当前位置:网站首页>BN folding and its quantification
BN folding and its quantification
2022-07-06 08:56:00 【cyz0202】
This paper introduces the process of quantification BN Fold ;
The following pictures are quoted from Quantization and Training of Neural Networks for Efficient Integer-Arithmetic-Only Inference
BN Fold
First introduced BN Fold ;BN Folding comes from BN The implementation difference between the training phase and the inference phase of the module ;
As shown in the figure below : With conv+BN For example , chart 1 For the training stage BN, chart 2 For the inferential stage BN
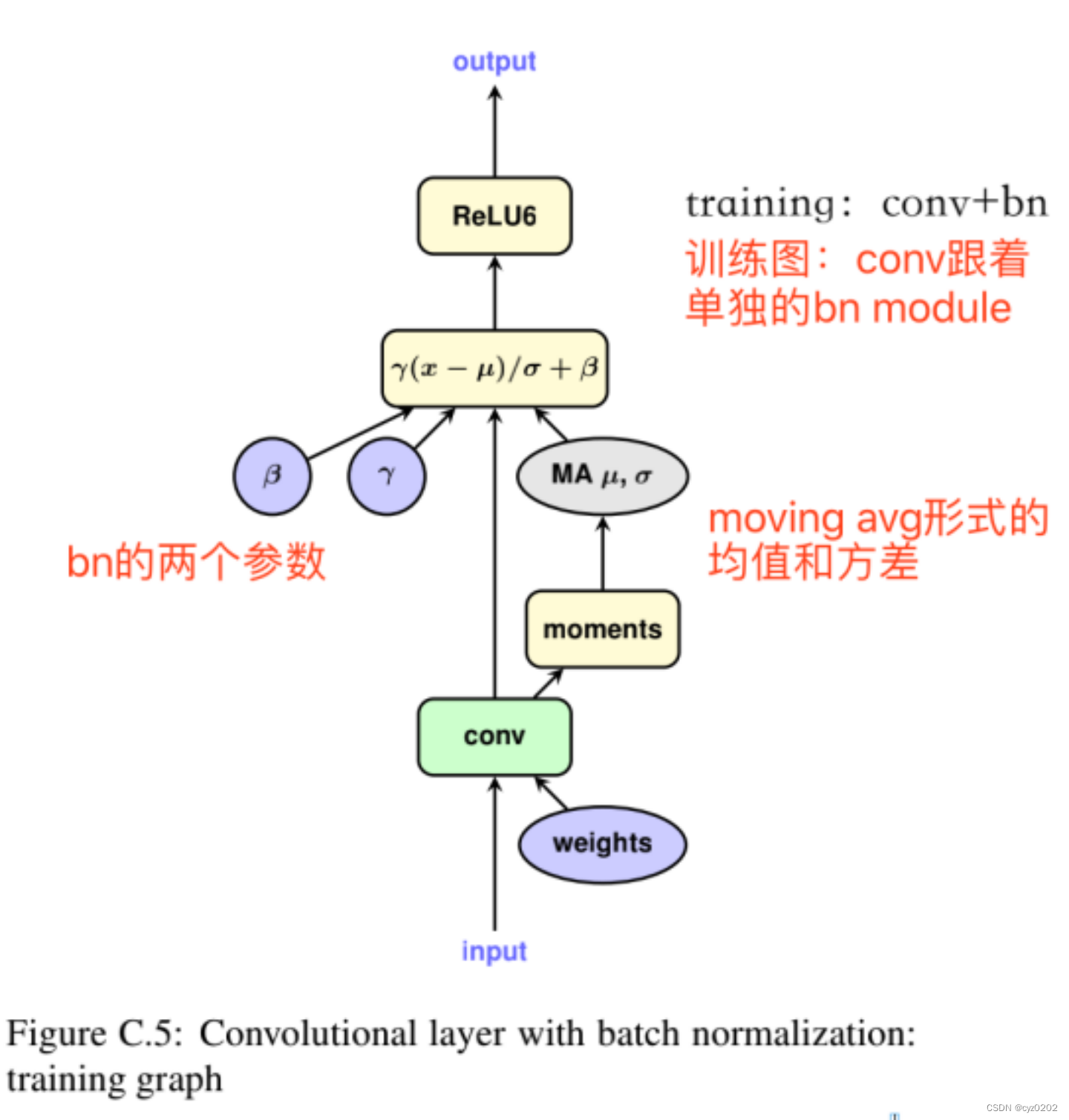
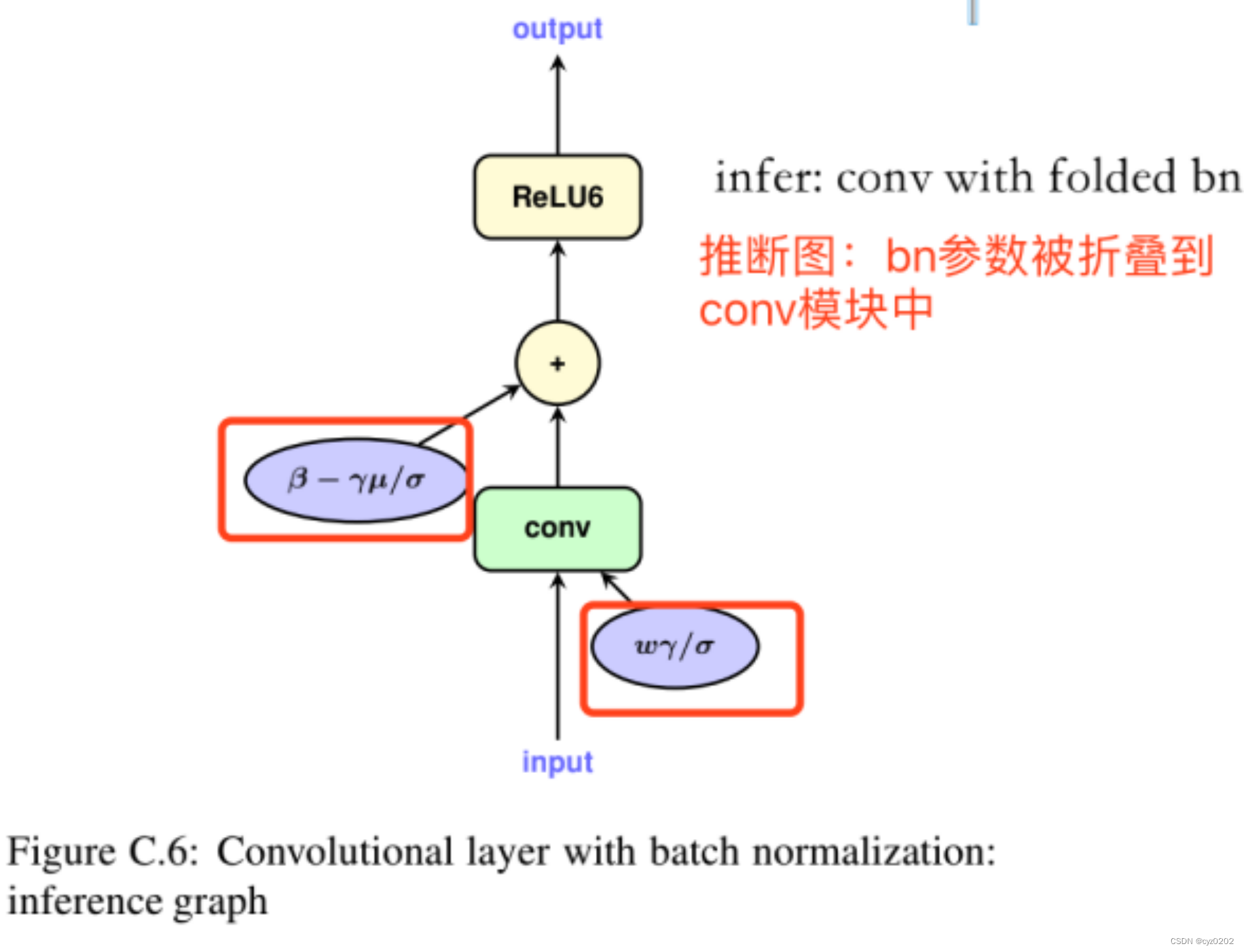
It can be proved that such folding is reasonable , Pay attention to the picture 2 Medium w It refers to the convolution kernel ;
In quantification BN Fold
For quantification , Especially quantitative perception ( Pseudo quantization ), We need to keep the pseudo quantization in the training stage and the above figure 2 The quantitative implementation of the inference phase of is consistent , Therefore, for the training stage CONV+BN Fold in two steps , Here's the picture 3:
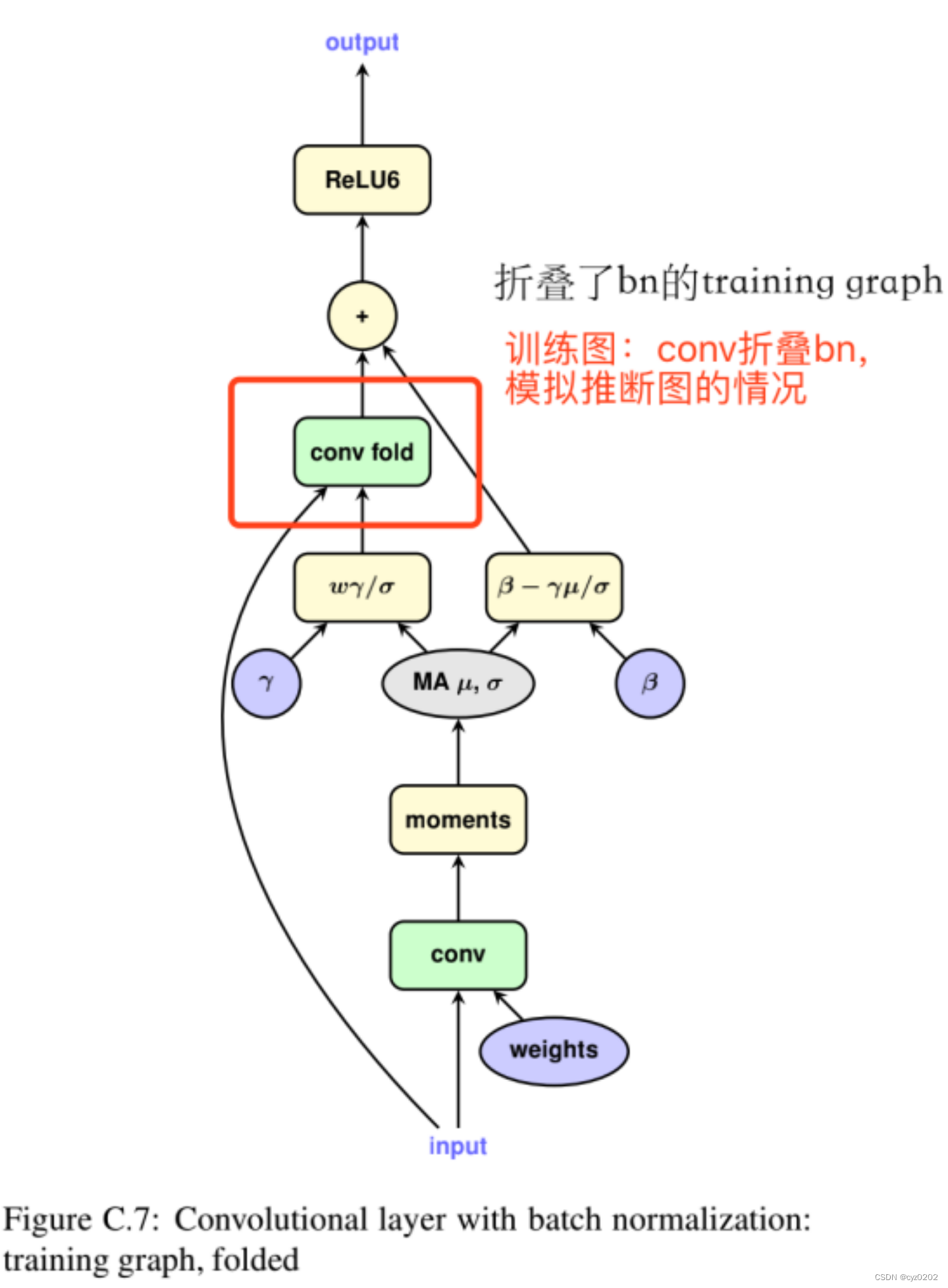
chart 3 Our design idea comes from figure 2, The basic idea is to seek Of EMA( In the picture moment And the steps before and after ) After taking it off, you should match the picture 2 Agreement ;
According to the figure 3 Shown BN Fold , You can do post training quantification or pseudo quantification of the folding in the training stage , Here's the picture 4
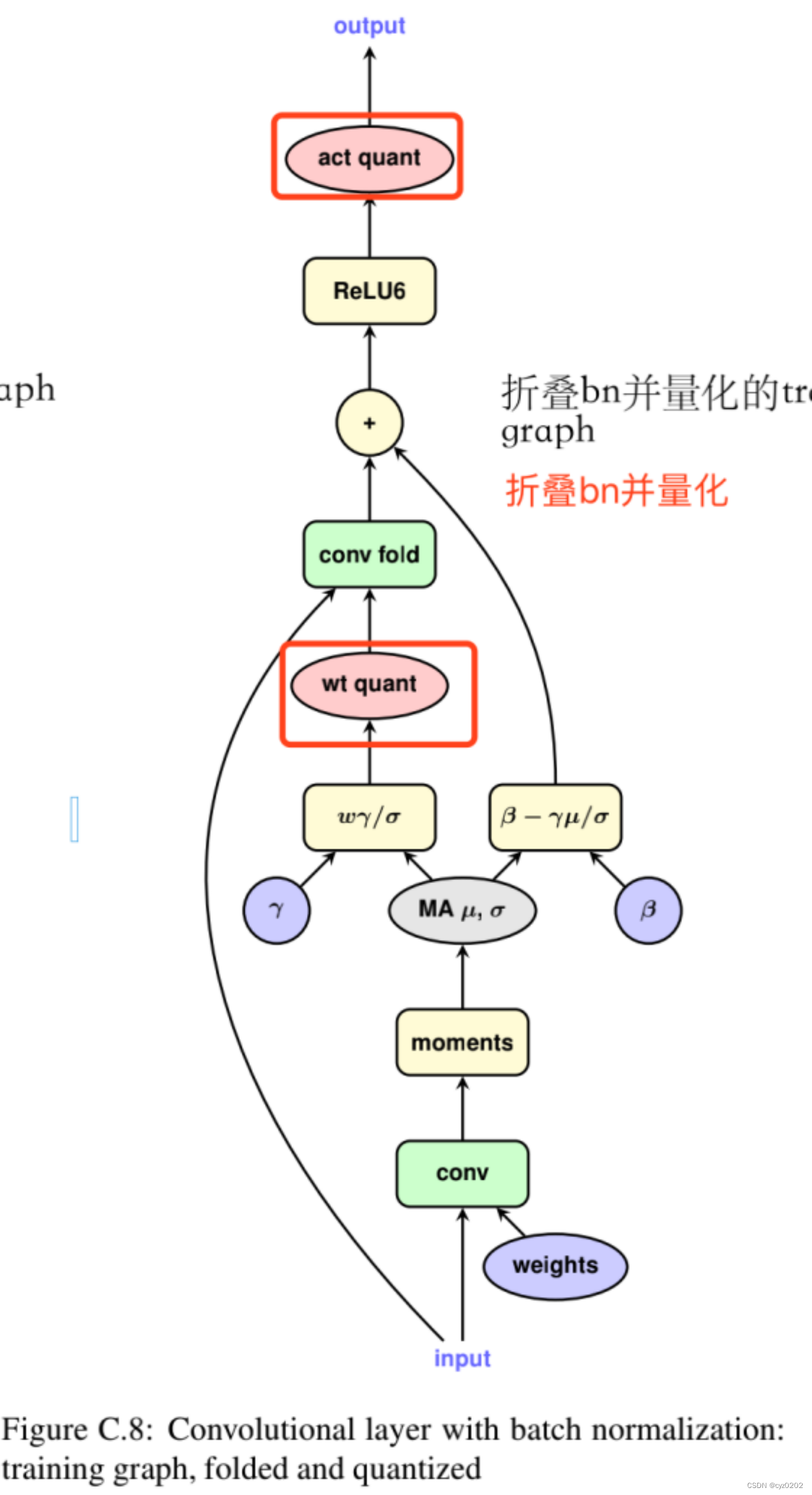
Code implementation
The following code block is from github distiller, There is no complete context implementation , Interested readers can read step by step
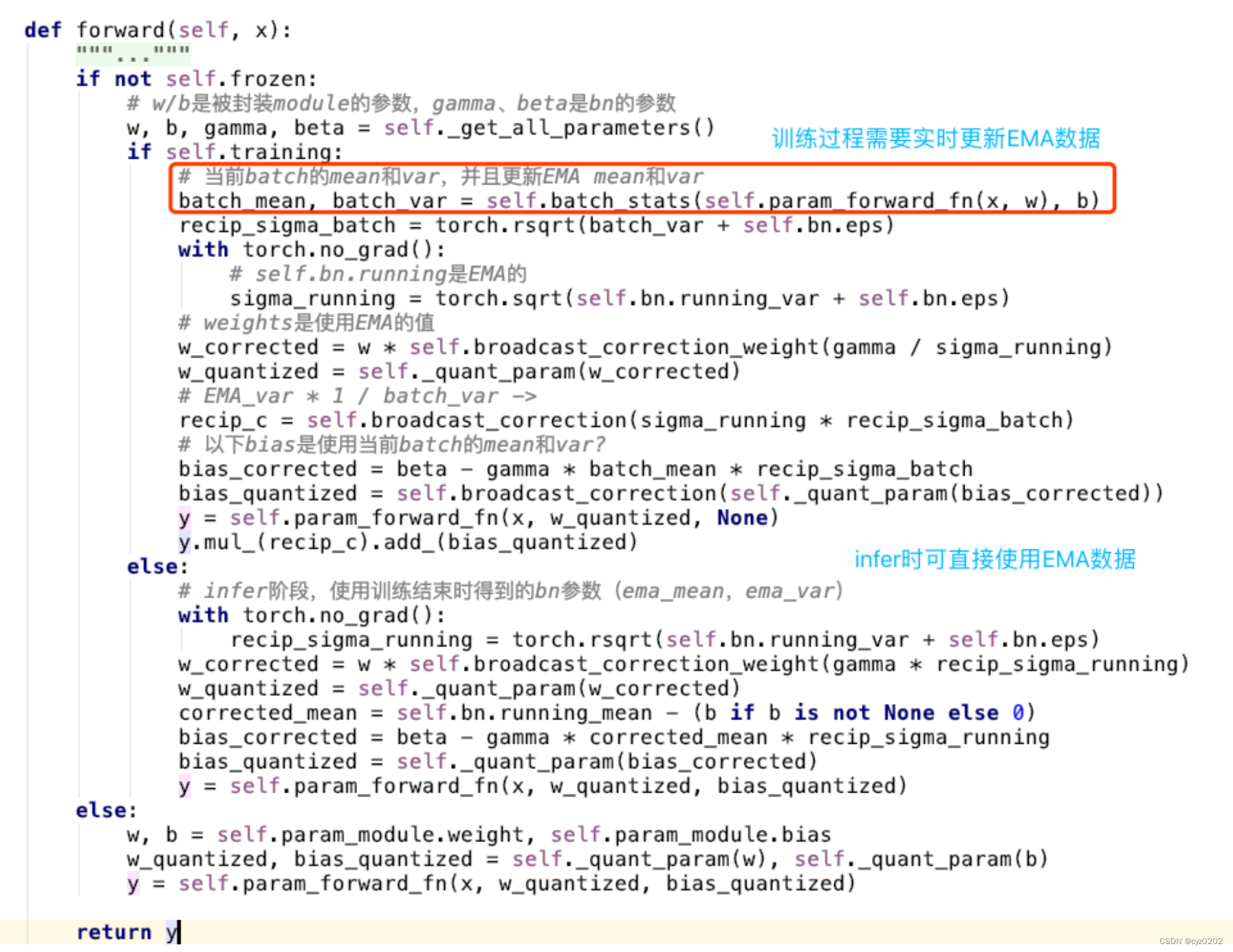
summary
- The above is a brief introduction to BN Folding and its quantification , The thought is quite ingenious , Interested readers can further read in depth according to the literature mentioned in the article , thank you
- Please correct any misunderstandings
边栏推荐
- LeetCode:124. Maximum path sum in binary tree
- Leetcode: Sword finger offer 42 Maximum sum of continuous subarrays
- 超高效!Swagger-Yapi的秘密
- Nacos 的安装与服务的注册
- Current situation and trend of character animation
- LeetCode:236. The nearest common ancestor of binary tree
- [Hacker News Weekly] data visualization artifact; Top 10 Web hacker technologies; Postman supports grpc
- LeetCode:34. Find the first and last positions of elements in a sorted array
- The problem and possible causes of the robot's instantaneous return to the origin of the world coordinate during rviz simulation
- MYSQL卸载方法与安装方法
猜你喜欢
[OC]-<UI入门>--常用控件-UIButton
After PCD is converted to ply, it cannot be opened in meshlab, prompting error details: ignored EOF
UML图记忆技巧
After reading the programmer's story, I can't help covering my chest...
UML diagram memory skills
Indentation of tabs and spaces when writing programs for sublime text
I-BERT
自定义卷积注意力算子的CUDA实现
Deep anatomy of C language -- C language keywords
Improved deep embedded clustering with local structure preservation (Idec)
随机推荐
UML圖記憶技巧
[OC]-<UI入门>--常用控件的学习
Current situation and trend of character animation
UnsupportedOperationException异常
在QWidget上实现窗口阻塞
可变长参数
Problems encountered in connecting the database of the project and their solutions
KDD 2022论文合集(持续更新中)
[OC]-<UI入门>--常用控件-提示对话框 And 等待提示器(圈)
ROS compilation calls the third-party dynamic library (xxx.so)
数学建模2004B题(输电问题)
Super efficient! The secret of swagger Yapi
Mongodb installation and basic operation
Improved deep embedded clustering with local structure preservation (Idec)
Alibaba cloud server mining virus solution (practiced)
自定义卷积注意力算子的CUDA实现
CUDA implementation of self defined convolution attention operator
Philosophical enlightenment from single point to distributed
LeetCode:39. Combined sum
vb. Net changes with the window, scales the size of the control and maintains its relative position