当前位置:网站首页>Pytorch: tensor operation (I) contiguous
Pytorch: tensor operation (I) contiguous
2022-07-06 12:13:00 【harry_ tea】
Contents of this article
tensor Storage in memory
Information area and storage area
tensor The storage in memory contains Information area and Storage area
- Information area (Tensor) contain tensor The shape of the size, step stride, data type type etc.
- Storage area (Storage) Contains stored data
High dimensional arrays are stored in memory according to row priority , What is row priority ? Suppose we have a (3, 4) Of tensor, It is actually stored as a one-dimensional array , But in tensor The information area of recorded his size and stride As a result, the array actually displayed is two-dimensional ,size by (3, 4)
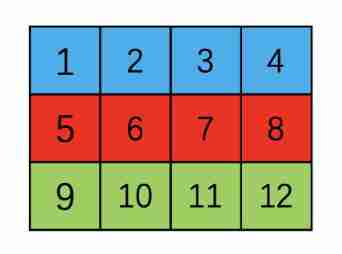
Two dimensional array

One dimensional form in memory
Now let's look at an example , Examples show that tensor The elements in are contiguous in memory , And it also proves that it is indeed row priority storage
tensor = torch.tensor([[[1 ,2, 3, 4], [5, 6, 7, 8], [9, 10,11,12]],
[[13,14,15,16],[17,18,19,20],[21,22,23,24]]])
print(tensor.is_contiguous())
for i in range(2):
for j in range(3):
for k in range(4):
print(tensor[i][j][k].data_ptr(), end=' ')
''' True 140430616343104 140430616343112 140430616343120 140430616343128 140430616343136 140430616343144 140430616343152 140430616343160 140430616343168 140430616343176 140430616343184 140430616343192 140430616343200 140430616343208 140430616343216 140430616343224 140430616343232 140430616343240 140430616343248 140430616343256 140430616343264 140430616343272 140430616343280 140430616343288 '''
shape && stride
Continuing with the above example, let's take a look at shape and stride attribute , about (2, 3, 4) Dimensional tensor His shape by (2, 3 ,4),stride by (12, 4, 1)
shape
shape It's easy to understand , Namely tensor Dimensions , The above example is (2, 3, 4) Of tensor, Dimension is (2, 3, 4)
stride
stride Represents the step size of multidimensional index , Each step represents an offset in memory +1, about (2, 3, 4) Dimensional tensor:stride+1 Represents the (dim2)+1,stride+4 For the rest dim unchanged ,(dim1)+1,stride+12 For the rest dim unchanged ,(dim0)+1, As shown in the figure below
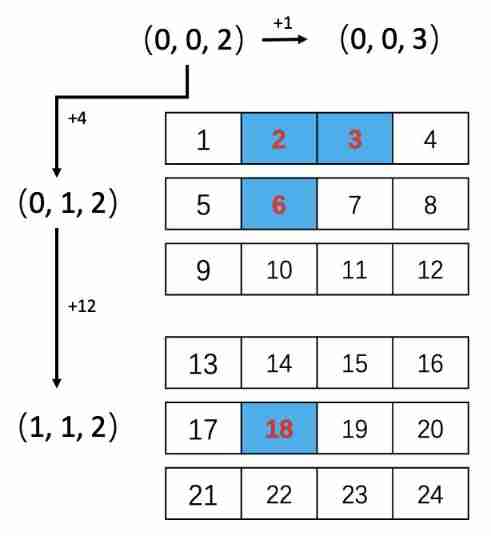
Icon stride
stride computing method
s t r i d e i = s t r i d e i + 1 ∗ s i z e i + 1 i ∈ [ 0 , n − 2 ] stride_{i} = stride_{i+1} * size_{i+1}~~~~i\in[0, n-2] stridei=stridei+1∗sizei+1 i∈[0,n−2]
about shape(2, 3, 4) Of tensor, The calculation is as follows (stride3=1)
s t r i d e 2 = s t r i d e 3 ∗ s h a p e 3 = 1 ∗ 4 = 4 s t r i d e 1 = s t r i d e 2 ∗ s h a p e 2 = 4 ∗ 3 = 12 stride_{2} = stride_{3} * shape_{3}=1*4=4 \\ stride_{1} = stride_{2} * shape_{2}=4*3=12 stride2=stride3∗shape3=1∗4=4stride1=stride2∗shape2=4∗3=12
stride = [1] # Initialize the first element
# Iterate back and forward to generate stride
for i in range(len(tensor.size())-2, -1, -1):
stride.insert(0, stride[0] * tensor.shape[i+1])
print(stride) # [12, 4, 1]
print(tensor.stride()) # (12, 4, 1)
I understand tensor After storage in memory , Let's see contiguous
contiguous
contiguous
Returns a continuous memory tensor
Returns a contiguous in memory tensor containing the same data as self tensor. If self tensor is already in the specified memory format, this function returns the self tensor.
When to use contiguous Well ?
The simple understanding is tensor Use when the storage order in the memory address is inconsistent with the actual one-dimensional index order , As shown below , Right up there tensor One dimensional index , The result is [1, 2, 3, 4, 5, 6, 7, 8, 9, 10, 11, 12], Original tensor Application transpose To transpose , In the one-dimensional index , The result is [1, 5, 9, 2, 6, 10, 3, 7, 11, 4, 8, 12], At this time, the index order changes , So we need to use contiguous
Be careful : No matter how it changes, the corresponding address of each element is unchanged , such as 11 The corresponding address is x11,transpose after 11 Still corresponding x11, So what has changed ? Remember tensor Is it divided into information area and storage area , The storage area does not change , What changes is the information area shape,stride Etc , Make an introduction when you have time ~
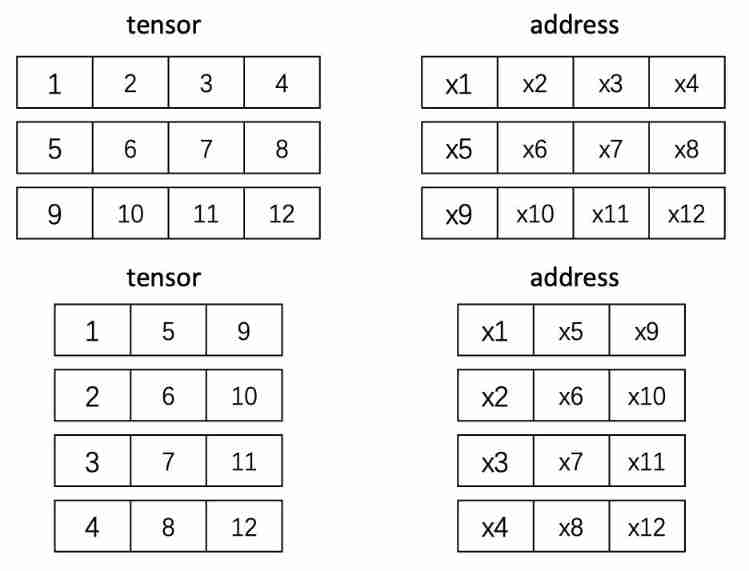
Code example
tensor = torch.tensor([[1,2,3,4],[5,6,7,8],[9,10,11,12]])
print(tensor)
print(tensor.is_contiguous())
tensor = tensor.transpose(1, 0)
print(tensor)
print(tensor.is_contiguous())
''' tensor([[ 1, 2, 3, 4], [ 5, 6, 7, 8], [ 9, 10, 11, 12]]) True tensor([[ 1, 5, 9], [ 2, 6, 10], [ 3, 7, 11], [ 4, 8, 12]]) False '''
Why use contiguous
Some people may have questions , Since the above index is different from before ( It's not continuous ), Why make him continuous ? because pytorch Some operations of require index and memory continuity , such as view
Code example ( Next, let's take the example above )
tensor = tensor.contiguous()
print(tensor.is_contiguous())
tensor = tensor.view(3, 4)
print(tensor)
''' True tensor([[ 1, 5, 9, 2], [ 6, 10, 3, 7], [11, 4, 8, 12]]) '''
If not contiguous The following errors will be reported
RuntimeError: view size is not compatible with input tensor's size and stride (at least one dimension spans across two contiguous subspaces). Use .reshape(...) instead.
Why? contiguous It works ?
contiguous In a simple and crude way , Since your previous index and memory are not continuous , Then I'll reopen a piece of continuous memory and index it
Code example , From the following code stride Changes can be seen ,transpose After that tensor It really changes the information in the information area
tensor = torch.tensor([[1,2,3,4],[5,6,7,8],[9,10,11,12]])
print(tensor.is_contiguous()) # True
for i in range(3):
for j in range(4):
print(tensor[i][j], tensor[i][j].data_ptr(), end=' ')
print()
print(tensor.stride()) # (4, 1)
''' True tensor(1) 140430616321664 tensor(2) 140430616321672 tensor(3) 140430616321680 tensor(4) 140430616321688 tensor(5) 140430616321696 tensor(6) 140430616321704 tensor(7) 140430616321712 tensor(8) 140430616321720 tensor(9) 140430616321728 tensor(10) 140430616321736 tensor(11) 140430616321744 tensor(12) 140430616321752 (4, 1) '''
tensor = tensor.transpose(1, 0)
print(tensor.is_contiguous()) # False
for i in range(4):
for j in range(3):
print(tensor[i][j], tensor[i][j].data_ptr(), end=' ')
print()
print(tensor.stride()) # (1, 4) changed
''' False tensor(1) 140430616321664 tensor(5) 140430616321696 tensor(9) 140430616321728 tensor(2) 140430616321672 tensor(6) 140430616321704 tensor(10) 140430616321736 tensor(3) 140430616321680 tensor(7) 140430616321712 tensor(11) 140430616321744 tensor(4) 140430616321688 tensor(8) 140430616321720 tensor(12) 140430616321752 (1, 4) '''
tensor = tensor.contiguous()
print(tensor.is_contiguous()) # True
for i in range(4):
for j in range(3):
print(tensor[i][j], tensor[i][j].data_ptr(), end=' ')
print()
print(tensor.stride()) # (3, 1)
''' True tensor(1) 140431681244608 tensor(5) 140431681244616 tensor(9) 140431681244624 tensor(2) 140431681244632 tensor(6) 140431681244640 tensor(10) 140431681244648 tensor(3) 140431681244656 tensor(7) 140431681244664 tensor(11) 140431681244672 tensor(4) 140431681244680 tensor(8) 140431681244688 tensor(12) 140431681244696 (3, 1) '''
边栏推荐
- Pat 1097 duplication on a linked list (25 points)
- Basic operations of databases and tables ----- creating data tables
- Pytorch实现简单线性回归Demo
- @Autowired 和 @Resource 的区别
- Working principle of genius telephone watch Z3
- VSCode基础配置
- uCOS-III 的特点、任务状态、启动
- JS object and event learning notes
- Whistle+switchyomega configure web proxy
- Arduino get random number
猜你喜欢
A possible cause and solution of "stuck" main thread of RT thread
Characteristics, task status and startup of UCOS III
Cannot change version of project facet Dynamic Web Module to 2.3.
Amba, ahb, APB, Axi Understanding
I2C总线时序详解
Esp8266 uses Arduino to connect Alibaba cloud Internet of things
Oppo vooc fast charging circuit and protocol
C语言回调函数【C语言】
Arduino uno R3 register writing method (1) -- pin level state change
RT-Thread 线程的时间片轮询调度
随机推荐
ESP8266使用arduino连接阿里云物联网
Raspberry pie tap switch button to use
imgcat使用心得
1081 rational sum (20 points) points add up to total points
ES6语法总结--上篇(基础篇)
记一次云服务器被密码爆破的经历——关小黑屋、改密码、改端口
Kaggle competition two Sigma connect: rental listing inquiries (xgboost)
高通&MTK&麒麟 手机平台USB3.0方案对比
MySQL takes up too much memory solution
Basic operations of databases and tables ----- modifying data tables
Time slice polling scheduling of RT thread threads
Reno7 60W super flash charging architecture
I2C总线时序详解
数据分析之缺失值填充(重点讲解多重插值法Miceforest)
VSCode基础配置
Pytorch-温度预测
Learning notes of JS variable scope and function
2022.2.12 resumption
ESP8266通过arduino IED连接巴法云(TCP创客云)
列表的使用