当前位置:网站首页>Centerx: open centernet in the way of socialism with Chinese characteristics
Centerx: open centernet in the way of socialism with Chinese characteristics
2022-07-07 03:07:00 【Tom Hardy】
The author 丨 [email protected] You know
Source https://zhuanlan.zhihu.com/p/323814368
Edit the market platform
Reading guide
The author put aside the tradition by combining the expression bag PR The seriousness of the manuscript , Use humorous and humorous language to explain their own reconstruction centernet Code for , Adopt the core thought of socialism with Chinese characteristics to explain centerX Of trick.centerX It is divided into five modules , It provides you with a feasible idea .
It's too long to read the edition
The author reconstructs a version centernet(objects as points) Code for , And added distillation , Multi model distillation , turn caffe, turn onnx, turn tensorRT, The post-processing is also done in the network forward , Very friendly to landing .
Let's play one. centerX Multi model distillation out of the renderings , The label of the data set is not used in distillation , Only two teacher Of model Distill the same student The Internet . We'll make it with our wives demo Well .
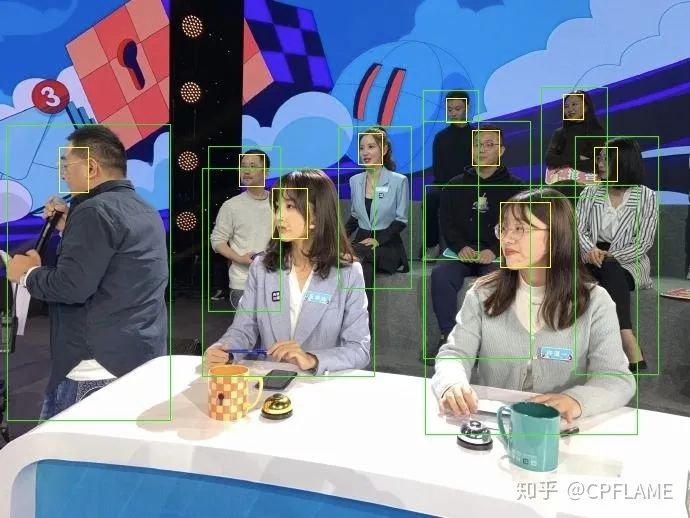
If you are not interested in children's shoes, you can collect the expression bag of the writer , If you think the emoticon bag is fun , Kneel down and beg to go github give the thumbs-up .
Code address :https://github.com/CPFLAME/centerX/github.com
Preface
centernet It's one of my favorite testing articles , No, anchor, No, nms, Simple structure , Strong expandability , The main thing is : Landing is extremely convenient , Choose a simple one backbone, There can be no bug Turn it into the model you want (caffe,onnx,tensorRT). And the post-processing is extremely convenient .
however centernet The original code was a little laborious at first , But there's no idea of refactoring , After some time, I found centernet-better and centernet-better-plus, So they put their code in vain, and then improve it by themselves , Form a code style that is friendly to me .( Of course, the most plagiarism is fast reID and detectron2)

The joy of whoring for nothing
Because I don't like to write pure technical blog , I don't want to write a pure article PR draft ( From the beginning of undergraduate, I hated pain and wrote experimental reports ), I don't want to make people feel that reading this article is learning , So this article is not very serious , There's no one holding one step at a time , It's different from other people's propaganda .
After all, the code is not about fighting , It's the sophistication of the world , If you really want to learn something, you have to read other people's articles , Look at mine, just for fun .
Publicity part
Generally speaking, people who read articles will take such a psychology when they click in , Why should I use it centerX, Obviously, I used other frames very well , It's a lot of trouble to turn around, you know , You're teaching me to do things ?

If you need to land fast with detection algorithms , We need a model that is fast and accurate , And it can turn around without pit caffe,onnx,tensorRT, At the same time, there is no need to write post-processing , that centerX It will suit you very well .( Original centernet The post-processing needs additional 3X3 pooling and topK The operation of , By the author with a very Sao operation put in the network )
If you want to experience the pleasure of model distillation on the test task , stay baseline It's painless , Or find some detection The distillation of inspiration , You can come centerX Kang Kang .
If you only have two singleton annotated datasets at the same time , But you don't have to fill in the missing category labels of these two datasets , You can try it centerX A detector is trained to predict two kinds of labels simultaneously .
If you want to be based on centernet Do some academic research , You can also be in centerX Of projects Refactoring your own code inside , and centerX Inside centernet Of codebase Not conflict , Can quickly locate bug.
If you're a tough student or a pathetic tool person , You can use it. centerX To manage your teacher or leader upward , because centerX Inside mAP It's not high , A little adjustment or add something can surpass my own baseline, When the time comes to report, you can pat your chest and say that the things you run out are several points higher than the author , And then your KPI You can have a little bit of security .( At the end of the article, I will give you some directions about how to run higher than the author )

centerX The underlying framework of the white from the excellent detection framework detectron2, If you've run before detectron2 Experience , I believe it can be the same as master Ma's lightning with five lashes , The use of seamless convergence .
without detectron2 Using experience of , It doesn't matter , I wrote about the lazy fool run.sh, It just needs to be changed config And run the command can be happy to run .
If none of the above reasons moves you , So if I amuse you with this article , Beg to go github Give me one star Well .
The core idea of the code
Inspired by the old leader Taoist thinking programming ,centerX Of trick It also carries out some of the central themes of socialism with Chinese characteristics .
Code cv Dafa ———— si
Model distillation ———— The rich lead the rich
Multi model distillation , Two single class detection models are fused into a multi class detection model ———— Saints have no regular teachers
communism loss, Solve the model for lr It's too sensitive ———— Marxism
Put the post-processing into the neural network ———— Unite our true friends , To attack our real enemies , Distinguish between ourselves and the enemy .《 Wool picking 》
centerX Each module
Basic implementation
There is nothing to say about this , And it doesn't differentiate from other frameworks , It's just detectron2 On the basis of centernet It's just a reproduction , And most of the code is from centernet-better and centernet-better-plus, Just go straight to COCO The results of the experiment on .
Backbone by resnet50
centerX_KD Yes, it is 27.9 Of resnet18 As a student network ,33.2 Of resnet50 As a result of teacher network distillation , The detailed process is described in the following chapters .
Backbone by resnet18
Model distillation
Daga good , I am a detection. I often look at the village next door with envy classification,embedding Waiting for a playmate , They're all mixed up in distillation , what logits Distillation , what KL The divergence , what Overhaul of Feature Distillation. Every day there are different tutors to guide them , Why should I detection There are very few educational resources , I detection When can I stand up !
The main reason for the above is that detection The paradigm of is more complex , It's not like the village next door classification embedding Etc , Start with a picture , Output one vector:
1.two stage The network itself is due to rpn The uncertainty of the output , Lead to teacher and student Of proposal Alignment is a big problem .
2. I've tried in the middle layer feature Distillation on top of , So you can be lazy and don't have to write the last logits Distillation part of the code , It turns out there's no egg , Still have to be logits The upper distillation is more stable .
3. I can't make it up
Let's look back centernet The paradigm of , Oh , My god , What a simple paradigm :
1. Network output three heads , A prediction center , A prediction of width and height , The offset of a predicted center point
2. No complex positive and negative sample sampling , Only the center of the object is a positive sample , The rest are negative samples
This let the author see in detection The hope of the tutor , So we copied centernet original loss Writing , It's modeled on a distilled loss. Specific implementation can go to code Look inside , Here's a simple idea .
1. For the output center point head, hold teacher and student Output head feature map Have a time relu layer , Take out the negative number , And make one mse Of loss, Just OK 了 .
2. For output width, height and center point head, According to the original centernet The implementation of is to learn only positive samples , I want to do this in a way : We use it teacher Output center point head After that relu After that feature As a coefficient , At the width, height and center point head All pixels on the L1 loss Multiply the coefficients before and after .
3. In distillation , Three head Distillation of loss Difference is very big , You need to manually adjust your own loss weight, Generally in 300 After iterations, each distillation loss stay 0~3 It will be better between .
4. So before I was 300 Time epoch And then stop it , And then according to the current loss Estimate one loss weight Start training again . This stupid operation came up with communism in my head for another time loss And then get rid of .
5. In model distillation, we can combine... On labeled data label Of loss Training , We can also use the output of teacher network to distill training on unlabeled datasets . Based on this feature, we have a lot of magic
6. When combining on tagged data label Of loss When training , The teacher says N individual epoch, Student training N individual epoch, Then the teacher taught the students , And keep the original label loss Retraining N individual epoch, So the students mAP It's the highest training .
7. When distilling training on an unlabeled dataset , We're going out of the data set limit , Let's start with the label data set N individual epoch, Then the teacher distilled the student model training on the unlabeled dataset N individual epoch, It can make the accuracy ratio of student model to baseline higher , And the generalization performance is better .
8. Before that centernet Of source code There was an experiment on , The same network , You can distill yourself, and you can go up . stay centerX I forgot to add .
We pull to the experimental part , The above conjecture is verified .
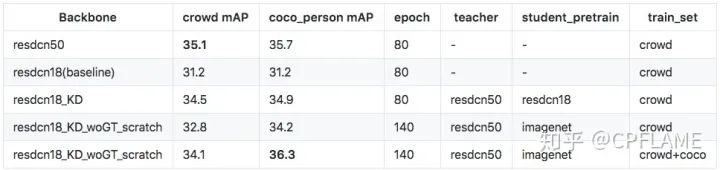
Multi model distillation
You can see the distillation effect , It can rise painlessly without increasing the amount of calculation , The writer was happy for a long time , Until the author encountered an embarrassing problem in the actual project scene :
I have a dataset A, There's something inside A The annotation
I have a dataset B, There's something inside B The annotation
Now, due to limited resources , Only one detection network can run , How do I get to be able to predict objects at the same time A And objects B The detector ?
Because the dataset A There may be a lot of unmarked B,B There will also be a lot of unmarked A, It's definitely not going to work together , The Internet will learn to be stupid .
The normal operation is to remove the dataset A Inside sign B, And then go to the dataset B Inside sign A, In this way, you can train on the data set added up . But tagging is expensive , What can I do ?
A little bit more coquettish is in A and B Train two networks , Then, the pseudo tags are predicted on the missing labeled dataset , And then train on the complete dataset
novelty The higher operation is to block the network output on the unlabeled dataset ,( This operation is only in C Two output detectors are available )
Is there a way , You don't need to label the data , It doesn't have to be as crude as a fake tag , Just lie flat , meanwhile novelty It's also higher , It's better to talk to the leaders KPI One way ?
After I patted my head again , I played my best skill : Whoring for nothing . Come up with such a plan :
I'll start with the data A Training a teacher model A, And then in the data B Training teacher model B, Then I put the teacher model A and B All the skills are passed on to the student model C, Beauty is not true ?
Let's see centernet The paradigm of , I've blown the author's work again , It's not just easy to understand the support centerPose,centertrack,center3Ddetection, Can also output rotatable object detection .
Similarly, , Maybe it's for reuse focal loss, The author used C A binary classifier , instead of softmax classification , This gives the author the inspiration of white whoring : Since it is C A binary classifier , So for each category , Then we can find a tutor for each student network , So you can have multiple happiness .
In theory, there can be many teachers , And each teacher can teach more than one category .
So our multi model distillation can be pieced together with the existing scheme . It's equivalent to that I paid my own code for nothing at the same time , And incomplete annotated datasets , White whoring really makes people happy . Compared with the above mentioned operations * a , As expected, the effect of multi model distillation is better . Another blind guess has been proved .
The author respectively in the human body and the car , And experiments on human and human faces . The data set is coco_car,crowd_human,widerface.
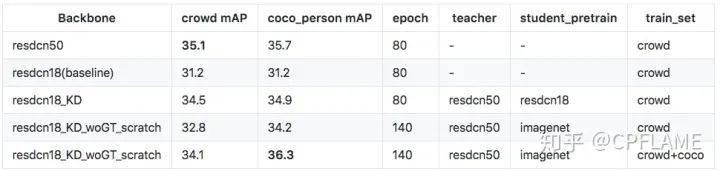
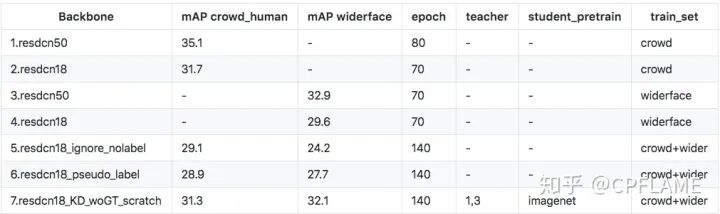
communism loss
The writer is training centerX when , There was such a problem , Set up appropriate lr when , Everything in training is so natural and harmonious , And when I lr When it's set big , Sometimes it's half done , Direct Internet loss Soar and mAP Return to zero and start climbing again , That led to the final model mAP It's very hip pulling . For this kind of situation, I have a bad temper .
After scolding, Shuang GUI Shuang , The problem is still to be solved , To solve this problem , The first thing I think about is where the author's code is bug, But I didn't find it after searching for a long time , The author also tried the following ways :
Join in clip gradients, No work
I added it myself skip loss, When this time iter Of loss It was last time loss Of k=1.1 More than times , This time, loss Set all 0, Don't update the network , No work
in lr_scheduler, in optimalizer, No work
Looks like this bug Oil and salt do not enter , Don't eat hard or soft . There will always be a certain period of time during training loss Suddenly it's growing , Then the network is trained from scratch .
This reminds me of the convolution acceleration , The capitalist bubble burst , After the great economic crisis, everything has been overturned and started again . Only at this time did I think of the good of communism , Chairman Mao is always a God .
In that case , Let's do it all the time , Give the cake directly to loss Let's divide , Let the light of the Communist proletariat shine on them , In a fit of anger, the writer put loss The size of each rabbit head We've made it a rule that , The specific operation is as follows :
For each loss Set a variable loss weight, Give Way loss Always at a fixed value .
Consider the fixed loss It's hard core , The author put lr Set to cosine Of lr, Give Way lr A more smooth descent , To simulate the gradient distribution learned by the network under normal conditions .
In fact, Ben loss You can change your name to adaptive loss, But in memory of this exasperation and sublimation of thinking , I still arbitrarily call it communism loss.
The next part of the experiment is to see if the tube doesn't work , So I tried the collapse of the previous lr, Benefit from the benefits of communism , Several datasets were changed, but the running experiment didn't appear mAP The situation of pulling the crotch , There were several times during this period loss Soaring situation , But in communism loss Under the powerful regulation ability, it quickly returned to normal state , It seems that socialism is indeed superior . At the same time, the author also tried to use the appropriate lr, run baseline And communism loss The experiment of , It is found that both of them are in the ±0.3 Of mAP about , The impact is not big .
The author has been happy for a long time , And found communism loss It can be used in distillation , And the performance is relatively stable , stay ±0.2 individual mAP about . This distillation can end2end Trained , No more human eyes to see loss、 count loss weight、 Stop training from scratch .
Model acceleration
This part of the code is in code Of projects/speedup in , Note that the network cannot contain DCN, Otherwise transcoding is very difficult .
centerX Provides a transition to caffe, turn onnx Code for ,onnx turn tensorRT As long as the environment is installed, a line of instructions can be converted , The author also provides forward code for different frameworks after transformation .
Among them, the author also found centernet Of tensorRT Forward version ( Later I call it centerRT), Use... In it cuda Yes centernet Post processing of ( Include 3X3 max pool and topK post-processing ). I'm finished tensorRT After that, I want to put centerRT Come here for nothing , It turns out there's still some trouble ,centerRT It's kind of like for centernet Original implementation customized to write . This leads to the following problems
Not only is tensorRT edition , I don't want to write troublesome post-processing on all frameworks , I want to write all the troublesome operations into the network , So I don't have to do anything , Just lie flat
stay centernet cls head Add another layer after the output of 3X3 Of max pooling, Can reduce a part of post-processing code
Is there any way to make the final central point head The output of satisfies the following conditions :1. Besides the central point , All the other pixel values are 0,( It's equivalent to having done it pseudo nms);2. Post processing only needs to be done here feature Up traversal >thresh The pixel position is OK .
If x1 Express centernet The central point output of ,x2 It means passing by 3X3 maxpool Output after , So in python In fact, you only need to write a line of code to get the above conditions :y = x1[x1==x2]. But when I use conversion ,onnx I won't support it == The operation of . We have to find another way .
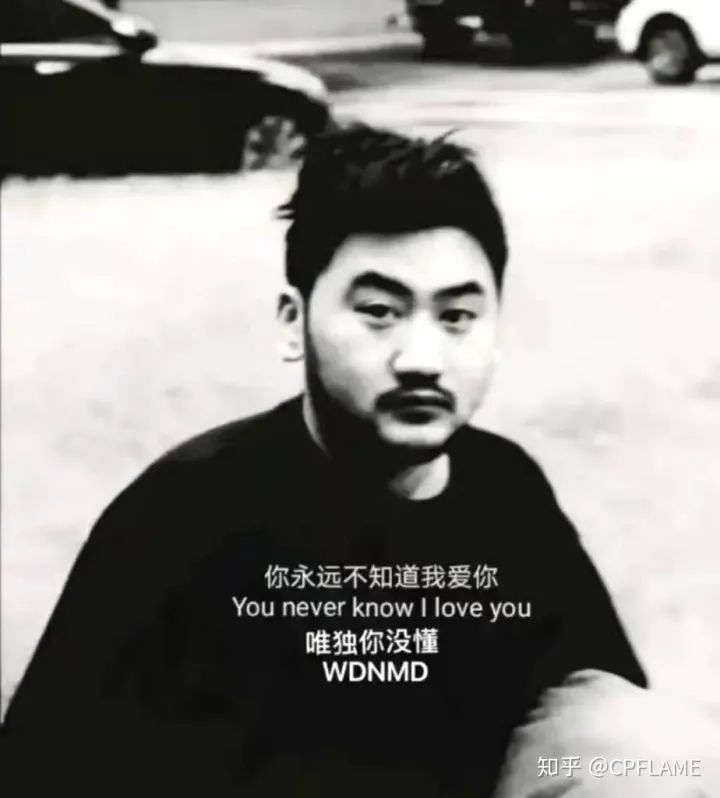
This time, I broke my head, but I didn't expect how to go whoring for nothing , So after sacrificing a few precious hairs , Forced to launch the throwing pot skill , Throw all post-processing operations to neural networks , The specific operation is as follows :
x2 yes x1 Of max pool, What we need x1[x1==x2] Of feature map
So we just need to get x1==x2, It's a binary picture mask, And then use mask*x1 That's all right. ,.
because x2 yes x1 Of max pool, therefore x1-x2 <= 0, We are x1-x2 Add a very small number to it , Make equal to 0 The number of pixels becomes positive , Less than 0 Is still negative . And then add a relu, Multiply by a coefficient so that the positive number is scaled to 1, We can get what we want .
The code is as follows :
def centerX_forward(self, x):
x = self.normalizer(x / 255.)
y = self._forward(x)
fmap_max = nn.MaxPool2d(kernel_size=3, stride=1, padding=1)(y['cls'])
keep = (y['cls'] - fmap_max).float() + 1e-9
keep = nn.ReLU()(keep)
keep = keep * 1e9
result = y['cls'] * keep
ret = [result,y['reg'],y['wh']] ## change dict to list
return ret
onnx The visualization is as follows :
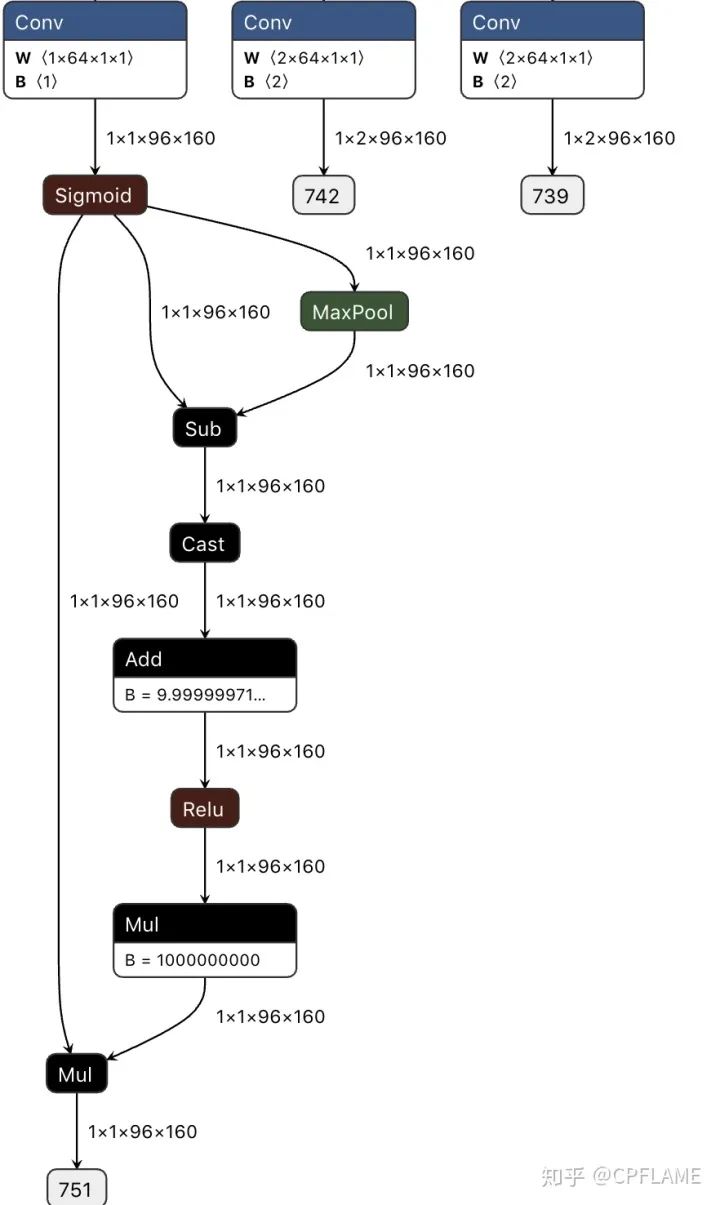
Then we can come to the post-processing code of Kangkang after the Sao operation , Extremely simple , I believe it can also be implemented quickly on any framework :
def postprocess(self, result, ratios, thresh=0.3):
clses, regs, whs = result
# clses: (b,c,h,w)
# regs: (b,2,h,w)
bboxes = []
for cls, reg, wh, ratio in zip(clses, regs, whs, ratios):
index = np.where(cls >= thresh)
ratio = 4 / ratio
score = np.array(cls[index])
cat = np.array(index[0])
ctx, cty = index[-1], index[-2]
w, h = wh[0, cty, ctx], wh[1, cty, ctx]
off_x, off_y = reg[0, cty, ctx], reg[1, cty, ctx]
ctx = np.array(ctx) + np.array(off_x)
cty = np.array(cty) + np.array(off_y)
x1, x2 = ctx - np.array(w) / 2, ctx + np.array(w) / 2
y1, y2 = cty - np.array(h) / 2, cty + np.array(h) / 2
x1, y1, x2, y2 = x1 * ratio, y1 * ratio, x2 * ratio, y2 * ratio
bbox = np.stack((cat, score, x1, y1, x2, y2), axis=1).tolist()
bbox = sorted(bbox, key=lambda x: x[1], reverse=True)
bboxes.append(bbox)
return bboxes
It is worth noting that the above operation is turning caffe I will make a mistake , So you can't add . If you have to add , You have to caffe Of prototxt Add your own scale layer ,elementwise layer ,relu layer , This author has not realized , If you are interested, you can add .
To optimize the direction
Considering that you need to manage upward , The author writes a few things that can rise
stay centernet The author originally issue Mentioned inside ,centernet It depends on the characteristics of the last layer of the network , So add dlaup It's going to go up a little bit obviously , But because of feature Of channel Too much , There will be some time lost . The author measured that in a certain backbone+deconv Add up dlaup after ,batchsize=8 By the time 32ms->44ms about , There are some costs , So I didn't add . The follow-up should be able to put dlaup All the convolutions in it are changed to depthwise Of , Find a balance between speed and precision
Think of a way to see if you can put Generalized Focal Loss,Giou loss Wait for plagiarism , Change it a little bit and add it to centernet Inside
Adjustable parameter ,lr,lossweight, Or communism loss It's fixed inside loss value , Different on different datasets backbone Can be optimized
With a cow pretrain model
Next door fast reid The automatic hyper parameter search of white whoring comes over
In addition to the above optimization in terms of accuracy , In fact, I also think of a lot of things that can be done , We don't play with others in precision , Because you can't roll anyone else , Testing this field is a real God fight , I can't beat you, but I can't . We want to make the cake bigger , We have meat to eat together
Distillation is not only applicable to centernet, The author raises another blind guess : be-all one-stage detector and anchor-free All the detectors can be distilled , And the last detector head cls All layers are changed to C individual 2 After classification , It should also be possible to achieve multi model distillation
centerPose, In fact, the original author's centerpose It's already done in a network , But I think we can make the best of it , Put it only in pose It was trained on the dataset simplebaseline Network distillation to centernet Go inside , The advantage of this is :1. The mark of detection and pose Can be separated from , Annotate as two separate datasets , In this way, there will be more data sets for free .2: And it will be faster in a network .
centerPoint, Directly output the offset of the four corners of the rectangular box relative to the center point , Not the width and height of the rectangle , In this case, the output of the detection is an arbitrary quadrilateral , The advantage is :1. We can add any rotational data enhancement while training without worrying about gt The problem with the label box getting bigger , At the same time, maybe we can use the existing detection data set + The network trained by rotation data enhancement has the ability to predict rotating objects .2. This network is detecting license plates , Or ID card and invoice have natural advantages , Direct prediction of four corners , You don't have to do any affine transformations , It doesn't have to be replaced by a bulky, segmented network .
Conclusion
In fact, there are too many things to add to centerX It went to the , There are many interesting and practical things to do , But personal energy is limited , And just started to do centerX It's all about hobbies , I'm just a slag , unable full time Jump on this thing , So let's see if I can do it in my lifetime , It's also very good if you can't do it to provide you with a feasible idea .
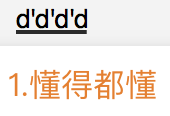
Thank you very much , He Lingxiao is right about centerX Code , And the contribution in the direction of development , Thank you Guo Cong , Yu Wanjin , Jiang Yuxiang , Zhang Jianhao and other students were right about centerX Accelerate the pit guidance of the module .
Play your own again github
https://github.com/CPFLAME/centerXgithub.com
And thanks for the following outstanding work
xingyizhou/CenterNetgithub.comfacebookresearch/detectron2github.com
https://github.com/FateScript/CenterNet-better
lbin/CenterNet-better-plus
JDAI-CV/fast-reid
https://github.com/daquexian/onnx-simplifier
https://github.com/CaoWGG/TensorRT-CenterNet
This article is only for academic sharing , If there is any infringement , Please contact to delete .
Dry goods download and learning
The background to reply : Barcelo that Autonomous University courseware , You can download the precipitation of foreign universities for several years 3D Vison High quality courseware
The background to reply : Computer vision Books , You can download it. 3D Classic books in the field of vision pdf
The background to reply :3D Visual courses , You can learn 3D Excellent courses in the field of vision
Computer vision workshop boutique course official website :3dcver.com
1. Multi sensor data fusion technology for automatic driving field
2. For the field of automatic driving 3D Whole stack learning route of point cloud target detection !( Single mode + Multimodal / data + Code )
3. Thoroughly understand the visual three-dimensional reconstruction : Principle analysis 、 Code explanation 、 Optimization and improvement
4. China's first point cloud processing course for industrial practice
5. laser - Vision -IMU-GPS The fusion SLAM Algorithm sorting and code explanation
6. Thoroughly understand the vision - inertia SLAM: be based on VINS-Fusion The class officially started
7. Thoroughly understand based on LOAM Framework of the 3D laser SLAM: Source code analysis to algorithm optimization
8. Thorough analysis of indoor 、 Outdoor laser SLAM Key algorithm principle 、 Code and actual combat (cartographer+LOAM +LIO-SAM)
10. Monocular depth estimation method : Algorithm sorting and code implementation
11. Deployment of deep learning model in autopilot
12. Camera model and calibration ( Monocular + Binocular + fisheye )
13. blockbuster ! Four rotor aircraft : Algorithm and practice
14.ROS2 From entry to mastery : Theory and practice
15. The first one in China 3D Defect detection tutorial : theory 、 Source code and actual combat
16. be based on Open3D Introduction and practical tutorial of point cloud processing
blockbuster ! Computer vision workshop - Study Communication group Established
Scan the code to add a little assistant wechat , You can apply to join 3D Visual workshop - Academic paper writing and contribution WeChat ac group , Aimed at Communication Summit 、 Top issue 、SCI、EI And so on .
meanwhile You can also apply to join our subdivided direction communication group , At present, there are mainly ORB-SLAM Series source code learning 、3D Vision 、CV& Deep learning 、SLAM、 Three dimensional reconstruction 、 Point cloud post processing 、 Autopilot 、CV introduction 、 Three dimensional measurement 、VR/AR、3D Face recognition 、 Medical imaging 、 defect detection 、 Pedestrian recognition 、 Target tracking 、 Visual products landing 、 The visual contest 、 License plate recognition 、 Hardware selection 、 Depth estimation 、 Academic exchange 、 Job exchange Wait for wechat group , Please scan the following micro signal clustering , remarks :” Research direction + School / company + nickname “, for example :”3D Vision + Shanghai Jiaotong University + quietly “. Please note... According to the format , Otherwise, it will not pass . After successful addition, relevant wechat groups will be invited according to the research direction . Original contribution Please also contact .
▲ Long press and add wechat group or contribute
▲ The official account of long click attention
3D Vision goes from entry to mastery of knowledge : in the light of 3D In the field of vision Video Course cheng ( 3D reconstruction series 、 3D point cloud series 、 Structured light series 、 Hand eye calibration 、 Camera calibration 、 laser / Vision SLAM、 Automatically Driving, etc )、 Summary of knowledge points 、 Introduction advanced learning route 、 newest paper Share 、 Question answer Carry out deep cultivation in five aspects , There are also algorithm engineers from various large factories to provide technical guidance . meanwhile , The planet will be jointly released by well-known enterprises 3D Vision related algorithm development positions and project docking information , Create a set of technology and employment as one of the iron fans gathering area , near 4000 Planet members create better AI The world is making progress together , Knowledge planet portal :
Study 3D Visual core technology , Scan to see the introduction ,3 Unconditional refund within days
There are high quality tutorial materials in the circle 、 Answer questions and solve doubts 、 Help you solve problems efficiently
Feel useful , Please give me a compliment ~
边栏推荐
- Redis getting started complete tutorial: common exceptions on the client
- Huitong programming introductory course - 2A breakthrough
- How to find file accessed / created just feed minutes ago
- Le tube MOS réalise le circuit de commutation automatique de l'alimentation principale et de l'alimentation auxiliaire, et la chute de tension "zéro", courant statique 20ua
- c语言(字符串)如何把字符串中某个指定的字符删除?
- Kysl Haikang camera 8247 H9 ISAPI test
- widerperson数据集转化为YOLO格式
- C language string sorting
- Data analysis from the perspective of control theory
- Introduction to ins/gps integrated navigation type
猜你喜欢
又一百万量子比特!以色列光量子初创公司完成1500万美元融资
Oauth2协议中如何对accessToken进行校验
Construction of knowledge map of mall commodities
“零售为王”下的家电产业:什么是行业共识?
NuScenes数据集关于Radar数据的统计
The annual salary of general test is 15W, and the annual salary of test and development is 30w+. What is the difference between the two?
尚硅谷JVM-第一章 类加载子系统
商城商品的知识图谱构建
Have fun | latest progress of "spacecraft program" activities
tensorboard的使用
随机推荐
Simple bubble sort
LeetCode 77:组合
2022年信息安全工程师考试大纲
Have fun | latest progress of "spacecraft program" activities
MySQL is an optimization artifact to improve the efficiency of massive data query
Use of promise in ES6
Cryptography series: detailed explanation of online certificate status protocol OCSP
Form validation of uniapp
Utilisation de la promesse dans es6
mos管实现主副电源自动切换电路,并且“零”压降,静态电流20uA
How does C language (string) delete a specified character in a string?
c语言(字符串)如何把字符串中某个指定的字符删除?
The so-called consumer Internet only matches and connects industry information, and does not change the industry itself
Unity使用MaskableGraphic画一条带箭头的线
MySQL
PSINS中19维组合导航模块sinsgps详解(初始赋值部分)
密码学系列之:在线证书状态协议OCSP详解
尚硅谷JVM-第一章 类加载子系统
Oracle中日期的使用方法实例
巴比特 | 元宇宙每日必读:IP授权是NFT的破圈之路吗?它的难点在哪里?Holder该如何选择合作平台?...