当前位置:网站首页>AI super clear repair resurfaces the light in Huang Jiaju's eyes, Lecun boss's "deep learning" course survival report, beautiful paintings only need one line of code, AI's latest paper | showmeai info
AI super clear repair resurfaces the light in Huang Jiaju's eyes, Lecun boss's "deep learning" course survival report, beautiful paintings only need one line of code, AI's latest paper | showmeai info
2022-07-07 00:33:00 【ShowMeAI】
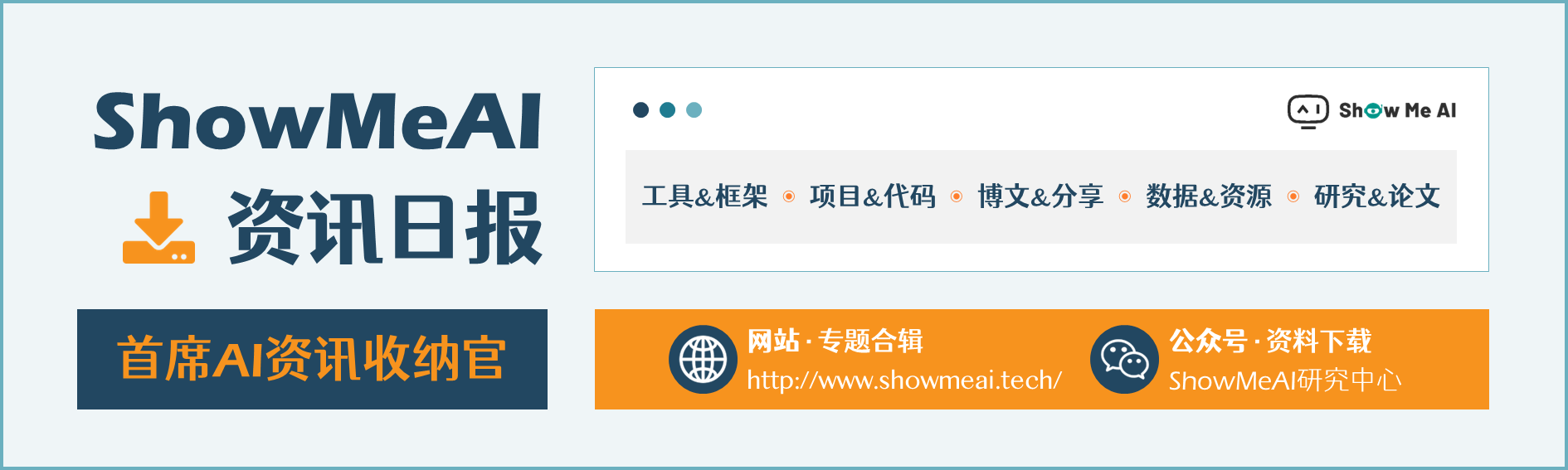
ShowMeAI daily Series new upgrade ! Cover AI Artificial intelligence Tools & frame | project & Code | post & Share | data & resources | Research & The paper Wait for the direction . Click to see List of historical articles , Subscribe to topics in the official account #ShowMeAI Information daily , Can receive the latest daily push . Click on Thematic collection & Electronic monthly Quickly browse the complete works of each topic . Click on here Reply key daily Free access AI Electronic monthly and information package .
AI Repair huangjiaju 31 Classic pictures years ago
7 month 3 Friday night , utilize AI Technology repair Beyond『1991 Life contact concert 』, Time interval 31 It was re shown on Tiktok platform in . This super clear repair remake , Insufficient resolution 540p To be close to 4K, The skin 、 The smile and other details are all clearly visible .
![]() ![]() |
Tools & frame

【DiscoArt】 Use one line of code Disco Diffusion Generate artwork
GitHub: https://github.com/jina-ai/discoart
Disco Diffusion Is a Google Colab Notebook Free tools , Use Python Language creates beautiful images and videos from text .DiscoArt Simplify the process , Make the results reproducible 、 persistence 、gRPC/HTTP service 、 Later analysis is more concise , Help artists and AI Lovers create more gracefully .
![]() ![]() |
【Versatile Data Kit (VDK) 】 Use SQL or Python Knowledge builds data pipeline
GitHub: https://github.com/vmware/versatile-data-kit
VDK( Multifunctional data toolkit ) It's an open source framework , from Data SDK and Control Service Two kits . Data engineers can use both SQL or Python Language , Development 、 Deploy 、 Run and manage data jobs . The following figure shows the data flow and VDK The role of .
![]() |
【The Nettle Magic Project】 Poker barcode scanner , Determine the position of each card by scanning the QR code composed of the side of the poker
GitHub: https://github.com/nettlep/magichttps://github.com/nettlep/magic
Find ink that can only be recognized under specific infrared conditions , And mark the edge of a deck of playing cards . Use a NoIR Raspberry pie for camera Zero W These markers can be detected , coordination iPad Running on iOS Client applications , You can clearly identify playing cards and infrared marks .
![]() ![]() ![]() |
【wenet_trt8】 use TRT8 Deploy the open source speech recognition toolkit
GitHub: https://github.com/huismiling/wenet_trt8
WeNet It is a speech recognition toolkit for industrial applications , Model from encoder and decoder Two parts . This project uses FP32 Model (Pytorch Directly derived ), Use ORT(ONNX Run Time) and PPQ Two quantitative methods are right WeNet The model is quantified .
- Use ORT quantitative : Model test results WER:6.06%, Time consuming :Encoder 7.34ms,Decoder 3.32ms. Data set effect decreases 1.46%, and Encoder Speed up 2.5 times ,Decoder Speed up 6.3 times .
- Use PPQ quantitative : Model test results WER:5.71%, Time consuming :Encoder 12.14ms,Decoder 2.68ms. Data set effect decreases 1.11%, and Encoder Speed up 1.5 times ,Decoder Speed up 7.7 times .
![]() ![]() |
post & Share

New York University 《 Deep learning (2021) 》 Course summary and study notes
note : https://dimgeo.com/posts/NYU-2021-DL-course-overview-and-notes/
Course : https://atcold.github.io/NYU-DLSP21/
NYU 2021《DEEP LEARNING》 Lecturer of , One is famous Yann LeCun, The other is an assistant professor in the Department of computer science at New York University Alfredo. Course about 50 Hours , Information is very dense 、 It covers a wide range of 、 And it includes Facebook AI The guest lecturer of the research laboratory showed CV and NLP The latest development of the industry .
however ! Be prepared !LeCun Our courses are not like Andrew NG Wu Enda is so friendly , And you need to have basic machine learning knowledge and linear algebra knowledge .
![]() ![]() |
《 Markov chain for programmers 》 Free ebooks
Github: https://github.com/czekster/markov
pdf: https://czekster.github.io/markov/MC-for-programmers2022.pdf
Author of this book Ricardo M. Czekster, Explain Markov chain and its basic solution , contain Markov Chains、DTMC、CTMC Other chapters ( See the picture below for the table of contents ). Can be in GitHub Find the basic materials of this book on the page , for example C Programming code and solutions 、MATLAB Script 、 This book provides examples of prismatic models (CTMC/DTMC) etc. .
![]() ![]() ![]() |
2022 China's future Unicorn TOP100 The list
At the 6th conference on the growth of all things · China future Unicorn Conference ,《2022 China's future Unicorn TOP100 The list 》 Official release , Focus on enterprise services 、 Artificial intelligence 、 The new consumer 、 Advanced manufacturing 、 Meta universe 5 Large area , And selected in various fields 20 High quality enterprises . The list is here ! You my AI Little cute people reference ~
![]() ![]() ![]() ![]() ![]() |
data & resources

University of Geneva, Switzerland 《 Deep learning 》 Course videos and materials
Address : https://fleuret.org/dlc/
UNIGE 14x050《Deep Learning》 The course provides a comprehensive introduction to in-depth learning , With PyTorch Examples in the framework :
- Machine learning goals and major challenges
- Tensor operation
- Automatic differentiation , gradient descent
- Deep learning of specific technologies
- Generated 、 Cyclic 、 Attention model
![]() ![]() |
Super resolution / hallucination Related work list
GitHub: https://github.com/junjun-jiang/Face-Hallucination-Benchmark
Face-Hallucination-Benchmark:A collection of state-of-the-art face super-resolution/hallucination methods by Junjun Jiang
![]() ![]() |
Research & The paper

You can click on the here Reply key daily , Get the collected papers for free .
Official account reply daily , Get the collected papers for free .
Research progress
2022 year 6 month 27 Japan 【 Deep learning 】| Benchopt: Reproducible, efficient and collaborative optimization benchmarks
ICLR 2020【 Figure neural network 】| Measuring and Improving the Use of Graph Information in Graph Neural Networks
2022 year 6 month 27 Japan 【 natural language processing 】| Center-Embedding and Constituency in the Brain and a New Characterization of Context-Free Languages
2022 year 6 month 27 Japan 【 natural language processing 】| Endowing Language Models with Multimodal Knowledge Graph Representations
2022 year 6 month 27 Japan 【 machine learning 】| Fast sequence to graph alignment using the graph wavefront algorithm
2022 year 6 month 27 Japan 【 Computer vision 】| CLTS-GAN: Color-Lighting-Texture-Specular Reflection Augmentation for Colonoscopy
The paper :Benchopt: Reproducible, efficient and collaborative optimization benchmarks
Paper title :Benchopt: Reproducible, efficient and collaborative optimization benchmarks
Paper time :27 Jun 2022
Field : Deep learning
Corresponding tasks :Image Classification,Stochastic Optimization, Image classification , Stochastic optimization
Address of thesis :https://arxiv.org/abs/2206.13424
Code implementation :https://github.com/benchopt/benchopt
Author of the paper :Thomas Moreau, Mathurin Massias, Alexandre Gramfort, Pierre Ablin, Pierre-Antoine Bannier, Benjamin Charlier, Mathieu Dagréou, Tom Dupré La Tour, Ghislain Durif, Cassio F. Dantas, Quentin Klopfenstein, Johan Larsson, En Lai, Tanguy Lefort, Benoit Malézieux, Badr Moufad, Binh T. Nguyen, Alain Rakotomamonjy, Zaccharie Ramzi, Joseph Salmon, Samuel Vaiter
Brief introduction of the paper :Numerical validation is at the core of machine learning research as it allows to assess the actual impact of new methods, and to confirm the agreement between theory and practice./ Numerical verification is the core of machine learning research , Because it can evaluate the actual impact of the new method , And confirm the consistency between theory and practice .
Abstract of paper :Numerical validation is at the core of machine learning research as it allows to assess the actual impact of new methods, and to confirm the agreement between theory and practice. Yet, the rapid development of the field poses several challenges: researchers are confronted with a profusion of methods to compare, limited transparency and consensus on best practices, as well as tedious re-implementation work. As a result, validation is often very partial, which can lead to wrong conclusions that slow down the progress of research. We propose Benchopt, a collaborative framework to automate, reproduce and publish optimization benchmarks in machine learning across programming languages and hardware architectures. Benchopt simplifies benchmarking for the community by providing an off-the-shelf tool for running, sharing and extending experiments. To demonstrate its broad usability, we showcase benchmarks on three standard learning tasks: ℓ2-regularized logistic regression, Lasso, and ResNet18 training for image classification. These benchmarks highlight key practical findings that give a more nuanced view of the state-of-the-art for these problems, showing that for practical evaluation, the devil is in the details. We hope that Benchopt will foster collaborative work in the community hence improving the reproducibility of research findings.
Numerical verification is the core of machine learning research , Because it can evaluate the actual impact of the new method , And confirm the consistency between theory and practice . However , The rapid development of this field has brought some challenges : Researchers face a large number of methods that need to be compared 、 Limited transparency and consensus on best practices , And tedious re implementation . therefore , Verification is often very one-sided , This may lead to wrong conclusions , Slow down the progress of research . We proposed Benchopt, This is a cooperation framework , For Automation 、 Replicate and publish optimization benchmarks for machine learning across programming languages and hardware architectures .Benchopt By providing an off the shelf tool to run 、 Share and expand experiments , This simplifies the benchmarking of the community . To prove its wide availability , We show benchmarks for three standard learning tasks :ℓ2-regularized logistic regression, Lasso, and ResNet18 Image classification training . These benchmarks highlight key practical findings , Give a more detailed view of the most advanced level of these issues , It shows that for the actual evaluation , The key lies in the details . We hope Benchopt It can promote community cooperation , So as to improve the repeatability of the research results .
![]() ![]() |
The paper :Measuring and Improving the Use of Graph Information in Graph Neural Networks
Paper title :Measuring and Improving the Use of Graph Information in Graph Neural Networks
Paper time :ICLR 2020
Field : Figure neural network
Corresponding tasks :Representation Learning, Representational learning
Address of thesis :https://arxiv.org/abs/2206.13170
Code implementation :https://github.com/yifan-h/CS-GNN
Author of the paper :Yifan Hou, Jian Zhang, James Cheng, Kaili Ma, Richard T. B. Ma, Hongzhi Chen, Ming-Chang Yang
Brief introduction of the paper :Graph neural networks (GNNs) have been widely used for representation learning on graph data./ Figure neural network (GNNs) It has been widely used in graph data representation learning .
Abstract of paper :Graph neural networks (GNNs) have been widely used for representation learning on graph data. However, there is limited understanding on how much performance GNNs actually gain from graph data. This paper introduces a context-surrounding GNN framework and proposes two smoothness metrics to measure the quantity and quality of information obtained from graph data. A new GNN model, called CS-GNN, is then designed to improve the use of graph information based on the smoothness values of a graph. CS-GNN is shown to achieve better performance than existing methods in different types of real graphs.
Figure neural network (GNNs) It has been widely used in graph data representation learning . However , People are right. GNNs There is limited understanding of how much performance is actually obtained from the graph data . This paper introduces a context based GNN frame , Two smoothness indexes are proposed to measure the quantity and quality of information obtained from graph data . Then I designed a new GNN Model , be called CS-GNN, To improve the use of graph information based on graph smoothness values .CS-GNN It has been proved that it has better performance than the existing methods in different types of real graphs .
![]() ![]() |
The paper :Center-Embedding and Constituency in the Brain and a New Characterization of Context-Free Languages
Paper title :Center-Embedding and Constituency in the Brain and a New Characterization of Context-Free Languages
Paper time :27 Jun 2022
Field : natural language processing
Corresponding tasks :Dependency Parsing, Dependency resolution
Address of thesis :https://arxiv.org/abs/2206.13217
Code implementation :https://github.com/dmitropolsky/assemblies
Author of the paper :Daniel Mitropolsky, Adiba Ejaz, Mirah Shi, Mihalis Yannakakis, Christos H. Papadimitriou
Brief introduction of the paper :We address two of the most important questions left open by that work: constituency (the identification of key parts of the sentence such as the verb phrase) and the processing of dependent sentences, especially center-embedded ones./ We discussed the two most important issues left by this work : Word formation ( Identify the key parts of the sentence , Such as verb phrases ) And subordinate sentence processing , Especially the sentence embedded in the center .
Abstract of paper :A computational system implemented exclusively through the spiking of neurons was recently shown capable of syntax, that is, of carrying out the dependency parsing of simple English sentences. We address two of the most important questions left open by that work: constituency (the identification of key parts of the sentence such as the verb phrase) and the processing of dependent sentences, especially center-embedded ones. We show that these two aspects of language can also be implemented by neurons and synapses in a way that is compatible with what is known, or widely believed, about the structure and function of the language organ. Surprisingly, the way we implement center embedding points to a new characterization of context-free languages.
lately , A computing system implemented entirely by neurons has been proved to be able to perform syntax , That is, dependency analysis of simple English sentences . We discussed the two most important issues left by this work : Word formation ( Identify the key parts of the sentence , Such as verb phrases ) And subordinate sentence processing , Especially the sentence embedded in the center . We show that , These two aspects of language can also be realized by neurons and synapses , Its way is consistent with the known or widely recognized structure and function of language organs . It's amazing , The way we implement central embedding points to the new features of contextless language .
![]() |
The paper :Endowing Language Models with Multimodal Knowledge Graph Representations
Paper title :Endowing Language Models with Multimodal Knowledge Graph Representations
Paper time :27 Jun 2022
Field : natural language processing
Corresponding tasks :Multilingual Named Entity Recognition,named-entity-recognition,+1
Address of thesis :https://arxiv.org/abs/2206.13163
Code implementation :https://github.com/iacercalixto/visualsem
Author of the paper :Ningyuan Huang, Yash R. Deshpande, Yibo Liu, Houda Alberts, Kyunghyun Cho, Clara Vania, Iacer Calixto
Brief introduction of the paper :We use the recently released VisualSem KG as our external knowledge repository, which covers a subset of Wikipedia and WordNet entities, and compare a mix of tuple-based and graph-based algorithms to learn entity and relation representations that are grounded on the KG multimodal information./ We use the recently released VisualSem KG As our external knowledge base , It covers Wikipedia and WordNet A subset of entities , And compares the hybrid algorithm based on tuple and graph to learn based on KG Entity and relationship representation of multimodal information .
Abstract of paper :We propose a method to make natural language understanding models more parameter efficient by storing knowledge in an external knowledge graph (KG) and retrieving from this KG using a dense index. Given (possibly multilingual) downstream task data, e.g., sentences in German, we retrieve entities from the KG and use their multimodal representations to improve downstream task performance. We use the recently released VisualSem KG as our external knowledge repository, which covers a subset of Wikipedia and WordNet entities, and compare a mix of tuple-based and graph-based algorithms to learn entity and relation representations that are grounded on the KG multimodal information. We demonstrate the usefulness of the learned entity representations on two downstream tasks, and show improved performance on the multilingual named entity recognition task by 0.3%–0.7% F1, while we achieve up to 2.5% improvement in accuracy on the visual sense disambiguation task. All our code and data are available in https://github.com/iacercalixto/visualsem-kg
We propose a way , Through the external knowledge map (KG) Store knowledge in and use dense indexes from this KG To retrieve the , Make the parameter efficiency of natural language understanding model higher . Given the downstream task data ( It may be multilingual ), For example, German sentences , We from KG Retrieve entities in , And use their multimodal representations to improve the performance of downstream tasks . We use the recently released VisualSem KG As our external knowledge base , It covers Wikipedia and WordNet A subset of entities , And compares the hybrid algorithm based on tuple and graph to learn based on KG Entity and relationship representation of multimodal information . We proved the usefulness of the learned entity representation in two downstream tasks , It also shows that the performance in multilingual named entity recognition task is improved 0.3%-0.7% F1, In the task of ambiguity in the visual sense , We achieved up to 2.5% Improve the accuracy of . All our codes and data can be found on the following website https://github.com/iacercalixto/visualsem-kg
![]() ![]() |
The paper :Fast sequence to graph alignment using the graph wavefront algorithm
Paper title :Fast sequence to graph alignment using the graph wavefront algorithm
Paper time :27 Jun 2022
Field : machine learning
Address of thesis :https://arxiv.org/abs/2206.13574
Code implementation :https://github.com/lh3/gwfa
Author of the paper :Haowen Zhang, Shiqi Wu, Srinivas Aluru, Heng Li
Brief introduction of the paper :Motivation: A pan-genome graph represents a collection of genomes and encodes sequence variations between them./ A pan genome map represents a collection of genomes , And encode the sequence changes between them .
Abstract of paper :Motivation: A pan-genome graph represents a collection of genomes and encodes sequence variations between them. It is a powerful data structure for studying multiple similar genomes. Sequence-to-graph alignment is an essential step for the construction and the analysis of pan-genome graphs. However, existing algorithms incur runtime proportional to the product of sequence length and graph size, making them inefficient for aligning long sequences against large graphs. Results: We propose the graph wavefront alignment algorithm (Gwfa), a new method for aligning a sequence to a sequence graph. Although the worst-case time complexity of Gwfa is the same as the existing algorithms, it is designed to run faster for closely matching sequences, and its runtime in practice often increases only moderately with the edit distance of the optimal alignment. On four real datasets, Gwfa is up to four orders of magnitude faster than other exact sequence-to-graph alignment algorithms. We also propose a graph pruning heuristic on top of Gwfa, which can achieve an additional ∼10-fold speedup on large graphs. Availability: Gwfa code is accessible at https://github.com/lh3/gwfa .
The pan genome map represents a collection of genomes , And encode the sequence changes between them . It is a powerful data structure , Used to study multiple similar genomes . Sequence and map alignment is an important step in the construction and analysis of Pan genome map . However , The running time of existing algorithms is proportional to the product of sequence length and graph size , It makes them inefficient in long sequence alignment of large graphs . result . We propose a graphic wavefront alignment algorithm (Gwfa), This is a new method of aligning sequences with sequence diagrams . Even though Gwfa The worst-case time complexity of the algorithm is the same as that of the existing algorithm , But it is designed to run faster against closely matched sequences , And its running time in practice often only increases moderately with the editing distance of the best alignment . On four real datasets ,Gwfa It is four orders of magnitude faster than other accurate sequence to graph arrangement algorithms . We also Gwfa A heuristic graph pruning algorithm is proposed based on , It can realize additional ∼10 Double the speed .Gwfa The code can be found in https://github.com/lh3/gwfa obtain .
![]() |
The paper :CLTS-GAN: Color-Lighting-Texture-Specular Reflection Augmentation for Colonoscopy
Paper title :CLTS-GAN: Color-Lighting-Texture-Specular Reflection Augmentation for Colonoscopy
Paper time :29 Jun 2022
Field : Computer vision
Address of thesis :https://arxiv.org/abs/2206.14951
Code implementation :https://github.com/nadeemlab/CEP
Author of the paper :Shawn Mathew, Saad Nadeem, Arie Kaufman
Brief introduction of the paper :Automated analysis of optical colonoscopy (OC) video frames (to assist endoscopists during OC) is challenging due to variations in color, lighting, texture, and specular reflections./ Because of the color 、 lighting 、 Changes in texture and specular reflection , Optical colonoscopy (OC) Automatic analysis of video frames ( stay OC During this period, assist the endoscopist ) Challenging .
Abstract of paper :Automated analysis of optical colonoscopy (OC) video frames (to assist endoscopists during OC) is challenging due to variations in color, lighting, texture, and specular reflections. Previous methods either remove some of these variations via preprocessing (making pipelines cumbersome) or add diverse training data with annotations (but expensive and time-consuming). We present CLTS-GAN, a new deep learning model that gives fine control over color, lighting, texture, and specular reflection synthesis for OC video frames. We show that adding these colonoscopy-specific augmentations to the training data can improve state-of-the-art polyp detection/segmentation methods as well as drive next generation of OC simulators for training medical students. The code and pre-trained models for CLTS-GAN are available on Computational Endoscopy Platform GitHub https://github.com/nadeemlab/CEP .
Because of the color 、 lighting 、 Changes in texture and specular reflection , Optical colonoscopy (OC) Automatic analysis of video frames ( stay OC During this period, assist the endoscope doctor ) Challenging . Previous methods either removed some of these changes through pretreatment ( Make the processing line cumbersome ), Or add different training data with annotations ( But expensive and time-consuming ). We proposed CLTS-GAN, A new deep learning model , Yes OC Color of video frame 、 lighting 、 Fine control of texture and specular synthesis . We show that , Add these colon specific enhancements to the training data , It can improve the most advanced polyp detection / Segmentation method , And promote the next generation colonoscopy simulator for training medical students .CLTS-GAN The code and pre training model are available on the computational endoscope platform GitHub https://github.com/nadeemlab/CEP Get on .
![]() |
We are ShowMeAI, Dedicated to communication AI High quality content , Share industry solutions , Accelerate every technological growth with knowledge ! Click to see List of historical articles , Subscribe to topics in the official account #ShowMeAI Information daily , Can receive the latest daily push . Click on Thematic collection & Electronic monthly Quickly browse the complete works of each topic . Click on here Reply key daily Free access AI Electronic monthly and information package .

- author : Han Xinzi @ShowMeAI
- List of historical articles
- Thematic collection & Electronic monthly
- Statement : copyright , For reprint, please contact the platform and the author and indicate the source
- Welcome to reply , Please praise , Leave a message to recommend valuable articles 、 Tools or suggestions , We will reply as soon as possible ~
边栏推荐
- 华为mate8电池价格_华为mate8换电池后充电巨慢
- Command line kills window process
- AI超清修复出黄家驹眼里的光、LeCun大佬《深度学习》课程生还报告、绝美画作只需一行代码、AI最新论文 | ShowMeAI资讯日报 #07.06
- Jenkins' user credentials plug-in installation
- kubernetes部署ldap
- Common shortcuts to idea
- The programmer resigned and was sentenced to 10 months for deleting the code. Jingdong came home and said that it took 30000 to restore the database. Netizen: This is really a revenge
- Racher integrates LDAP to realize unified account login
- Data analysis course notes (V) common statistical methods, data and spelling, index and composite index
- Typescript incremental compilation
猜你喜欢
How can computers ensure data security in the quantum era? The United States announced four alternative encryption algorithms
1000 words selected - interface test basis
2022/2/12 summary
Uniapp uploads and displays avatars locally, and converts avatars into Base64 format and stores them in MySQL database
DAY FOUR
Introduction au GPIO
[boutique] Pinia Persistence Based on the plug-in Pinia plugin persist
What can the interactive slide screen demonstration bring to the enterprise exhibition hall
三维扫描体数据的VTK体绘制程序设计
What is AVL tree?
随机推荐
从外企离开,我才知道什么叫尊重跟合规…
Pdf document signature Guide
DAY TWO
St table
Random类的那些事
C language input / output stream and file operation [II]
Business process testing based on functional testing
DAY SIX
工程师如何对待开源 --- 一个老工程师的肺腑之言
[daily problem insight] prefix and -- count the number of fertile pyramids in the farm
Leecode brush questions record interview questions 32 - I. print binary tree from top to bottom
DAY ONE
《LaTex》LaTex数学公式简介「建议收藏」
Operation test of function test basis
Use type aliases in typescript
【vulnhub】presidential1
openresty ngx_ Lua subrequest
Typescript incremental compilation
Compilation of kickstart file
Sword finger offer 26 Substructure of tree