method and Newton method can be derived by Taylor expansion , But there's a little bit of a difference in the way the reasoning is based .
In practice , Newton method and gradient descent method are widely used in machine learning . The difference between the two is actually written in many blogs , such as : gradient descent or Quasi Newton method ?
4. Quasi Newton method
In the parameter iterative updating formula of Newton method above , We can see f ’ ’ ( x 0 ) f’’(x_0) f’’(x0) It's in the denominator . remember , The mathematical derivation above is a function of one variable , For multivariate functions , The existence of this denominator is equivalent to calculating Hessian The inverse of the matrix , It's very difficult and time-consuming . therefore , Many variations of Newton's algorithm have appeared , This kind of deformation is called quasi Newton algorithm .BFGS It's an iterative method to approximate the Hessian matrix . and BFGS We need to store the approximate Hessian matrix , So there's an improved version L-BFGS.
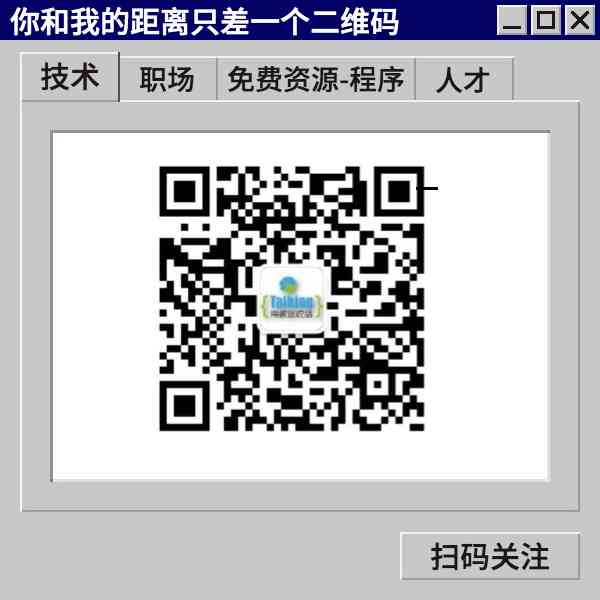
版权声明
本文为[Elementary school students in IT field]所创,转载请带上原文链接,感谢
边栏推荐
- 一篇文章带你了解CSS对齐方式
- Working principle of gradient descent algorithm in machine learning
- Process analysis of Python authentication mechanism based on JWT
- This article will introduce you to jest unit test
- Skywalking series blog 5-apm-customize-enhance-plugin
- Filecoin主网上线以来Filecoin矿机扇区密封到底是什么意思
- PHPSHE 短信插件说明
- html
- 一篇文章带你了解CSS 分页实例
- How to become a data scientist? - kdnuggets
猜你喜欢
Vue 3 responsive Foundation
Word segmentation, naming subject recognition, part of speech and grammatical analysis in natural language processing
零基础打造一款属于自己的网页搜索引擎
Mac installation hanlp, and win installation and use
教你轻松搞懂vue-codemirror的基本用法:主要实现代码编辑、验证提示、代码格式化
keras model.compile Loss function and optimizer
用一个例子理解JS函数的底层处理机制
I think it is necessary to write a general idempotent component
What is the side effect free method? How to name it? - Mario
How to select the evaluation index of classification model
随机推荐
Skywalking series blog 2-skywalking using
PHPSHE 短信插件说明
Let the front-end siege division develop independently from the back-end: Mock.js
How long does it take you to work out an object-oriented programming interview question from Ali school?
Analysis of etcd core mechanism
Save the file directly to Google drive and download it back ten times faster
NLP model Bert: from introduction to mastery (2)
零基础打造一款属于自己的网页搜索引擎
2019年的一个小目标,成为csdn的博客专家,纪念一下
Common algorithm interview has been out! Machine learning algorithm interview - KDnuggets
Programmer introspection checklist
What to do if you are squeezed by old programmers? I don't want to quit
Summary of common algorithms of binary tree
Nodejs crawler captures ancient books and records, a total of 16000 pages, experience summary and project sharing
5.5 controlleradvice notes - SSM in depth analysis and project practice
git rebase的時候捅婁子了,怎麼辦?線上等……
Python基础变量类型——List浅析
一篇文章带你了解CSS3图片边框
Filecoin最新动态 完成重大升级 已实现四大项目进展!
5.4 static resource mapping